5月18日
研討會, 演講, 講座
CHEM - PhD Student Seminar - Self-Healing Materials for Energy Conversion and Storage Systems
Student: Miss Yiming AN
Department: Department of Chemistry, HKUST
Supervisor: Professor Shihe YANG
5月18日
研討會, 演講, 講座
CHEM - PhD Student Seminar - Synthesis of 2D Layered MXenes and Their Application in Zinc-Air Batteries
Student: Mr. He LIN
Department: Department of Chemistry, HKUST
Supervisor: Professor Shihe YANG
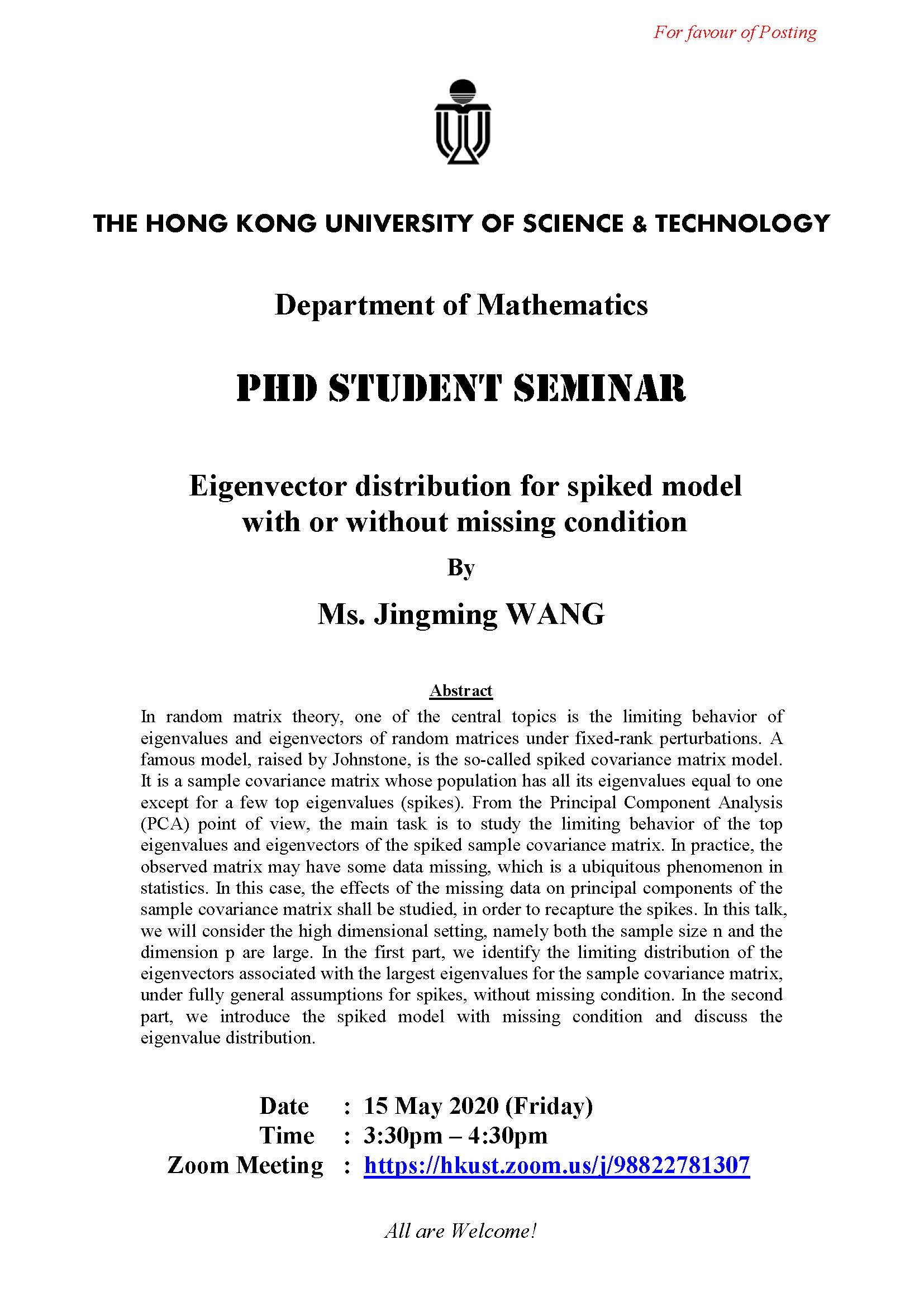
5月15日
研討會, 演講, 講座
MATH_PhD Student Seminar - Eigenvector distribution for spiked model with or without missing condition
In random matrix theory, one of the central topics is the limiting behavior of eigenvalues and eigenvectors of random matrices under fixed-rank perturbations. A famous model, raised by Johnstone, is the so-called spiked covariance matrix model.
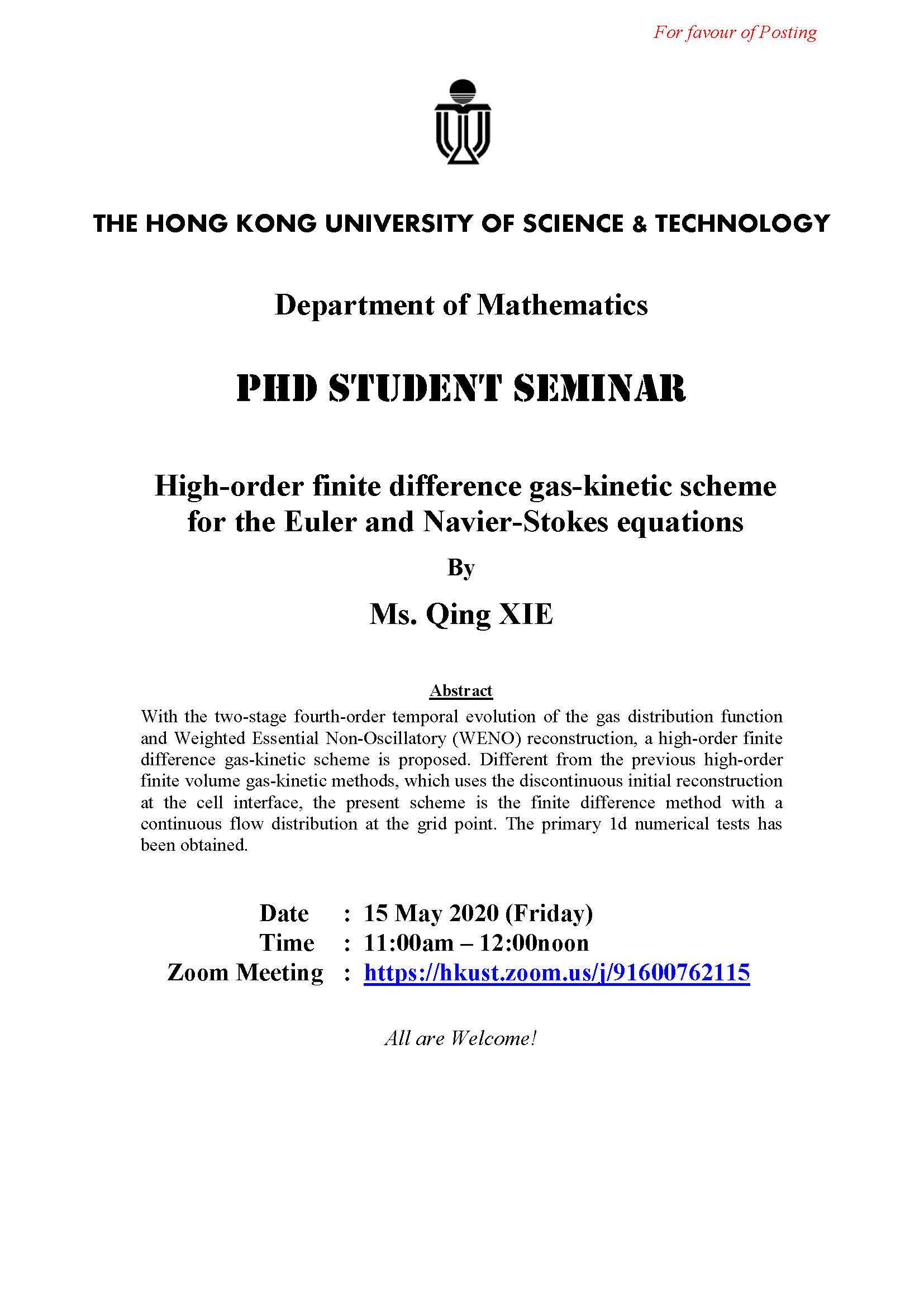
5月15日
研討會, 演講, 講座
MATH_PhD Student Seminar - High-order finite difference gas-kinetic scheme for the Euler and Navier-Stokes equations
With the two-stage fourth-order temporal evolution of the gas distribution function and Weighted Essential Non-Oscillatory (WENO) reconstruction, a high-order finite difference gas-kinetic scheme is proposed.
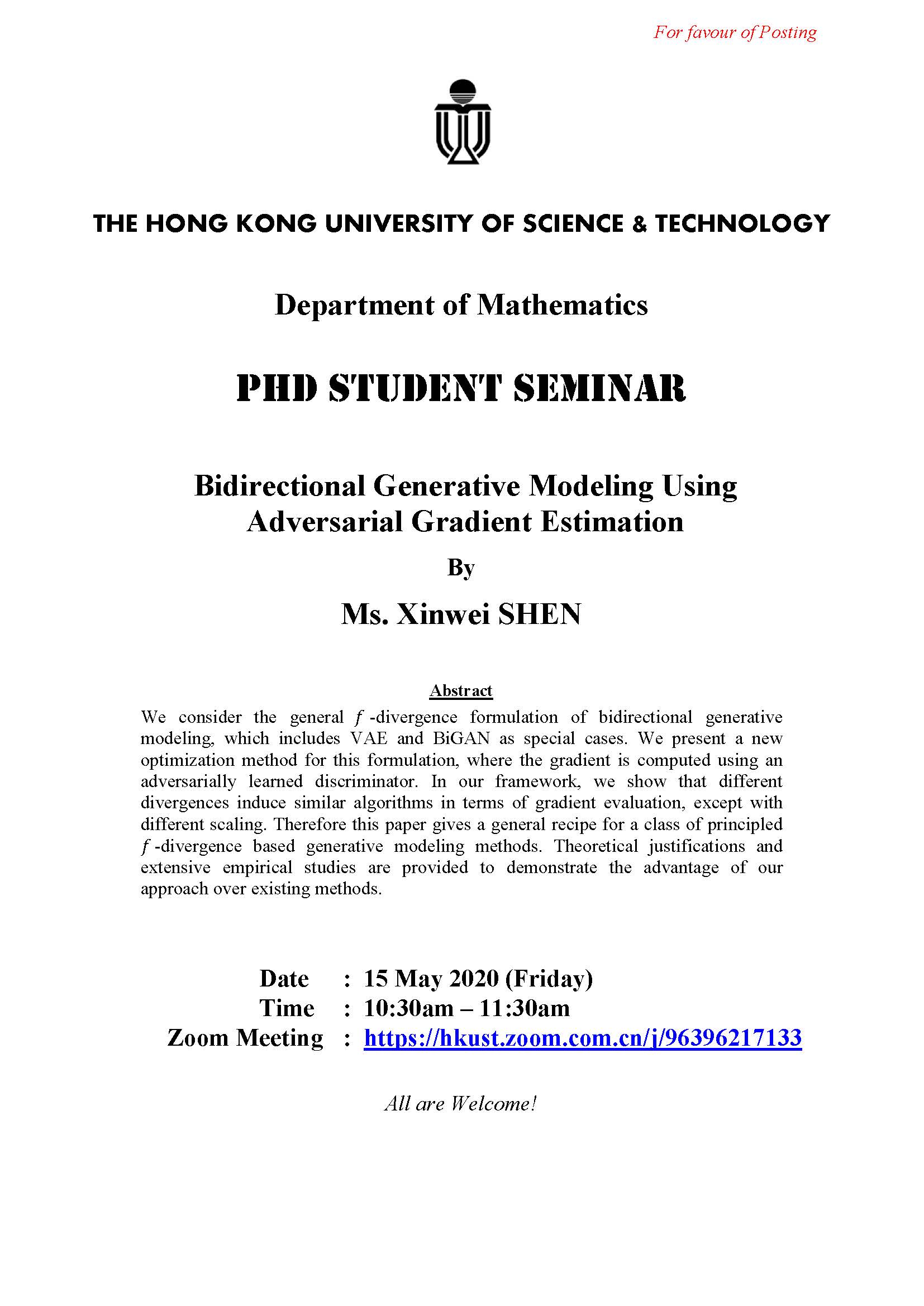
5月15日
研討會, 演講, 講座
MATH_PhD Student Seminar - Bidirectional Generative Modeling Using Adversarial Gradient Estimation
We consider the general $f$-divergence formulation of bidirectional generative modeling, which includes VAE and BiGAN as special cases. We present a new optimization method for this formulation, where the gradient is computed using an adversarially learned discriminator.
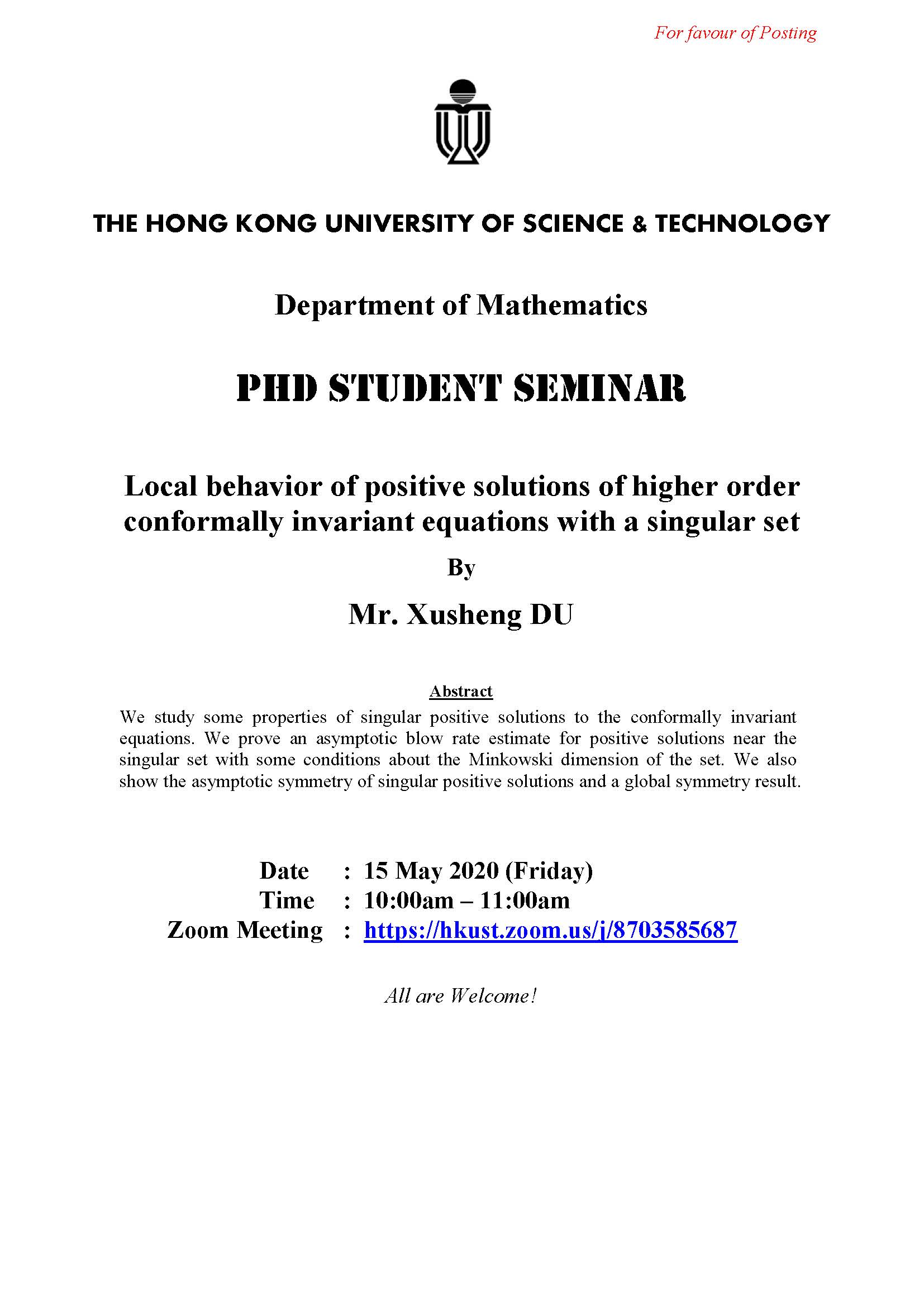
5月15日
研討會, 演講, 講座
MATH_PhD Student Seminar - Local behavior of positive solutions of higher order conformally invariant equations with a singular set
We study some properties of singular positive solutions to the conformally invariant equations. We prove an asymptotic blow rate estimate for positive solutions near the singular set with some conditions about the Minkowski dimension of the set.

5月15日
研討會, 演講, 講座
MATH_PhD Student Seminar - Black-Box Adversarial Attack
Current neural network-based classifiers are susceptible to adversarial examples even in the black-box setting, where the attacker could only query the output of the network. We present a new method for black-box adversarial attack.
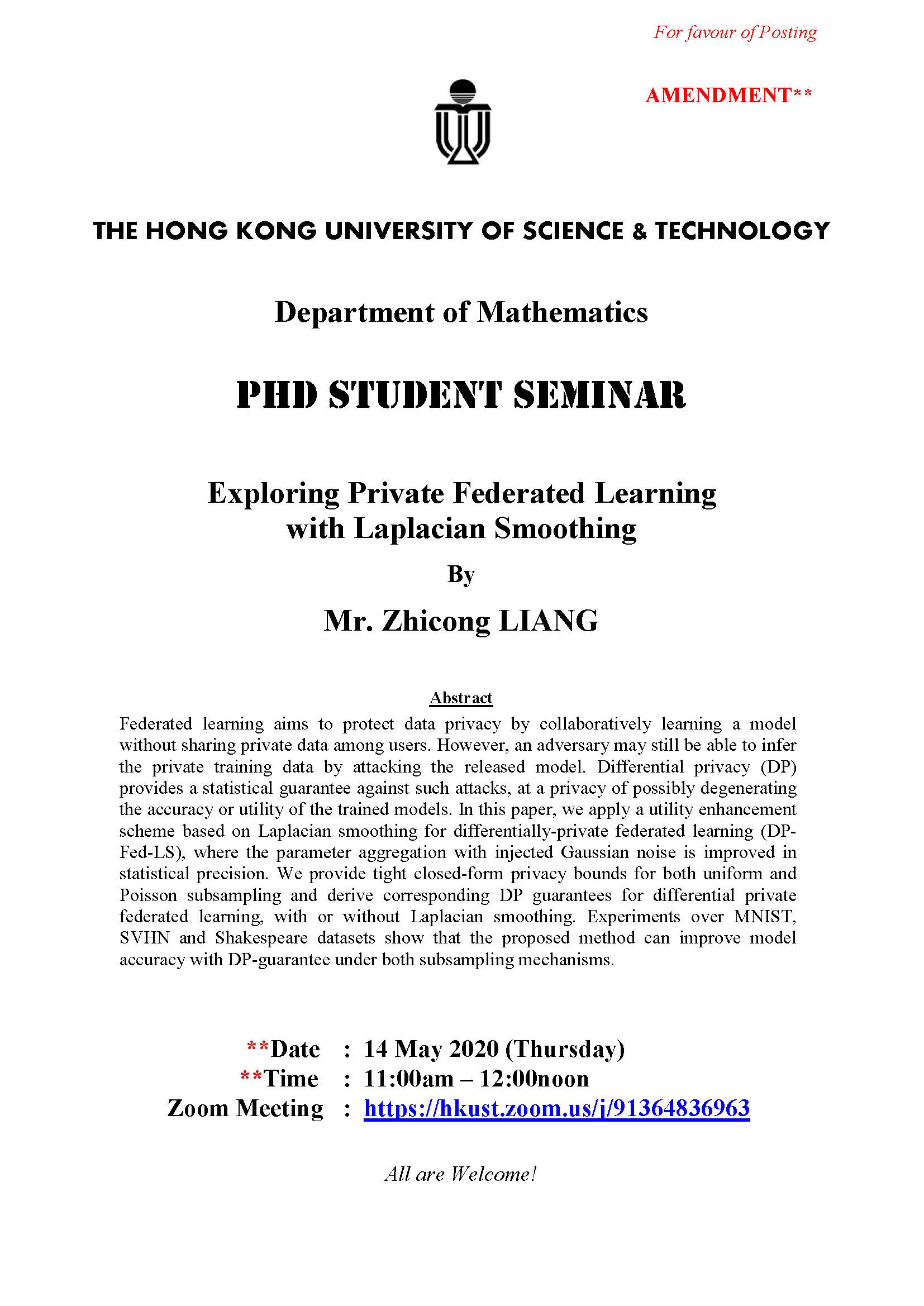
5月14日
研討會, 演講, 講座
MATH_PhD Student Seminar - Exploring Private Federated Learning with Laplacian Smoothing
Federated learning aims to protect data privacy by collaboratively learning a model without sharing private data among users. However, an adversary may still be able to infer the private training data by attacking the released model.
瀏覽理學院過往舉辦的活動。