Independence test for the components of a random vector is a classical problem. When the variances of the components are unknown, various statistics constructed from the sample correlation matrices are often used. In the literature, the limiting distributions of these statistics have been well-studied in both low and high dimensional cases. In this talk, we will discuss a rather general extension of this independence test problem, in high dimensional case. We consider the independence test for k subvectors of a random vector with dimension p, where the dimension of the subvector pi’s can vary from 1 to order p. When the population covariance matrices of the subvectors are unknown, we construct a random matrix model called (sample) block correlation matrix, based on n samples. It turns out that the spectral statistics of the block correlation matrix do not depend on the unknown population covariance. Further, under the null hypothesis, the limiting behavior of the spectral statistics can be described with the aid of the free probability theory. When p/n → y ∈ (0,+∞) as n → ∞, we show that the empirical spectral distribution of the block correlation matrix converges to the free Poisson binomial distribution. Specially, with the increase of components (k → ∞), the empirical spectral distribution of the block correlation matrix converges to the free Poisson distribution (Marchenko-Pastur law). We then further derive the Central Limit Theorem (CLT) for the linear spectral statistics of the block correlation matrix. Our results are established under general distribution assumption on the random vector. It turns out that the CLT is universal and does not depend on the 4-th cumulants of the vector components, due to a self-normalizing effect of the correlation type matrices.
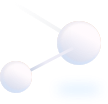
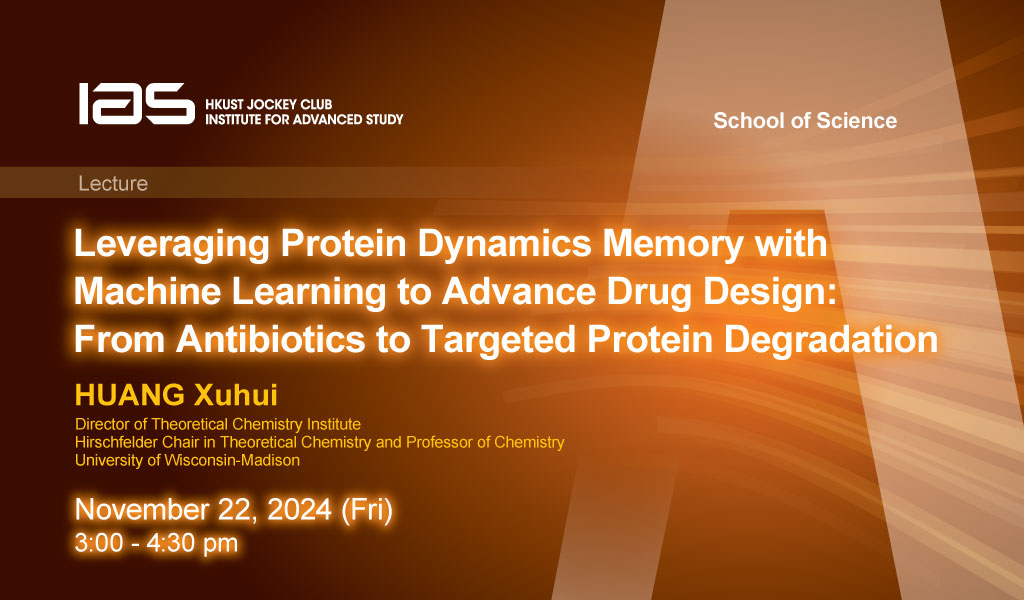
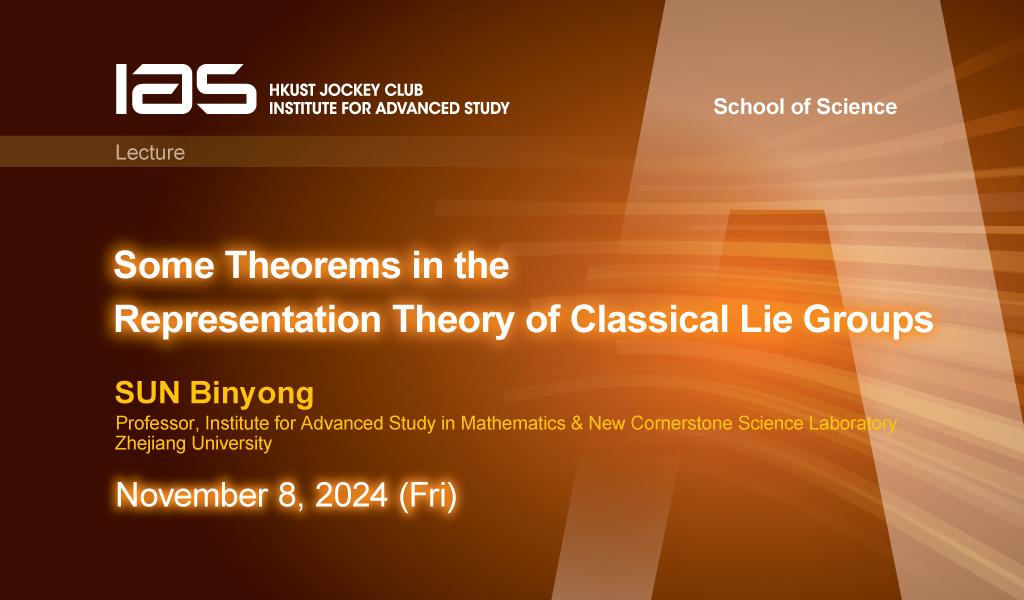