A recent breakthrough in deep learning theory shows that the training of over-parameterized deep neural networks (DNNs) can be characterized by the neural tangent kernel (NTK). However, existing optimization and generalization guarantees for deep neural networks (DNNs) typically require a network width larger than a high degree polynomial of the training sample size $n$ and the inverse of the target accuracy $epsilon^{-1}$. In this talk, I will discuss how this over-parameterization condition can be improved to more practical settings. Specifically, I will first explain why over-parameterized DNNs can be optimized to zero training error in the NTK regime, and then show what kind of functions can be learnt by DNNs with small test errors. Under standard NTK-type assumptions, these optimization and generalization guarantees hold with network width polylogarithmic in $n$ and $epsilon^{-1}$.
4月30日
3:00pm - 4:00pm
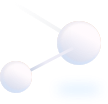
地点
https://hkust.zoom.com.cn/j/5616960008
讲者/表演者
Dr. Yuan CAO
University of California at Los Angeles
University of California at Los Angeles
主办单位
Department of Mathematics
联系方法
mathseminar@ust.hk
付款详情
对象
Alumni, Faculty and Staff, PG Students, UG Students
语言
英语
其他活动
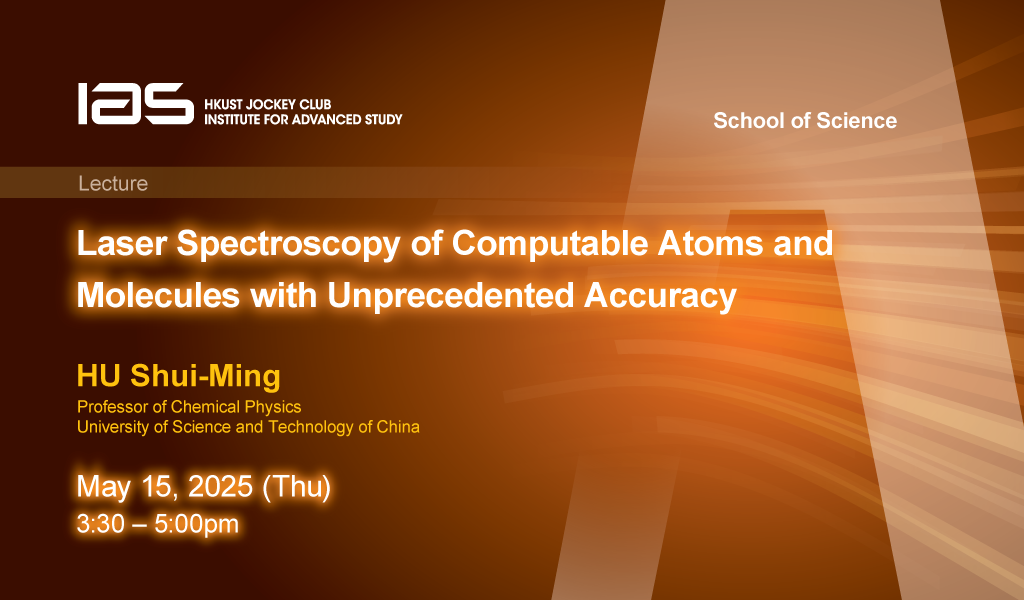
5月15日
研讨会, 演讲, 讲座
IAS / School of Science Joint Lecture - Laser Spectroscopy of Computable Atoms and Molecules with Unprecedented Accuracy
Abstract
Precision spectroscopy of the hydrogen atom, a fundamental two-body system, has been instrumental in shaping quantum mechanics. Today, advances in theory and experiment allow us to ext...
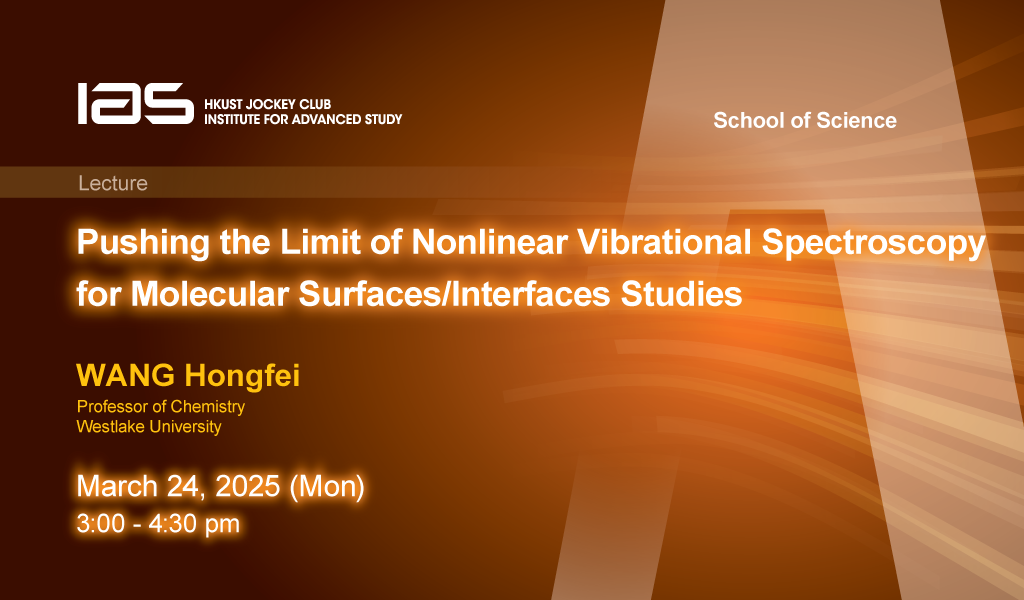
3月24日
研讨会, 演讲, 讲座
IAS / School of Science Joint Lecture - Pushing the Limit of Nonlinear Vibrational Spectroscopy for Molecular Surfaces/Interfaces Studies
Abstract
Surfaces and interfaces are ubiquitous in Nature. Sum-frequency generation vibrational spectroscopy (SFG-VS) is a powerful surface/interface selective and sub-monolayer sensitive spect...