Recently, [1] showed that several machine learning algorithms, such as Lasso, Support Vector Machines, and regularized logistic regression, and many others can be represented exactly as distributionally robust optimization (DRO) problems. The uncertainty is then defined as a neighborhood centered at the empirical distribution. A key element of the study of uncertainty is the Robust Wasserstein Profile function. In [1], the authors study the asymptotic behavior of the RWP function in the case of L^p costs under the true parameter. We consider costs in more generalized forms, namely Bregman distance or in the more general symmetric format of d(x-y) and analyze the asymptotic behavior of the RWPI function in these cases. For the purpose of statistical applications, we then study the RWP function with plug-in estimators. This is a joint work with Yue Hui, Jose Blanchet and Peter Glynn.
[1] Blanchet, J., Kang, Y., & Murthy, K. Robust Wasserstein Profile Inference and Applications to Machine Learning, arXiv:1610.05627, 2016.
[1] Blanchet, J., Kang, Y., & Murthy, K. Robust Wasserstein Profile Inference and Applications to Machine Learning, arXiv:1610.05627, 2016.
9月18日
3:00pm - 4:30pm
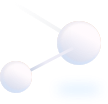
地点
LTJ, Academic Building (near Lift 33), HKUST
讲者/表演者
Dr. Jin XIE
Stanford University
Stanford University
主办单位
Department of Mathematics
联系方法
mathseminar@ust.hk
付款详情
对象
Alumni, Faculty and Staff, PG Students, UG Students
语言
英语
其他活动
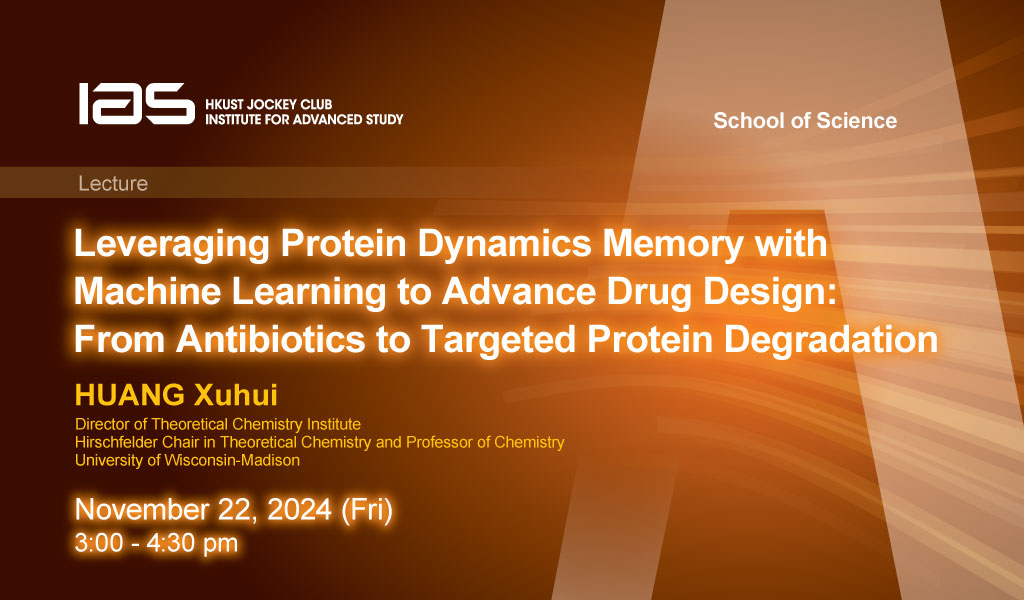
11月22日
研讨会, 演讲, 讲座
IAS / School of Science Joint Lecture - Leveraging Protein Dynamics Memory with Machine Learning to Advance Drug Design: From Antibiotics to Targeted Protein Degradation
Abstract
Protein dynamics are fundamental to protein function and encode complex biomolecular mechanisms. Although Markov state models have made it possible to capture long-timescale protein co...
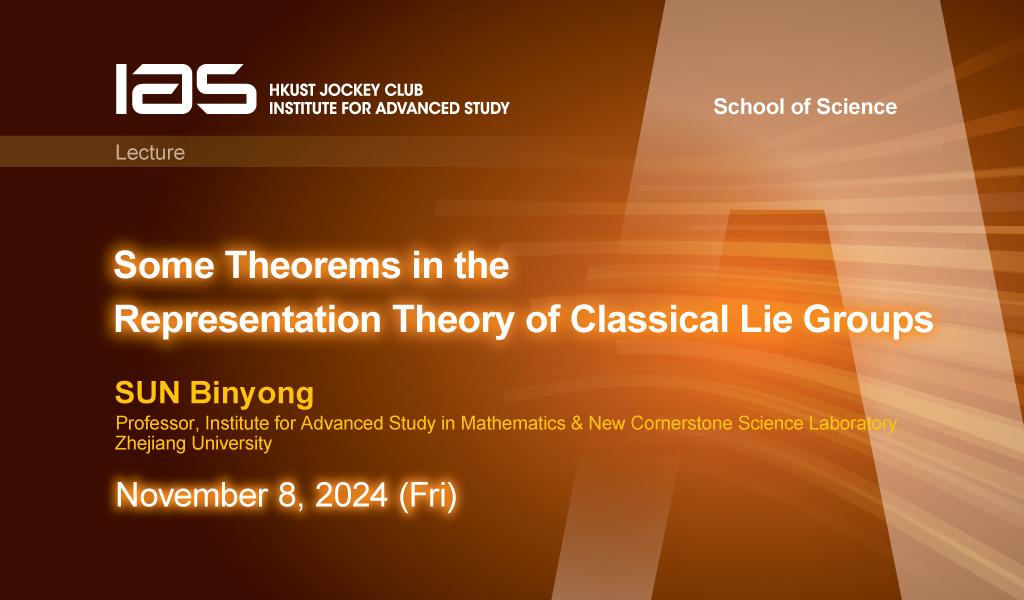
11月8日
研讨会, 演讲, 讲座
IAS / School of Science Joint Lecture - Some Theorems in the Representation Theory of Classical Lie Groups
Abstract
After introducing some basic notions in the representation theory of classical Lie groups, the speaker will explain three results in this theory: the multiplicity one theorem for classical...