Early stopping is a widely-used regularization technique to avoid overfitting in iterative algorithms. In particular, Split Linearized Bregman Iteration methods are often equipped with an early stopping rule to achieve model selection consistency to recover the structural sparsity of parameters. However, theoretical early stopping rule with model selection consistency requires the incoherence condition, which is unknown in applications. In this work, we propose a data adaptive early stopping rule towards the False Discovery Rate (FDR) control under the framework of Knockoff methods. An inflated FDR is proved under a relaxation of the exchangeability condition in traditional Knockoff methods. The effectiveness of the proposed method is demonstrated by both simulations and two real world application examples, Alzheimer’s Disease (AD) and partial order ranking of basketball teams.
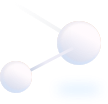
HKUST
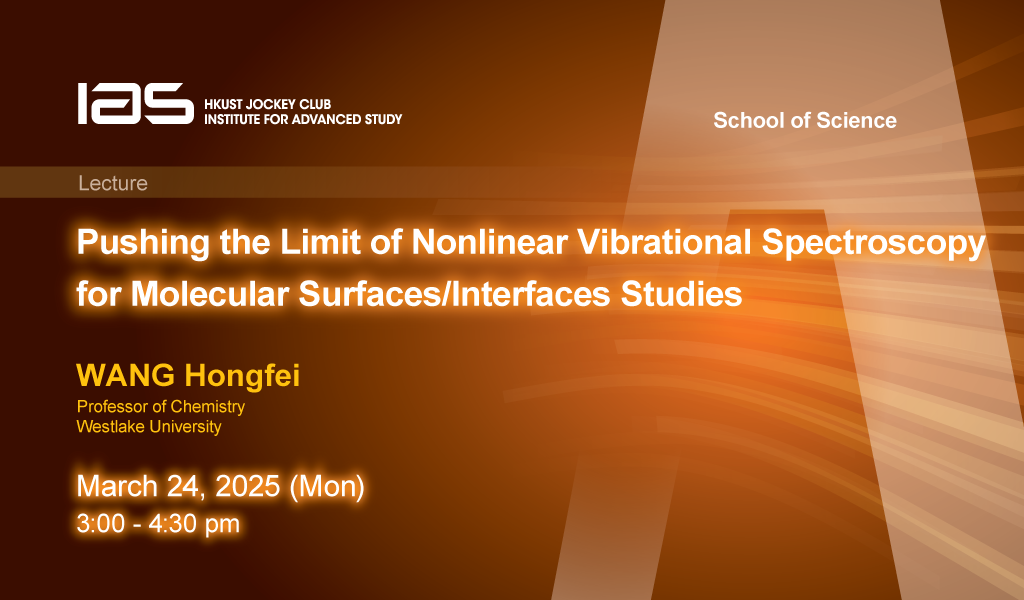
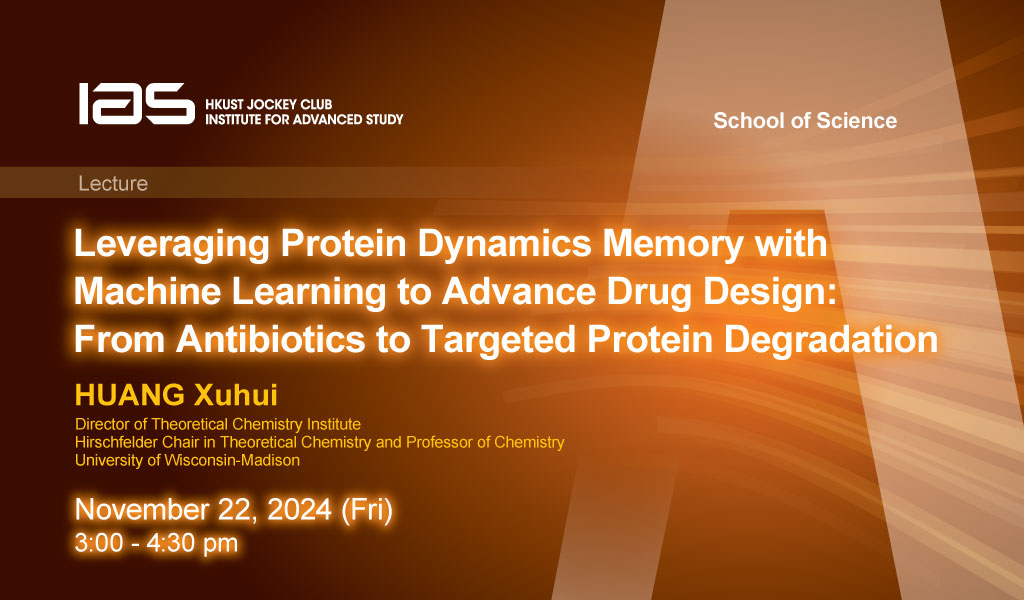