Policy gradient (PG) methods and their variants lie at the heart of modern reinforcement learning. Due to the intrinsic non-concavity of value maximization, however, the theoretical underpinnings of PG-type methods have been limited even until recently. In this talk, we discuss both the ineffectiveness and effectiveness of nonconvex policy optimization. On the one hand, we demonstrate that the popular softmax policy gradient method can take exponential time to converge. On the other hand, we show that employing natural policy gradients and enforcing entropy regularization allows for fast global convergence.
10月17日
11:00am - 12:00pm
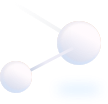
地點
https://hkust.zoom.us/j/94883840530 (Passcode: hkust)
講者/表演者
Prof. Yuting WEI
The Wharton School, University of Pennsyvania
The Wharton School, University of Pennsyvania
主辦單位
Department of Mathematics
聯絡方法
付款詳情
對象
Alumni, Faculty and staff, PG students, UG students
語言
英語
其他活動
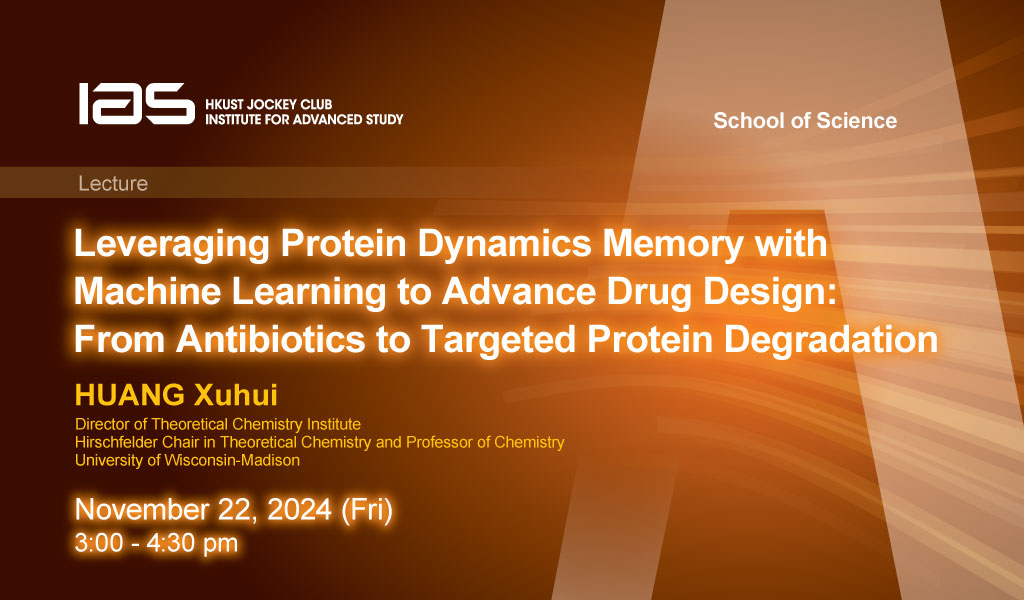
11月22日
研討會, 演講, 講座
IAS / School of Science Joint Lecture - Leveraging Protein Dynamics Memory with Machine Learning to Advance Drug Design: From Antibiotics to Targeted Protein Degradation
Abstract
Protein dynamics are fundamental to protein function and encode complex biomolecular mechanisms. Although Markov state models have made it possible to capture long-timescale protein co...
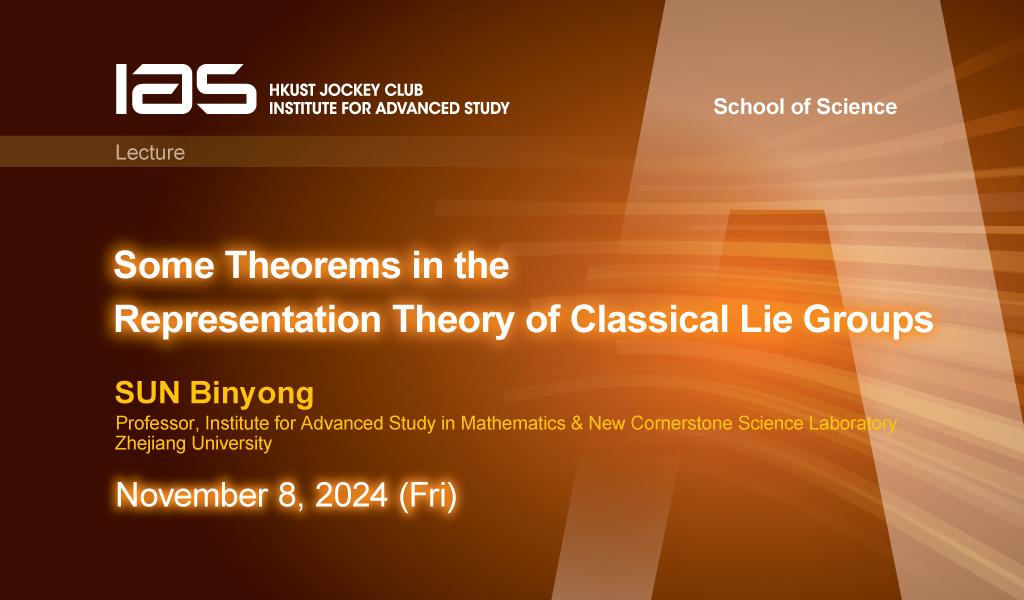
11月8日
研討會, 演講, 講座
IAS / School of Science Joint Lecture - Some Theorems in the Representation Theory of Classical Lie Groups
Abstract
After introducing some basic notions in the representation theory of classical Lie groups, the speaker will explain three results in this theory: the multiplicity one theorem for classical...