In this paper, we propose a robust policy evaluation algorithm in reinforcement learning, to feature outlier contamination and heavy-tailed reward distributions. We further develop a fully-online method to conduct statistical inference for the modeling parameters. Our method converges faster to the minimum asymptotic variance than the classical temporal difference (TD) learning and avoids the selection of the step sizes. Numerical experiments are provided on the effectiveness of the proposed algorithm in real-world reinforcement learning experiments, which highlight the efficiency and robustness of our approach when compared to the existing online bootstrap method. This work is joint with Jiyuan Tu (SUFE), Xi Chen (NYU), and Weidong Liu (SJTU).
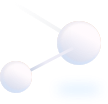
Purdue University
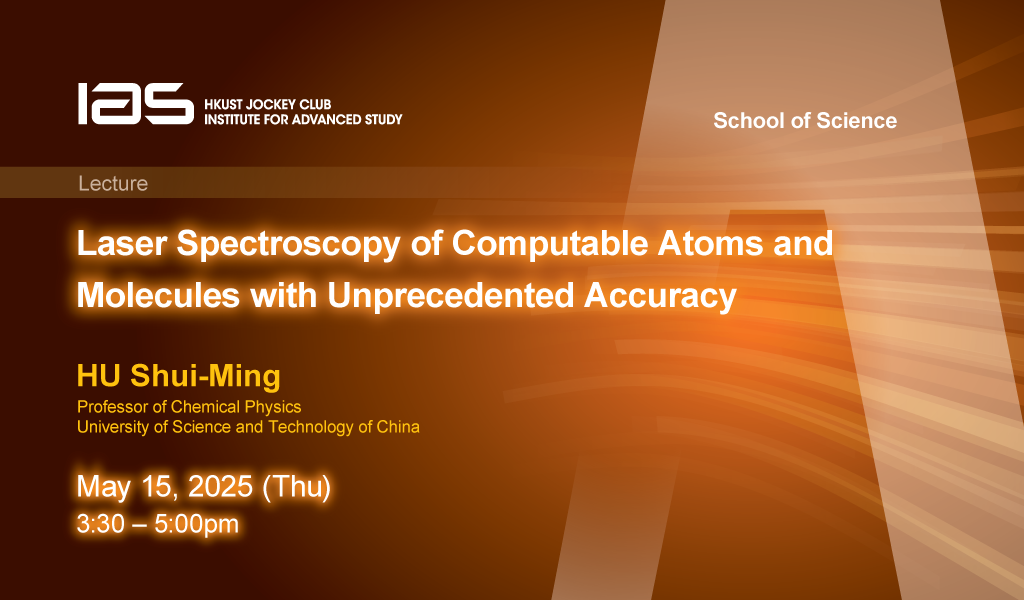
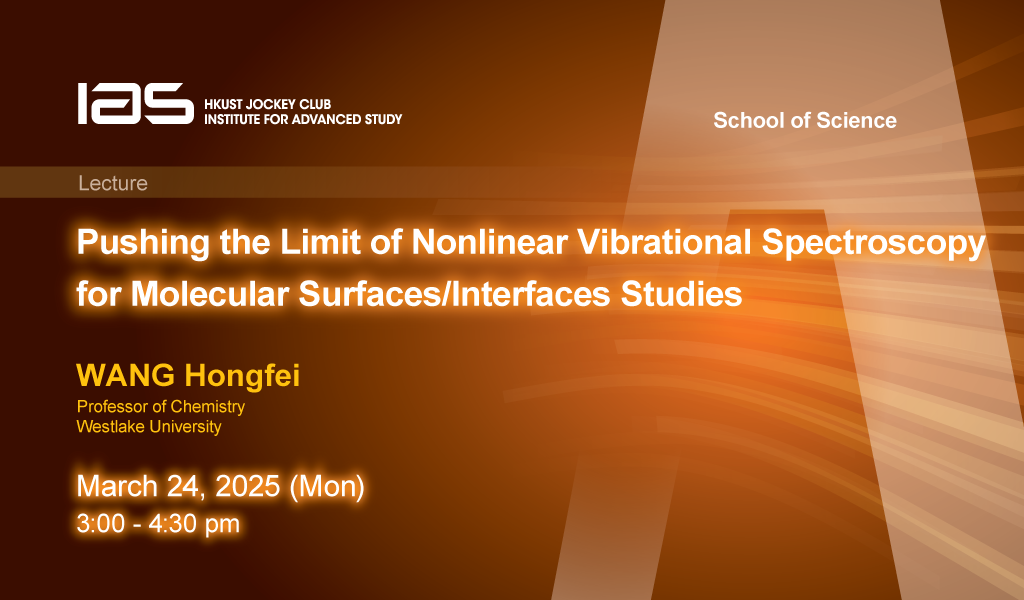