Current neural networks can be easily attacked by small artificially chosen noise called adversarial examples. Although adversarial training and its variants currently constitute the most effective way to achieve robustness to adversarial attacks, their poor generalization limits their performance on the test samples. In this seminar, I will talk about a method to improve the generalization and robust accuracy of adversarially-trained networks via self-supervised test-time fine-tuning. To this end, I introduce a meta adversarial training method that incorporates the test-time fine-tuning procedure into the training phase, so as to strengthen the correlation between the self-supervised and classification tasks, which yields a good starting point for test-time fine-tuning. The extensive experiments on CIFAR10 and STL10 using different self-supervised tasks show that the method consistently improves the robust accuracy under different attack strategies for both the white-box and black-box attacks.
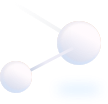
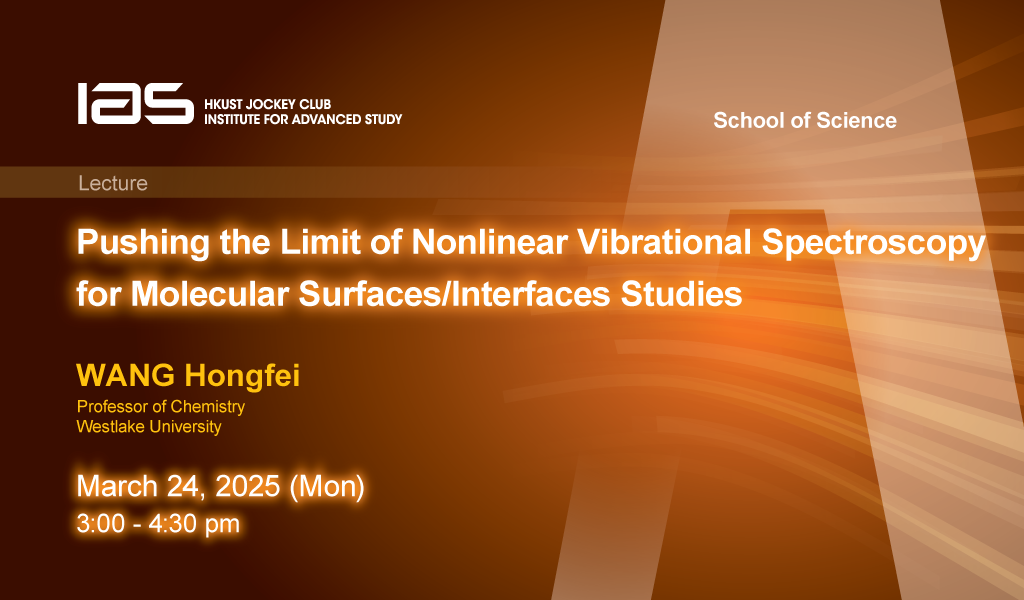
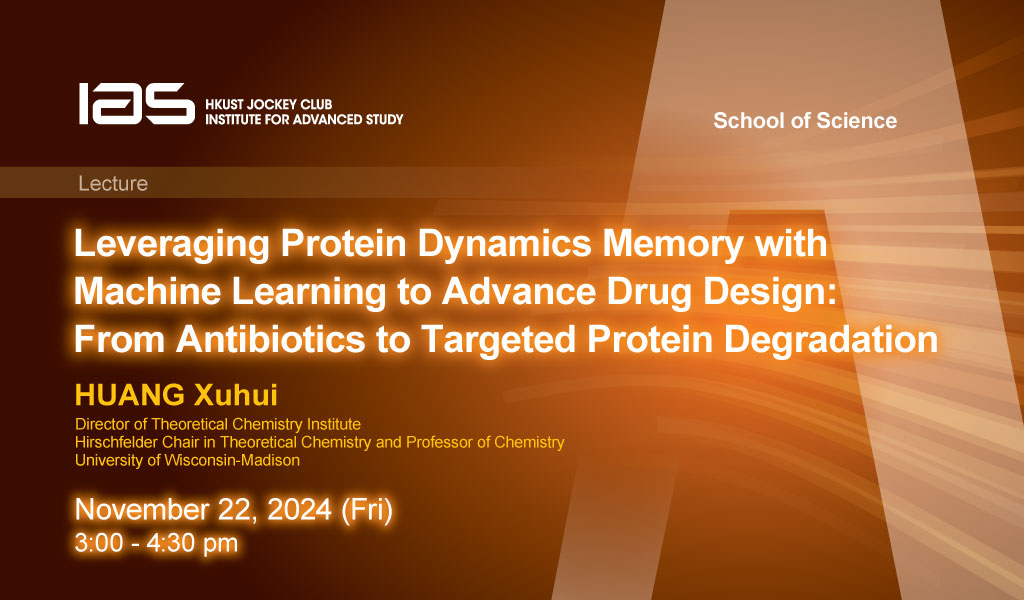