In this seminar, we will discuss private federated learning. We will firstly provide new optimization error bounds for differential private federated learning with Laplacian Smoothing (DP-Fed-LS) and heterogeneous data. The error bounds help us better understand the influence of errors introduced by differential privacy, heterogeneity of data and variance of stochastic gradient descent over the convergence of DP-Fed-LS. For another, we will also explore how to push the limit of private federated learning by improving current gradient attack. Experiment shows that our proposed new attack can recover training data with high quality while the targeted model is untrained and when the batch size is small. Attacks on more realistic settings are to be discussed.
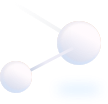
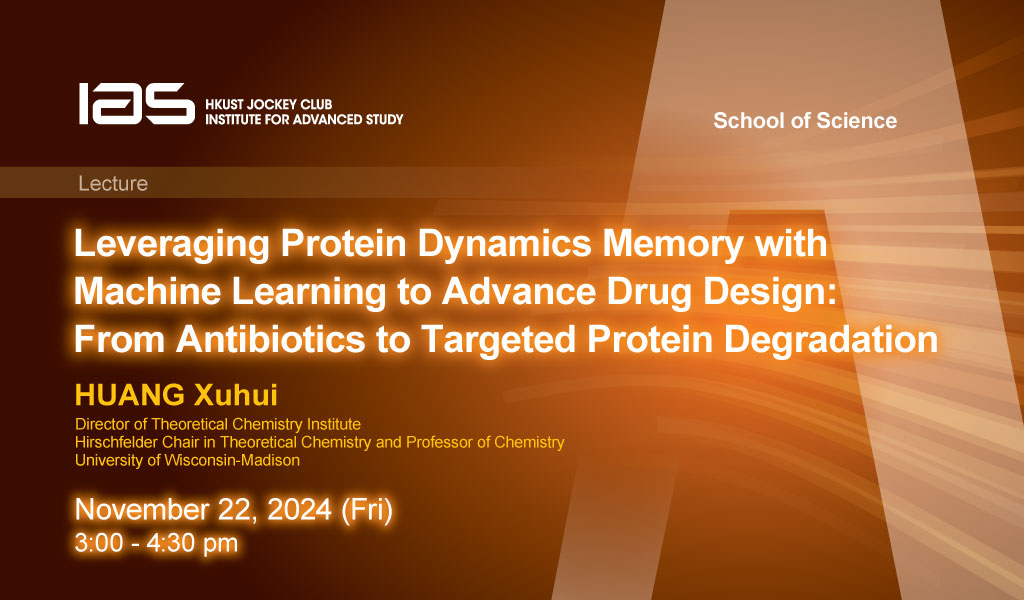
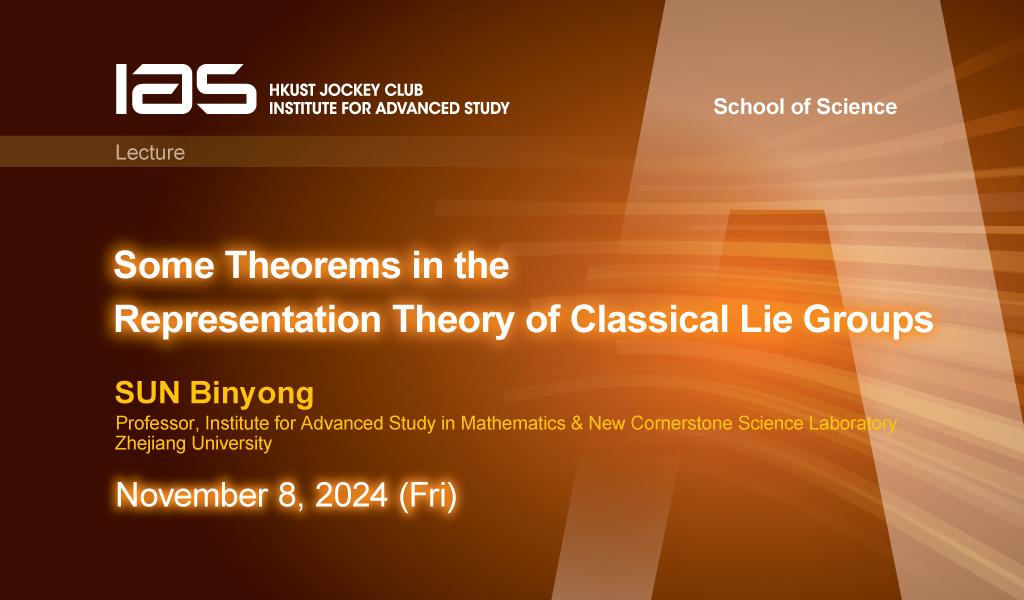