Expected Shortfall (ES), also known as superquantile or Conditional Value-at-Risk, has been recognized as an important measure in risk analysis and stochastic optimization. In finance, it refers to the conditional expected return of an asset given that the return is below some quantile of its distribution. In this work, we consider a joint regression framework that simultaneously models the conditional quantile and ES of a response variable given a set of covariates, for which the state-of-the-art approach is based on minimizing a joint loss function that is non-differentiable and non-convex.
Motivated by the idea of using Neyman-orthogonal scores to reduce sensitivity with respect to nuisance parameters, we propose a statistically robust and computationally efficient two-step procedure for fitting joint quantile and ES regression models. With increasing covariate dimensions, we establish explicit non-asymptotic bounds on estimation and Gaussian approximation errors, which lay the foundation for statistical inference. Under high-dimensional sparse models, we consider a lasso-penalized two-step approach and its robust counterpart, and propose debased estimators for inference. We further discuss a more general weighted-average quantile regression framework, including ES regression as a special case. This talk is based on joint works with Xuming He, Kean Ming Tan and Shushu Zhang.
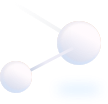
University of California San Diego
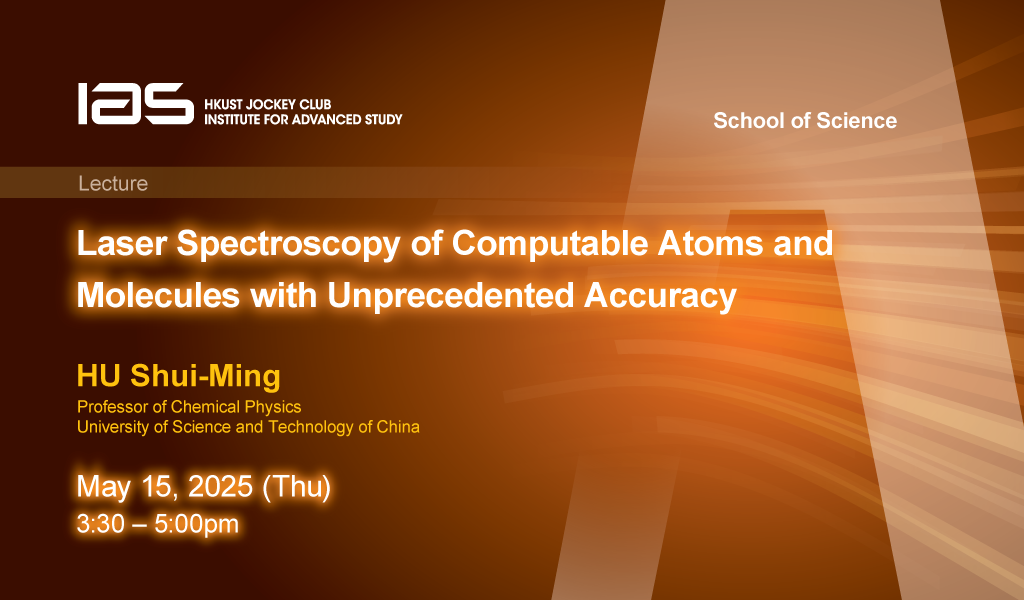