In this talk, we first consider a canonical clustering problem where one receives unlabeled samples drawn from a balanced mixture of two elliptical distributions and aims for a classifier to estimate the labels. Many popular methods including PCA and k-means require individual components of the mixture to be somewhat spherical, and perform poorly when they are stretched. To overcome this issue, we propose a non-convex program seeking for an affine transform to turn the data into a one-dimensional point cloud concentrating around -1 and 1, after which clustering becomes easy. Our theoretical contributions are two-fold: (1) we show that the non-convex loss function exhibits desirable landscape properties as long as the sample size exceeds some constant multiple of the dimension, and (2) we leverage this to prove that an efficient first-order algorithm achieves near-optimal statistical precision even without good initialization. We also propose a general methodology for multi-class clustering tasks with flexible choices of feature transforms and loss objectives.
5月8日
9:30am - 11:00am
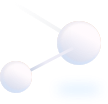
地點
https://hkust.zoom.us/j/5616960008
講者/表演者
Dr. Kaizheng WANG
Princeton University and Columbia University
Princeton University and Columbia University
主辦單位
Department of Mathematics
聯絡方法
mathseminar@ust.hk
付款詳情
對象
Alumni, Faculty and Staff, PG Students, UG Students
語言
英語
其他活動
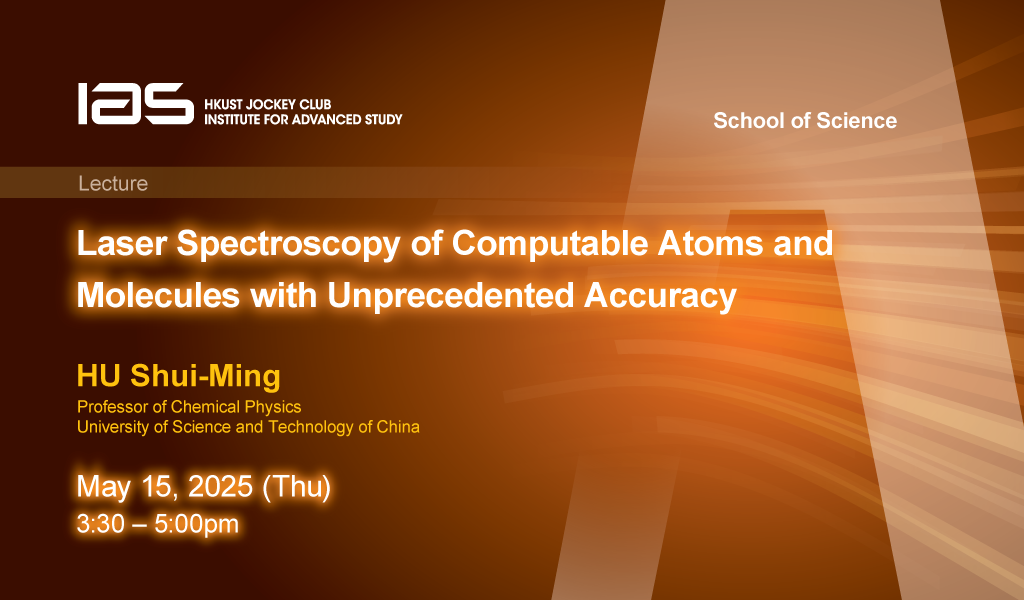
5月15日
研討會, 演講, 講座
IAS / School of Science Joint Lecture - Laser Spectroscopy of Computable Atoms and Molecules with Unprecedented Accuracy
Abstract
Precision spectroscopy of the hydrogen atom, a fundamental two-body system, has been instrumental in shaping quantum mechanics. Today, advances in theory and experiment allow us to ext...
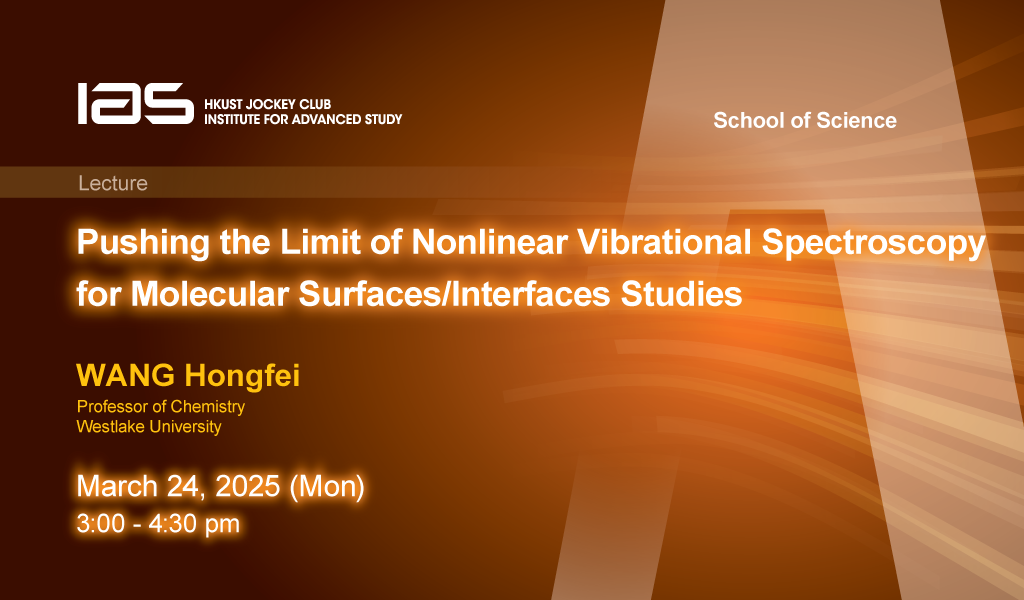
3月24日
研討會, 演講, 講座
IAS / School of Science Joint Lecture - Pushing the Limit of Nonlinear Vibrational Spectroscopy for Molecular Surfaces/Interfaces Studies
Abstract
Surfaces and interfaces are ubiquitous in Nature. Sum-frequency generation vibrational spectroscopy (SFG-VS) is a powerful surface/interface selective and sub-monolayer sensitive spect...