Non-convex optimization is widely involved in many machine learning problems, and it usually implicates a two-stage algorithm: a refined initialization followed by a local gradient search. Even though recent studies in global geometric analysis have revealed that empirical loss function of many low-rank related problems have favourable landscape in parameterized Euclidean space, it is generally difficult to analyze. In this talk, I will discuss a new unified framework for the analysis of low-rank matrix recovery problems. Instead of classical parameterization in Euclidean space, we considers emperical least square loss function on the manifold of low-rank matrices directly. We show that (1) if the measurement operator satisfies RIP condition with constantly small enough, there would be no spurious critical points, and manifold gradient descent would generate linear convergent sequence to global minimum (e.g. matrix sensing); (2) under weaker assumptions, but with RIP-like distance-preserving condition, global linear convergence rate to local minimum is still guaranteed (e.g. phase retrieval).
7月12日
10:00am - 11:00am
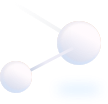
地點
Room 5506, Academic Building, (near Lifts 25-26)
講者/表演者
Ms. Zhenzhen LI
HKUST
HKUST
主辦單位
Department of Mathematics
聯絡方法
mathseminar@ust.hk
付款詳情
對象
Alumni, Faculty and Staff, PG Students, UG Students
語言
英語
其他活動
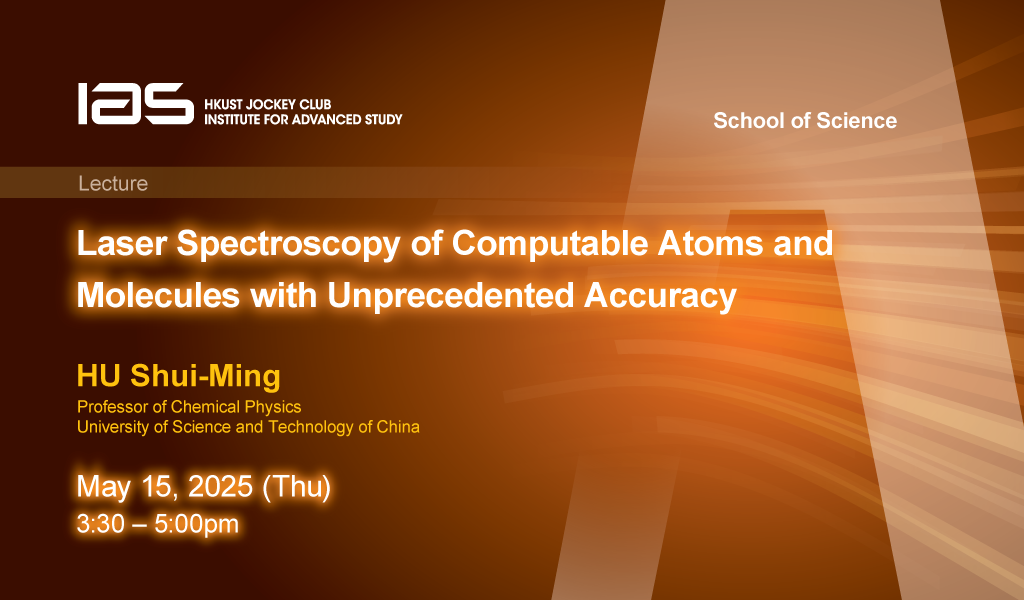
5月15日
研討會, 演講, 講座
IAS / School of Science Joint Lecture - Laser Spectroscopy of Computable Atoms and Molecules with Unprecedented Accuracy
Abstract
Precision spectroscopy of the hydrogen atom, a fundamental two-body system, has been instrumental in shaping quantum mechanics. Today, advances in theory and experiment allow us to ext...