Deep learning is widely used in different areas such as computer vision, natural language processing, and medical data analysis. The success of deep learning relies on large scale dataset which contains data from different sources. However, the massive data collection raises privacy concerns. This seminar introduces a new privacy preserving machine learning (PPML) algorithm called Differential Private Massive Feature Mix-up ( DP-MFM). DP-MFM first utilizes an encoder to map the raw image to a “mixup-robust” feature space, where we can train machine learning algorithms with averaged features and one-hot labels of many samples without severe performance decay comparing with training with data without average. Then a feature dataset is created by averaging hundreds of features and corresponding one-hot labels. Further, massive feature mixup is friendly to differential privacy framework so we can give a differential private guarantee to the feature dataset after injecting gaussian noise. Extensive experiments show its advantage in both utility and privacy comparing with other privacy preserving machine learning algorithms.
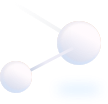
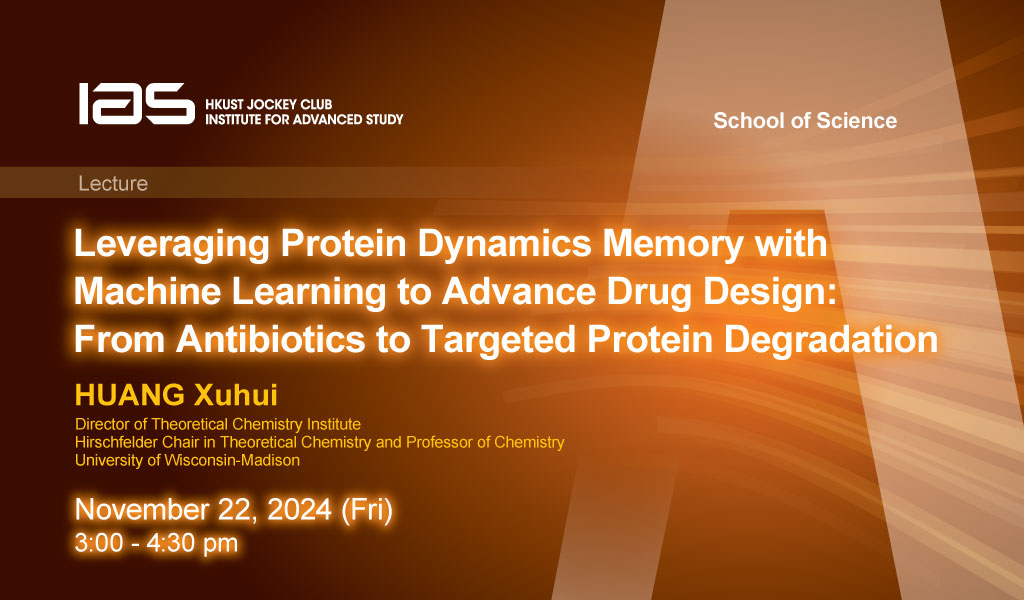
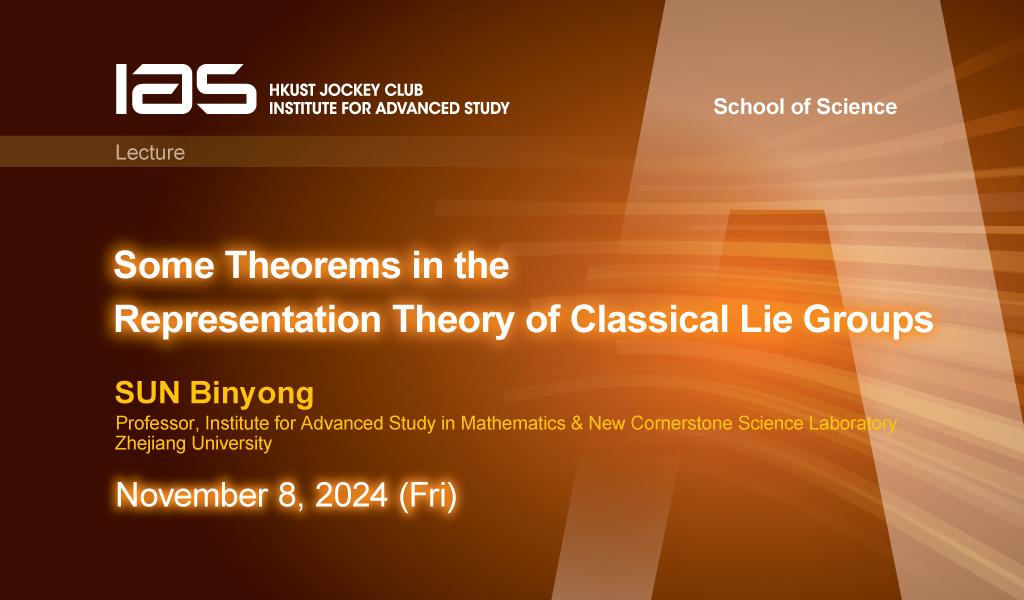