In this talk, we study the multi-task learning problem that aims to simultaneously analyze multiple datasets collected from different sources and learn one model for each of them. We propose a family of adaptive methods that automatically utilize possible similarities among those tasks while carefully handling their differences. We derive optimal statistical guarantees for the methods and prove their robustness against outlier tasks. Numerical experiments on synthetic and real datasets demonstrate the efficacy of our new methods.
4月13日
10:30am - 11:30am
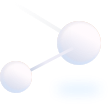
地点
https://hkust.zoom.us/j/5616960008 (Passcode: hkust)
讲者/表演者
Prof. Kaizheng WANG
Columbia University
Columbia University
主办单位
Department of Mathematics
联系方法
付款详情
对象
Alumni, Faculty and staff, PG students, UG students
语言
英语
其他活动
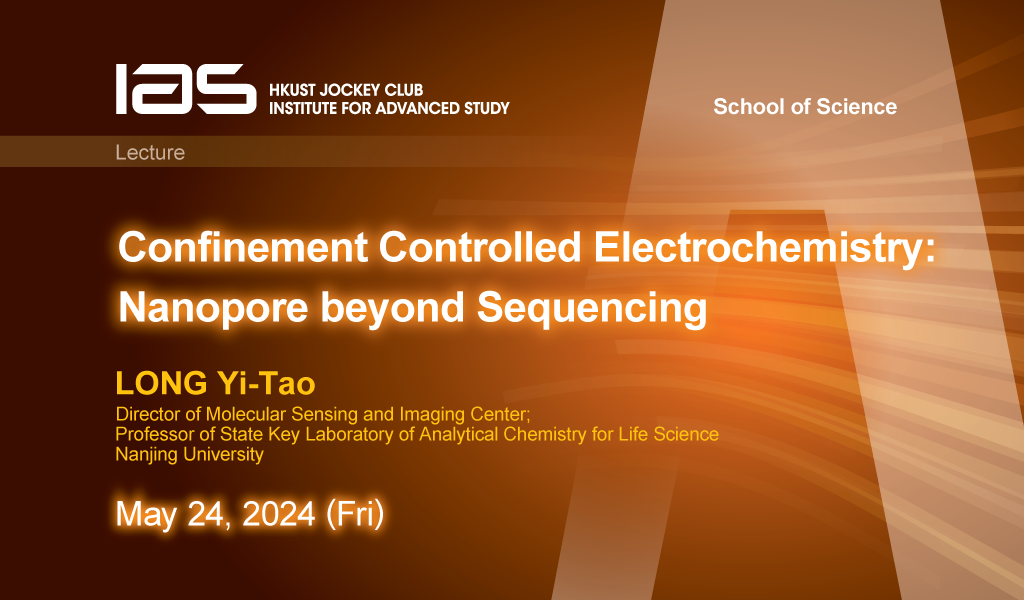
5月24日
研讨会, 演讲, 讲座
IAS / School of Science Joint Lecture - Confinement Controlled Electrochemistry: Nanopore beyond Sequencing
Abstract
Nanopore electrochemistry refers to the promising measurement science based on elaborate pore structures, which offers a well-defined geometric confined space to adopt and characterize sin...
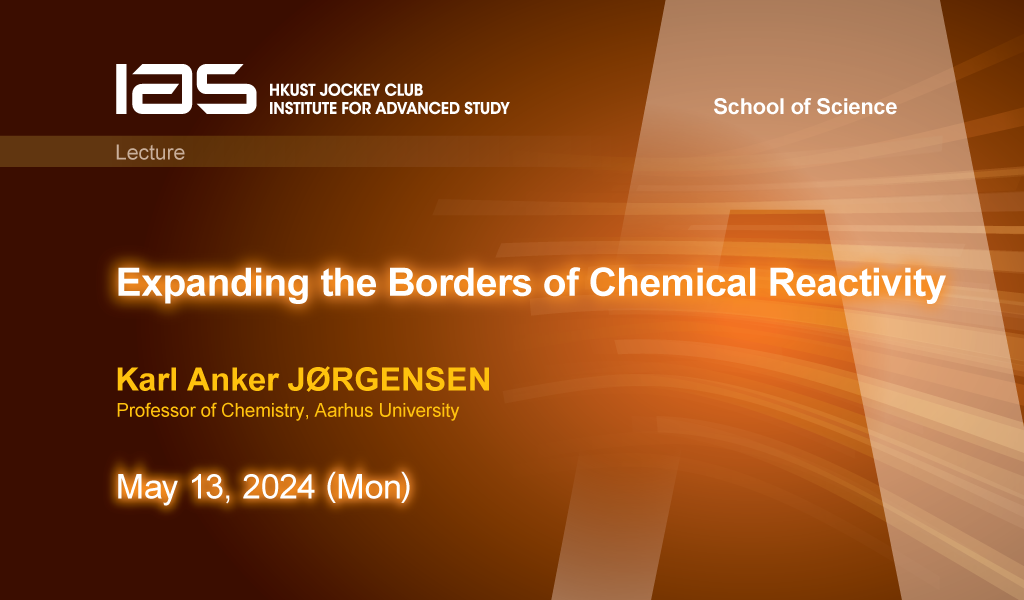
5月13日
研讨会, 演讲, 讲座
IAS / School of Science Joint Lecture – Expanding the Borders of Chemical Reactivity
Abstract
The lecture will demonstrate how it has been possible to expand the borders of cycloadditions beyond the “classical types of cycloadditions” applying organocatalytic activation principles....