Deep generative models are a category of machine learning models that utilizes deep neural networks to model data distributions and generate new samples. In this seminar, we first introduce our proposed framework to learn a generative model via Schrödinger Bridge, as a stochastic differential equation (SDE)-based generative model. The generative learning task can be formulated as interpolating between a reference distribution and a target distribution based on the Kullback-Leibler divergence, which can be characterized via an SDE on [0, 1] with a time-varying drift term. However, although SDE-based generative models have achieved state-of-the-art performance, they have a less efficient sampling procedure compared with other models such as generative adversarial networks. In the next part, we will discuss feasible ways to solve this problem.
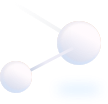
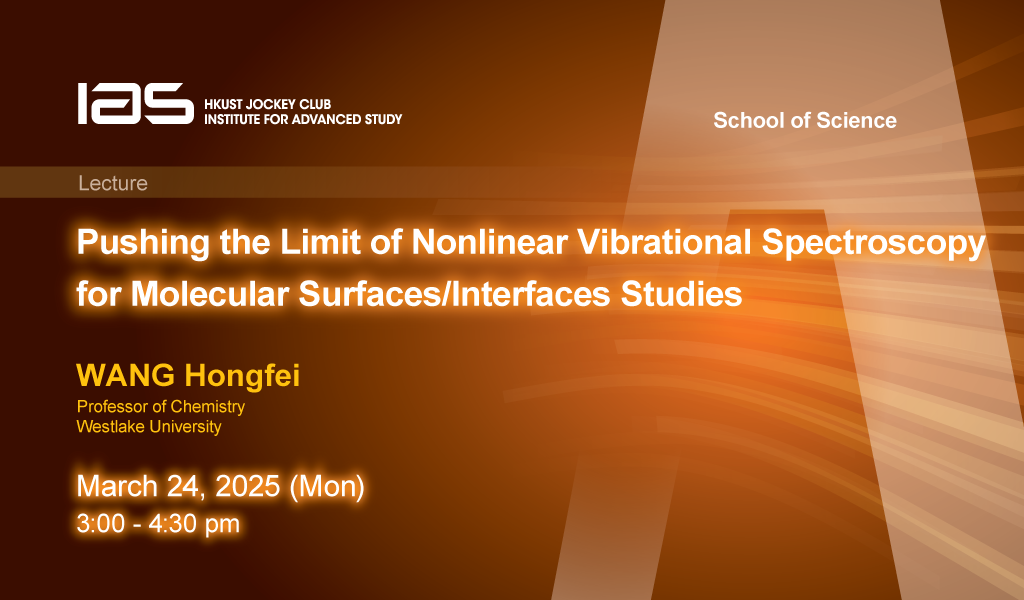
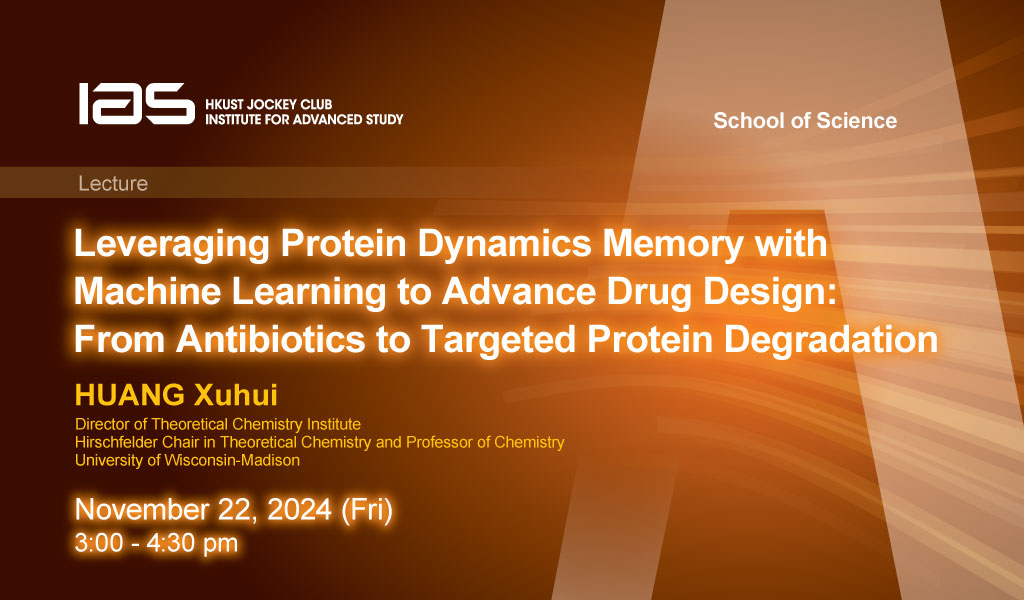