The projection pursuit regression (PPR) has played an important role in the development of statistics and machine learning. However, when compared to other established methods like random forests (RF) and support vector machines (SVM), PPR has yet to showcase a similar level of accuracy as a statistical learning technique. In this paper, we revisit the estimation of PPR and propose an optimal greedy algorithm and an ensemble approach via "feature bagging", hereafter referred to as ePPR, aiming to improve the efficacy. Compared to RF, ePPR has two main advantages. Firstly, its theoretical consistency can be proved for more general regression functions as long as they are L2 integrable, and higher consistency rates can be achieved. Secondly, ePPR does not split the samples, and thus each term of PPR is estimated using the whole data, making the minimization more efficient and guaranteeing the smoothness of the estimator. Extensive comparisons based on real data sets show that ePPR is more efficient in regression and classification than RF and other competitors. The efficacy of ePPR, as a variant of Artificial Neural Networks (ANN), demonstrates that with suitable statistical tuning, ANN can equal or even exceed RF in dealing with small to medium-sized datasets. This finding challenges the widespread belief that ANN ’ s superiority over RF is limited to processing big data.
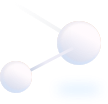
Department of Statistics and Applied Probability, National University of Singapore
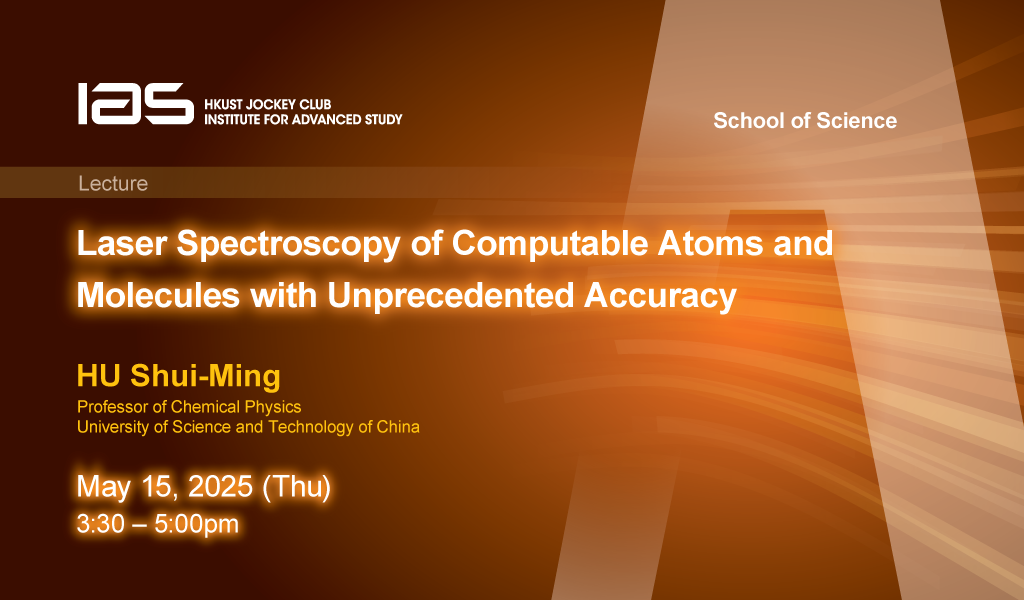