We consider the problem of assessing the importance of multiple variables or factors from a dataset when side information is available. In principle, using side information can allow the statistician to pay attention to variables with a greater potential, which in turn, may lead to more discoveries. We introduce an adaptive knockoff filter, which generalizes the knockoff procedure (Barber and Candès, 2015; Candès et al., 2018) in that it uses both the data at hand and side information to adaptively order the variables under study and focus on those that are most promising. Adaptive knockoffs controls the finite-sample false discovery rate (FDR) and we demonstrate its power by comparing it with other structured multiple testing methods. We also apply our methodology to real genetic data in order to find associations between genetic variants and various phenotypes such as Crohn’s disease and lipid levels. Here, adaptive knockoffs makes more discoveries than reported in previous studies on the same datasets.
3月26日
10:30am - 11:30am
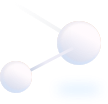
地點
https://hkust.zoom.com.cn/j/5616960008
講者/表演者
Ms. Zhimei REN
Stanford University
Stanford University
主辦單位
Department of Mathematics
聯絡方法
mathseminar@ust.hk
付款詳情
對象
Alumni, Faculty and Staff, PG Students, UG Students
語言
英語
其他活動
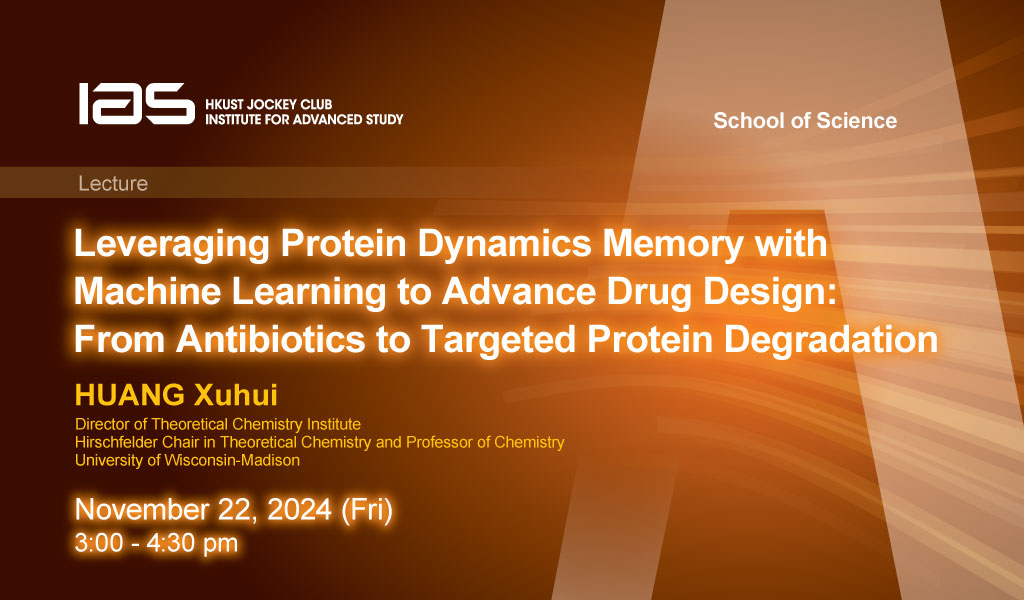
11月22日
研討會, 演講, 講座
IAS / School of Science Joint Lecture - Leveraging Protein Dynamics Memory with Machine Learning to Advance Drug Design: From Antibiotics to Targeted Protein Degradation
Abstract
Protein dynamics are fundamental to protein function and encode complex biomolecular mechanisms. Although Markov state models have made it possible to capture long-timescale protein co...
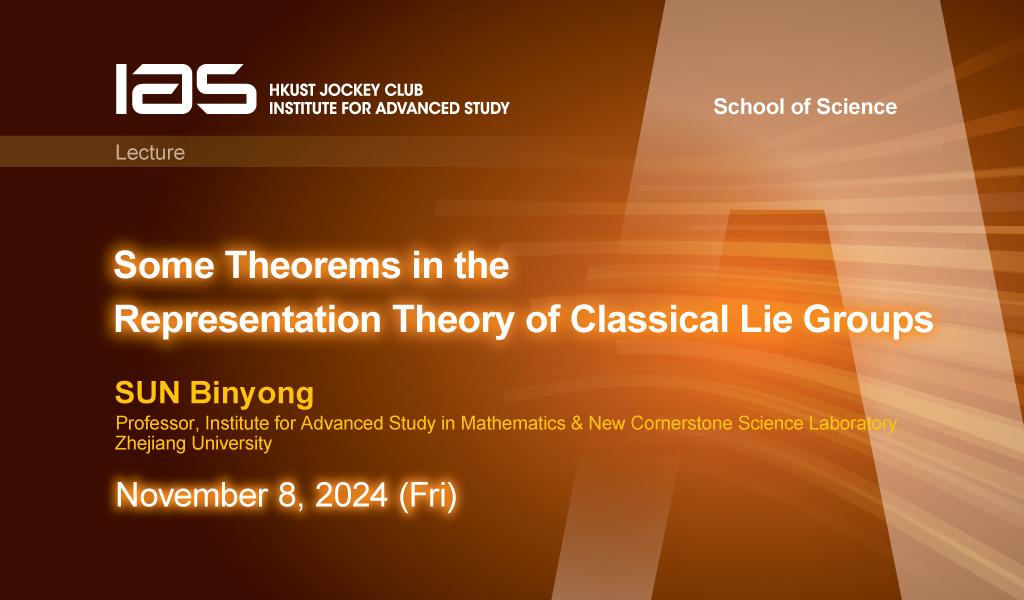
11月8日
研討會, 演講, 講座
IAS / School of Science Joint Lecture - Some Theorems in the Representation Theory of Classical Lie Groups
Abstract
After introducing some basic notions in the representation theory of classical Lie groups, the speaker will explain three results in this theory: the multiplicity one theorem for classical...