In the Gaussian sequence model , we study the likelihood ratio test (LRT) for testing
versus
, where
, and
is a closed convex set in
. In particular, we show that under the null hypothesis, normal approximation holds for the log-likelihood ratio statistic for a general pair
, in the high dimensional regime where the estimation error of the associated least squares estimator diverges in an appropriate sense. The normal approximation further leads to a precise characterization of the power behavior of the LRT in the high dimensional regime. These characterizations show that the power behavior of the LRT is in general non-uniform with respect to the Euclidean metric, and illustrate the conservative nature of existing minimax optimality and sub-optimality results for the LRT. A variety of examples, including testing in the orthant/circular cone, isotonic regression, Lasso, and testing parametric assumptions versus shape-constrained alternatives, are worked out to demonstrate the versatility of the developed theory.
This talk is based on joint work with Yandi Shen(UW, Chicago) and Bodhisattva Sen(Columbia).
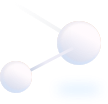
Rutgers University
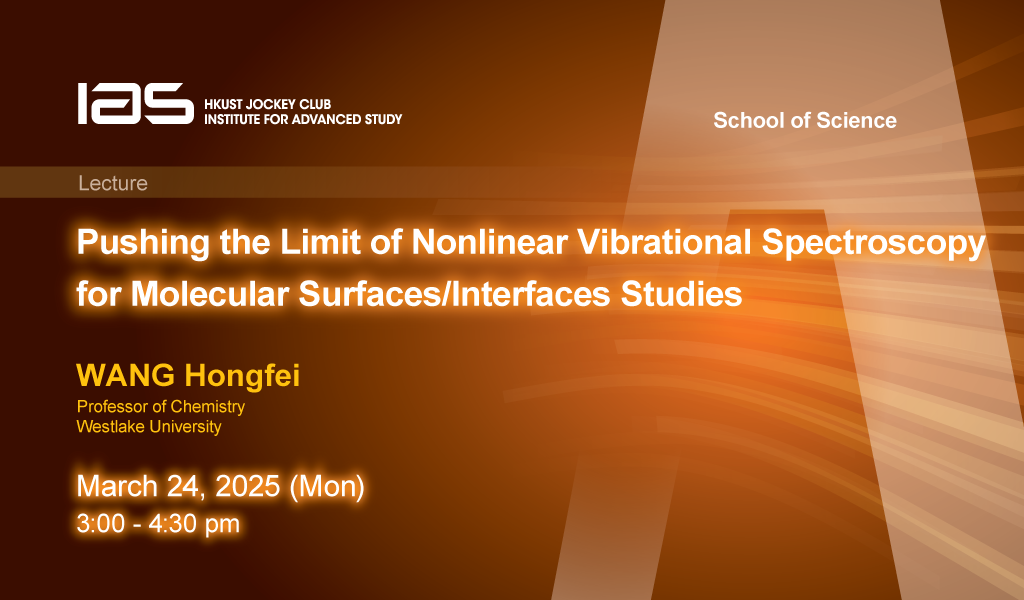
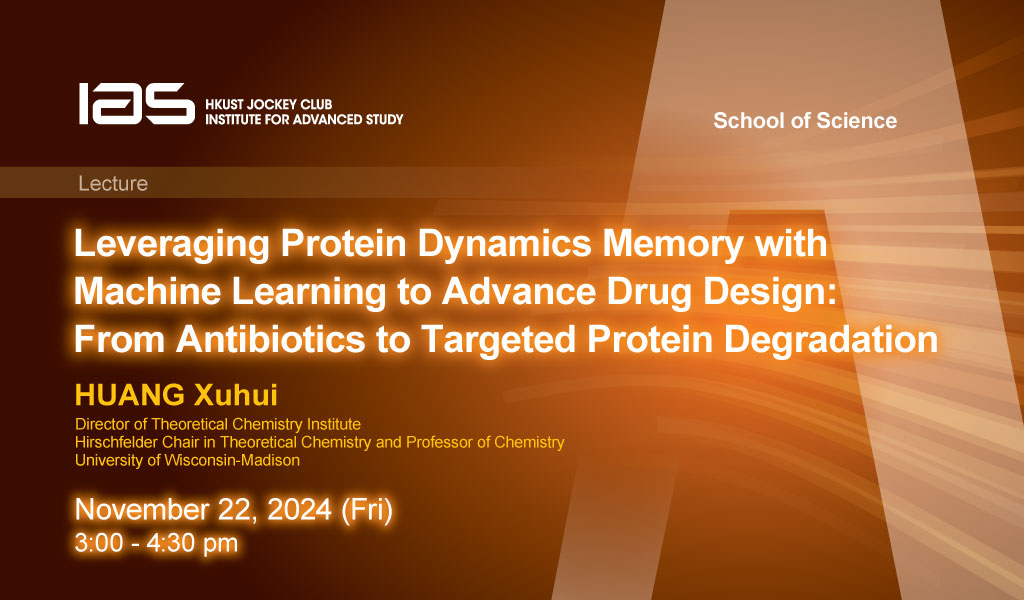