Differentially private synthetic data provide a powerful mechanism to enable data analysis while protecting sensitive information about individuals. We first present a highly effective algorithmic approach for generating differentially private synthetic data in a bounded metric space with near-optimal utility guarantees under the Wasserstein distance. When the data lie in a high-dimensional space, the accuracy of the synthetic data suffers from the curse of dimensionality. We then propose an algorithm to generate low-dimensional private synthetic data efficiently from a high-dimensional dataset. A key step in our algorithm is a private principal component analysis (PCA) procedure with a near-optimal accuracy bound. Based on joint work with Yiyun He (UC Irvine), Roman Vershynin (UC Irvine), and Thomas Strohmer (UC Davis).
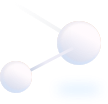
University of California, Irvine
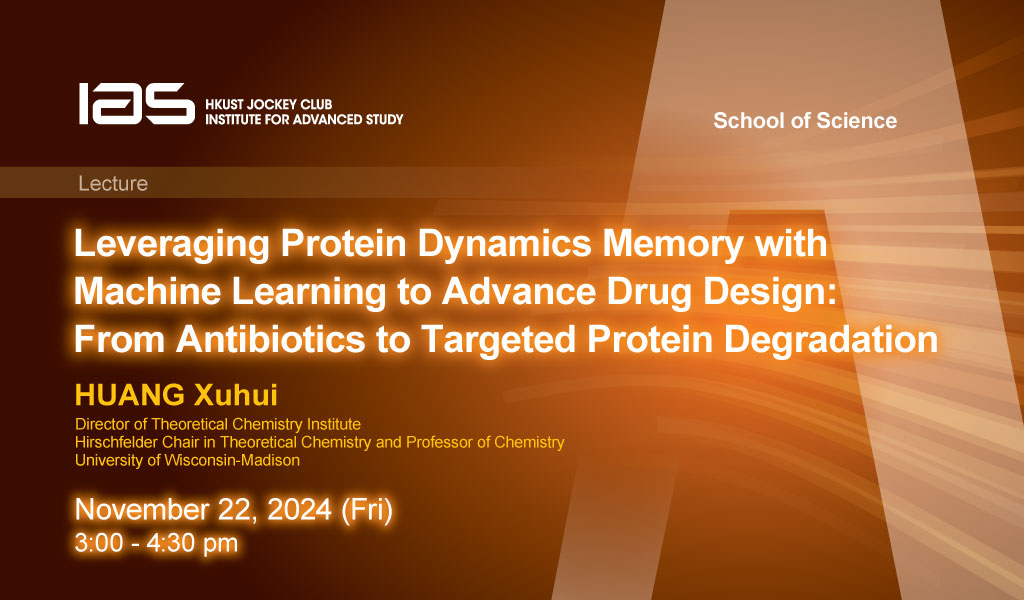
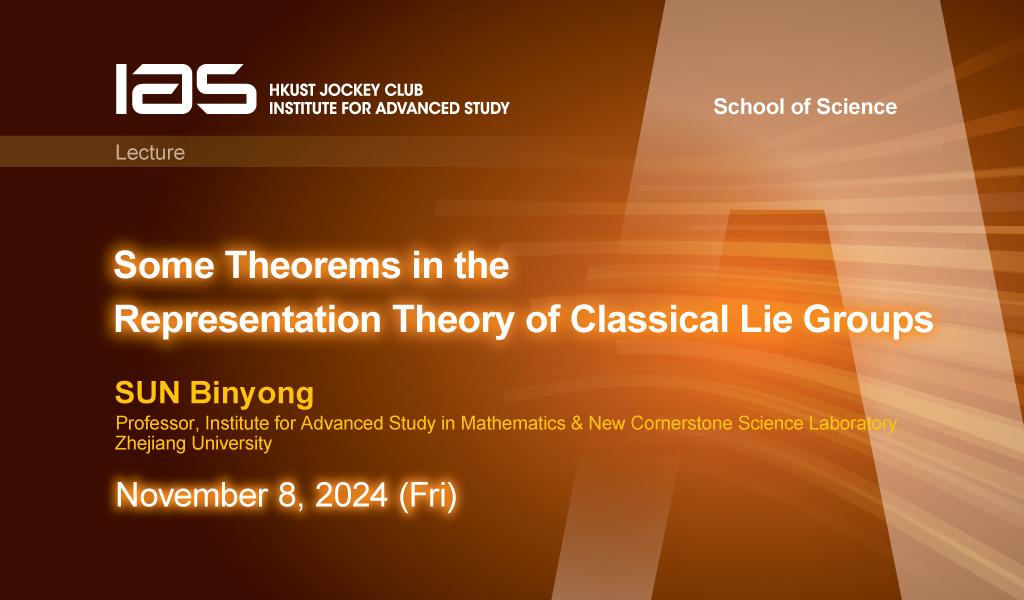