In this seminar I will talk about a provably stable architecture for Neural Ordinary Differential Equations (ODEs) which achieves non-trivial adversarial robustness under white-box adversarial attacks even when the network is trained naturally. For most existing defense methods withstanding strong white-box attacks, to improve robustness of neural networks, they need to be trained adversarially, hence have to strike a trade-off between natural accuracy and adversarial robustness. Inspired by dynamical system theory, we design a stabilized neural ODE network named SONet whose ODE blocks are skew-symmetric and proved to be input-output stable. With natural training, SONet can achieve comparable robustness with the state-of-art adversarial defense methods. In particular, under PGD-20 ($ell_infty=0.031$) attack on CIFAR-10 dataset, our method of natural training achieves 89.36% natural accuracy and 61.62% robust accuracy, while a counterpart architecture of ResNet trained with TRADES achieves natural and robust accuracy 85.28% and 23.06% respectively, in the same setting.
5月14日
4:00pm - 5:00pm
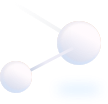
地点
https://hkust.zoom.us/j/98027512081
讲者/表演者
Mr. Yifei HUANG
HKUST
HKUST
主办单位
Department of Mathematics
联系方法
mathseminar@ust.hk
付款详情
对象
Alumni, Faculty and Staff, PG Students, UG Students
语言
英语
其他活动
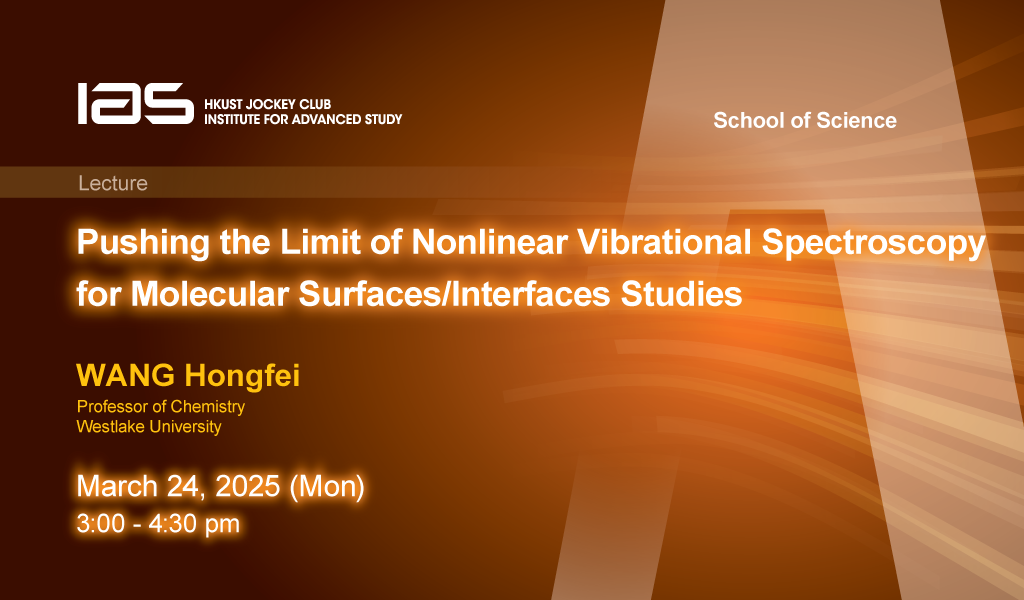
3月24日
研讨会, 演讲, 讲座
IAS / School of Science Joint Lecture - Pushing the Limit of Nonlinear Vibrational Spectroscopy for Molecular Surfaces/Interfaces Studies
Abstract
Surfaces and interfaces are ubiquitous in Nature. Sum-frequency generation vibrational spectroscopy (SFG-VS) is a powerful surface/interface selective and sub-monolayer sensitive spect...
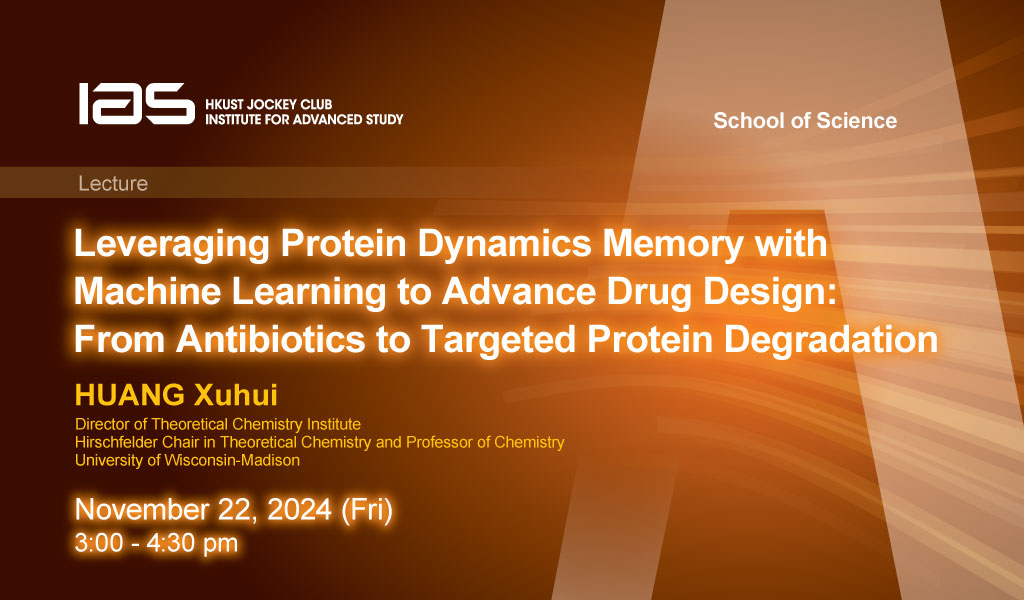
11月22日
研讨会, 演讲, 讲座
IAS / School of Science Joint Lecture - Leveraging Protein Dynamics Memory with Machine Learning to Advance Drug Design: From Antibiotics to Targeted Protein Degradation
Abstract
Protein dynamics are fundamental to protein function and encode complex biomolecular mechanisms. Although Markov state models have made it possible to capture long-timescale protein co...