In many applications fields such as economics, finance and engineering, matrix or tensor observations are now commonly observed over time. Often the dimensions of these time series data high. Factor models have been used as a dimension reduction tool, resulting in matrix/tensor factor models. Such factor models do not assume any specific dynamic structures of the latent factor process, hence is not generative and cannot be used to make predictions. In this paper, we propose a dynamic matrix/tensor factor model that extends the factor model by modeling the latent low dimensional factor process with an autoregressive structure. A two stage procedure is used for estimation. Theoretical properties and finite sample empirical properties of the estimator are presented, along with application examples.
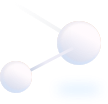
Rutgers University
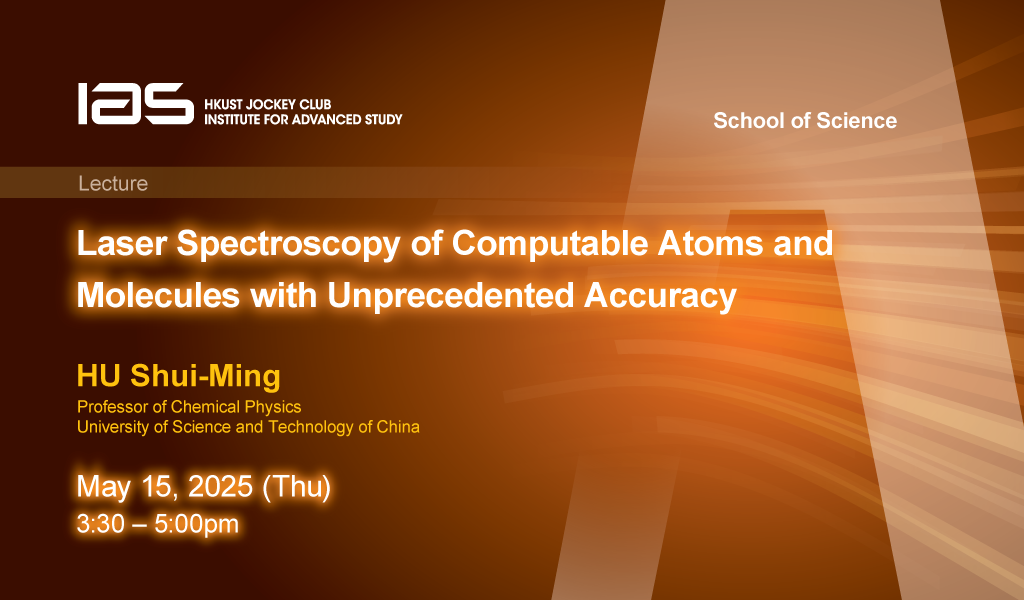
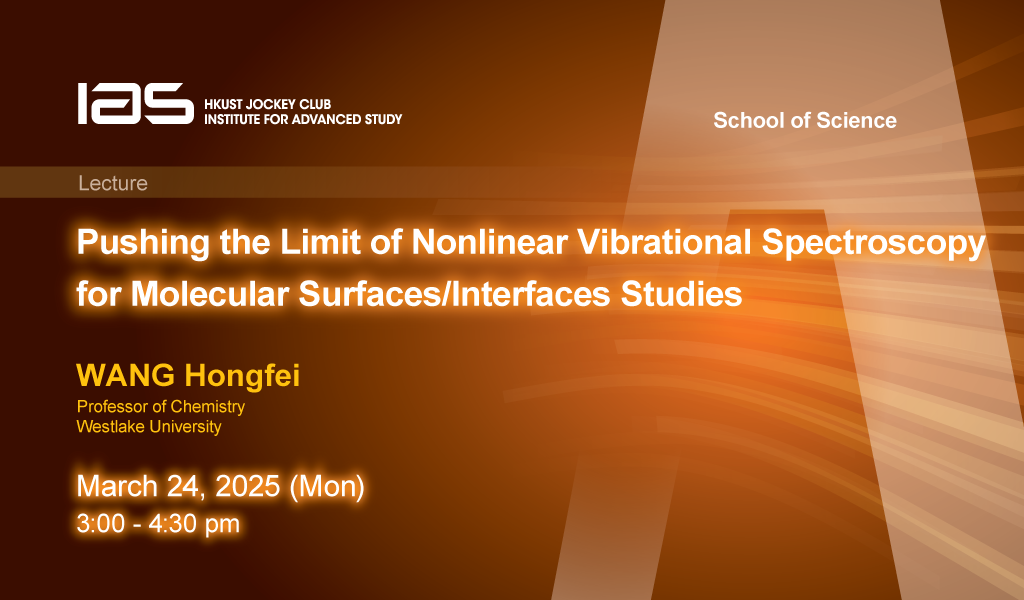