Pruning is a model compression method that removes redundant parameters and accelerates the inference speed of deep neural networks while maintaining accuracy. Most available pruning methods impose various conditions on parameters or features directly. In this talk, we introduce a simple and effective regularization strategy to improve the structured sparsity and structured pruning in DNNs from a new perspective of evolution of features. In particular, we consider the trajectories connecting features of adjacent hidden layers, namely feature flow. We propose feature flow regularization (FFR) to penalize the length and the total absolute curvature of the trajectories, which implicitly increases the structured sparsity of the parameters. The principle behind FFR is that short and straight trajectories will lead to an efficient network that avoids redundant parameters. We provide experiment results on CIFAR-10 and ImageNet datasets which show that FFR improves structured sparsity and achieves pruning results comparable to or even better than those state-of-the-art methods.
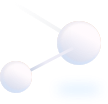
HKUST
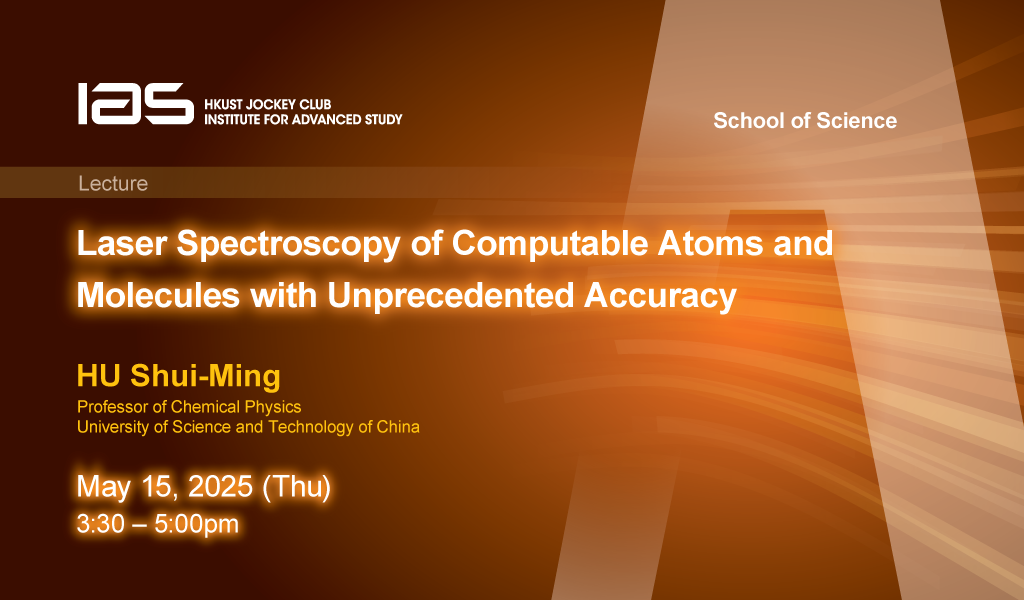