With the development of financial markets, more and more complicated and advanced derivatives are designed to fit the market’s interests. There exotics products are often linked with multi-assets and under untraditional risk, e.g. correlation, credit-default-risk, etc. Also, as hedging instruments for exotic products can also be illiquid/exotic, hedging can be significant and has a great contribution to final PnL.
If we take all above into consideration, traditional approach of derivative pricing might fail as a lot of assumptions will not hold. At the same time, as we know the analytical solutions of derivative pricing under risk-neutral measure is an optimal solution of B/S PDE, which can also be solved numerically, this gives us the idea to utilize neural networks to conduct optimization to solve these kind of problems.
In this presentation, we will show that we can solve different types of derivative pricing based on back-propagation, starting from vanilla European style options. Also, we can see that a lot of important features of B/S pricing also hold by using NN pricing engine (e.g. vol smile, put-call parity, etc.) by introducing different objective function. In the end, we will talk about the potential of implementing more advanced NN structure to more complicated payoffs that are actually being traded in the market.
If we take all above into consideration, traditional approach of derivative pricing might fail as a lot of assumptions will not hold. At the same time, as we know the analytical solutions of derivative pricing under risk-neutral measure is an optimal solution of B/S PDE, which can also be solved numerically, this gives us the idea to utilize neural networks to conduct optimization to solve these kind of problems.
In this presentation, we will show that we can solve different types of derivative pricing based on back-propagation, starting from vanilla European style options. Also, we can see that a lot of important features of B/S pricing also hold by using NN pricing engine (e.g. vol smile, put-call parity, etc.) by introducing different objective function. In the end, we will talk about the potential of implementing more advanced NN structure to more complicated payoffs that are actually being traded in the market.
9月10日
6:00pm - 7:00pm
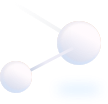
地點
Room 4472, Academic Building (near Lifts 25-26)
講者/表演者
Mr. Hongyu MAO
HKUST
HKUST
主辦單位
Department of Mathematics
聯絡方法
mathseminar@ust.hk
付款詳情
對象
Alumni, Faculty and Staff, PG Students, UG Students
語言
英語
其他活動
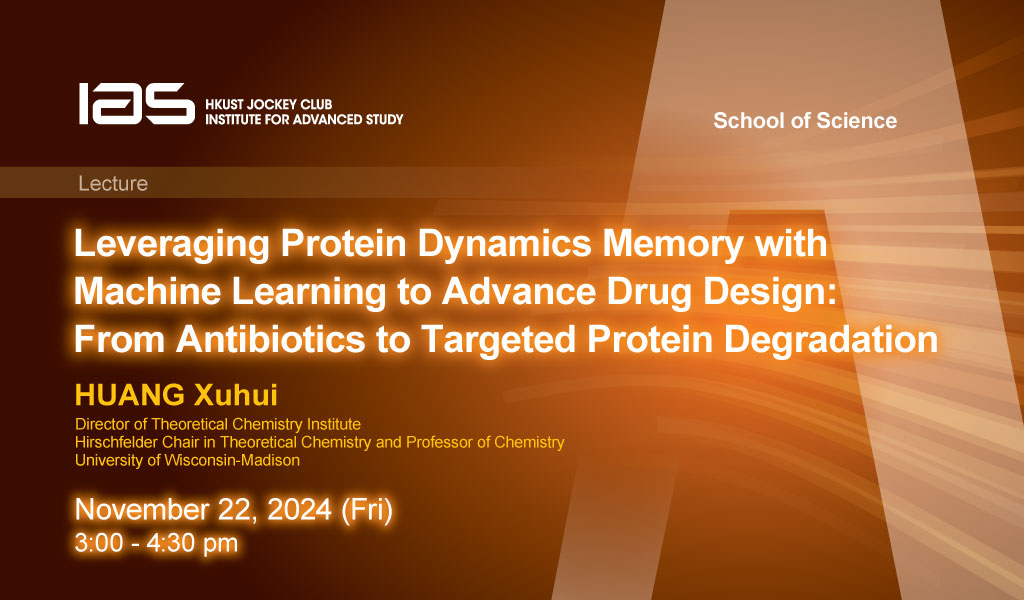
11月22日
研討會, 演講, 講座
IAS / School of Science Joint Lecture - Leveraging Protein Dynamics Memory with Machine Learning to Advance Drug Design: From Antibiotics to Targeted Protein Degradation
Abstract
Protein dynamics are fundamental to protein function and encode complex biomolecular mechanisms. Although Markov state models have made it possible to capture long-timescale protein co...
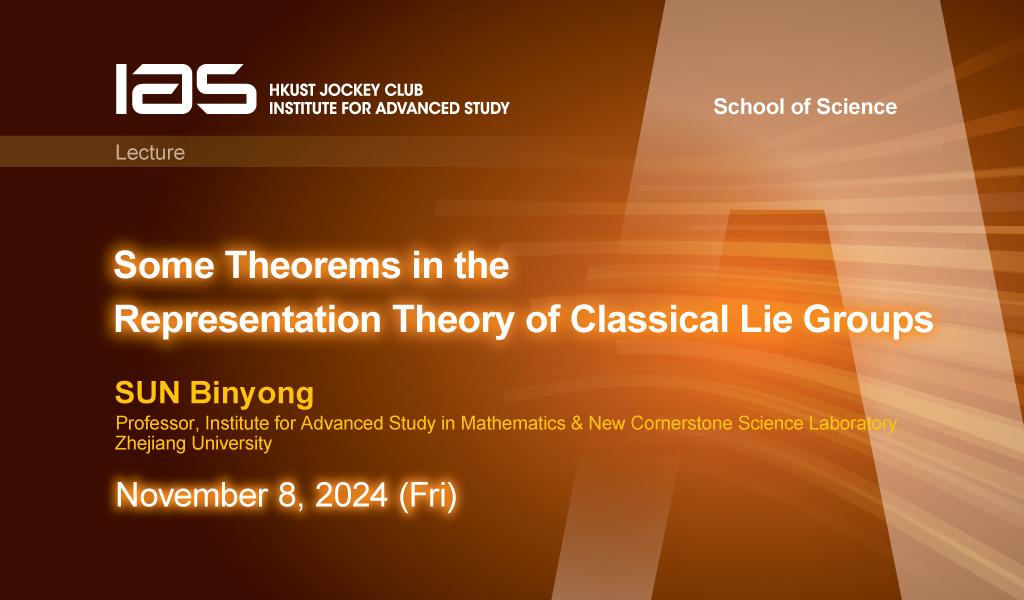
11月8日
研討會, 演講, 講座
IAS / School of Science Joint Lecture - Some Theorems in the Representation Theory of Classical Lie Groups
Abstract
After introducing some basic notions in the representation theory of classical Lie groups, the speaker will explain three results in this theory: the multiplicity one theorem for classical...