We consider the general $f$-divergence formulation of bidirectional generative modeling, which includes VAE and BiGAN as special cases. We present a new optimization method for this formulation, where the gradient is computed using an adversarially learned discriminator. In our framework, we show that different divergences induce similar algorithms in terms of gradient evaluation, except with different scaling. Therefore this paper gives a general recipe for a class of principled $f$-divergence based generative modeling methods. Theoretical justifications and extensive empirical studies are provided to demonstrate the advantage of our approach over existing methods.
5月15日
10:30am - 11:30am
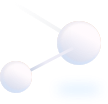
地點
https://hkust.zoom.com.cn/j/96396217133
講者/表演者
Ms. Xinwei SHEN
HKUST
HKUST
主辦單位
Department of Mathematics
聯絡方法
mathseminar@ust.hk
付款詳情
對象
Alumni, Faculty and Staff, PG Students, UG Students
語言
英語
其他活動
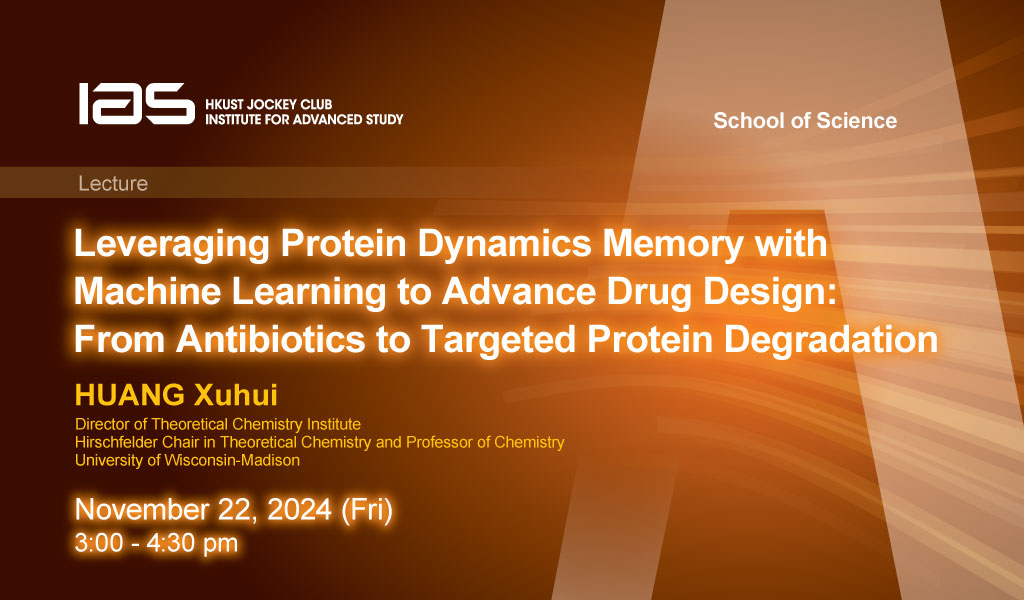
11月22日
研討會, 演講, 講座
IAS / School of Science Joint Lecture - Leveraging Protein Dynamics Memory with Machine Learning to Advance Drug Design: From Antibiotics to Targeted Protein Degradation
Abstract
Protein dynamics are fundamental to protein function and encode complex biomolecular mechanisms. Although Markov state models have made it possible to capture long-timescale protein co...
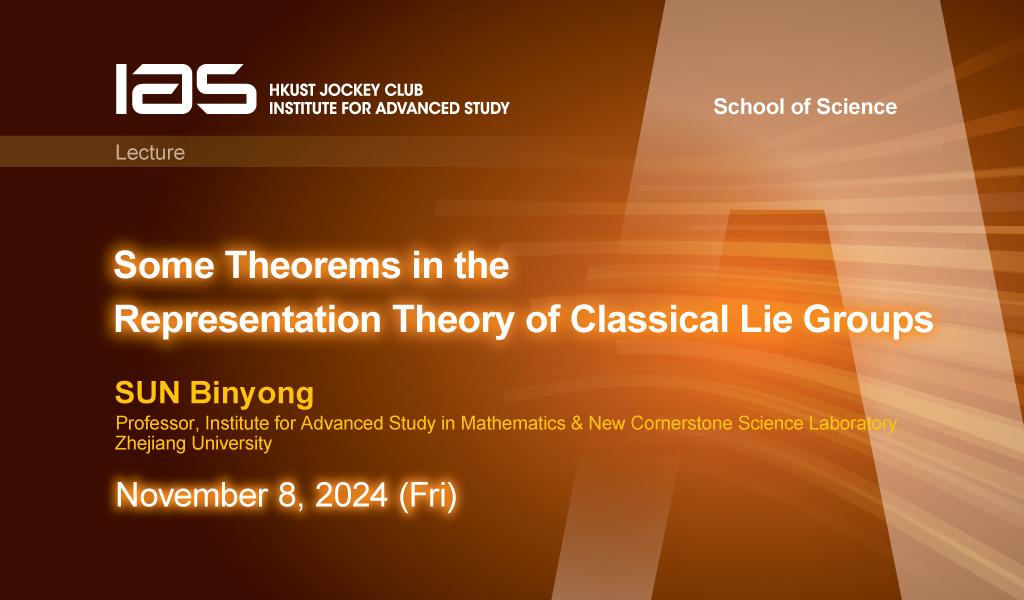
11月8日
研討會, 演講, 講座
IAS / School of Science Joint Lecture - Some Theorems in the Representation Theory of Classical Lie Groups
Abstract
After introducing some basic notions in the representation theory of classical Lie groups, the speaker will explain three results in this theory: the multiplicity one theorem for classical...