Latent variable models lay the statistical foundation for data science problems with unstructured, incomplete and heterogeneous information. Spectral methods extract low-dimensional geometric structures for downstream tasks in a computationally efficient way. Despite their conceptual simplicity and wide applicability, theoretical understanding is lagging far behind and that hinders development of principled approaches. In this talk, I will first talk about the bias and variance of PCA, and apply the results to distributed estimation of principal eigenspaces. Then I will present an $ell_p$ theory of eigenvector analysis that yields optimal recovery guarantees for spectral methods in many challenging problems. The results find applications in dimensionality reduction, mixture models, network analysis, recommendation systems, ranking and beyond.
3月20日
9:30am - 10:30am
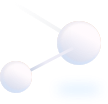
地点
https://hkust.zoom.com.cn/j/5616960008
讲者/表演者
Dr. Kaizheng WANG
Princeton University
Princeton University
主办单位
Department of Mathematics
联系方法
mathseminar@ust.hk
付款详情
对象
Alumni, Faculty and Staff, PG Students, UG Students
语言
英语
其他活动
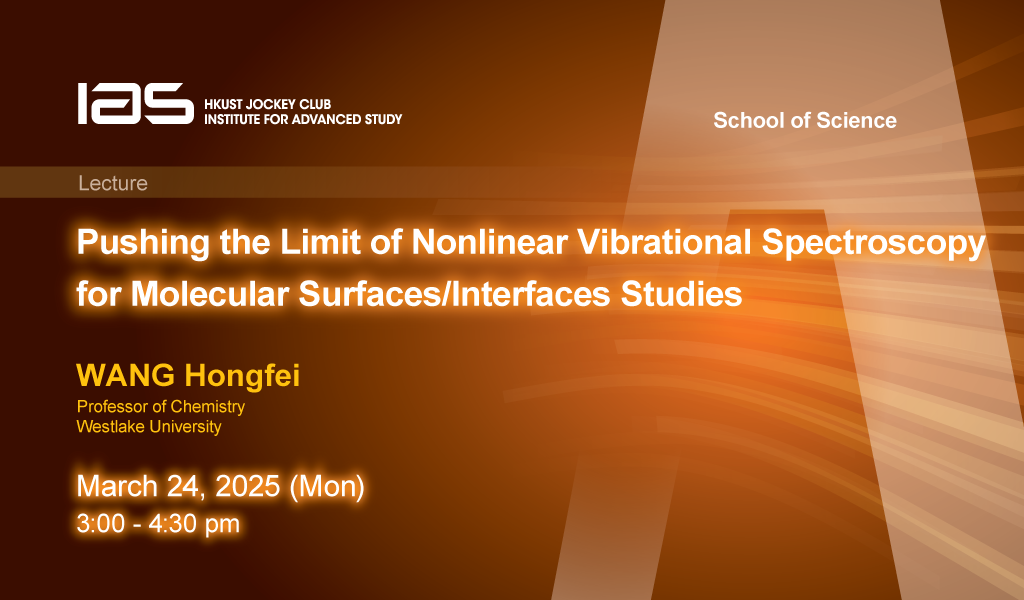
3月24日
研讨会, 演讲, 讲座
IAS / School of Science Joint Lecture - Pushing the Limit of Nonlinear Vibrational Spectroscopy for Molecular Surfaces/Interfaces Studies
Abstract
Surfaces and interfaces are ubiquitous in Nature. Sum-frequency generation vibrational spectroscopy (SFG-VS) is a powerful surface/interface selective and sub-monolayer sensitive spect...
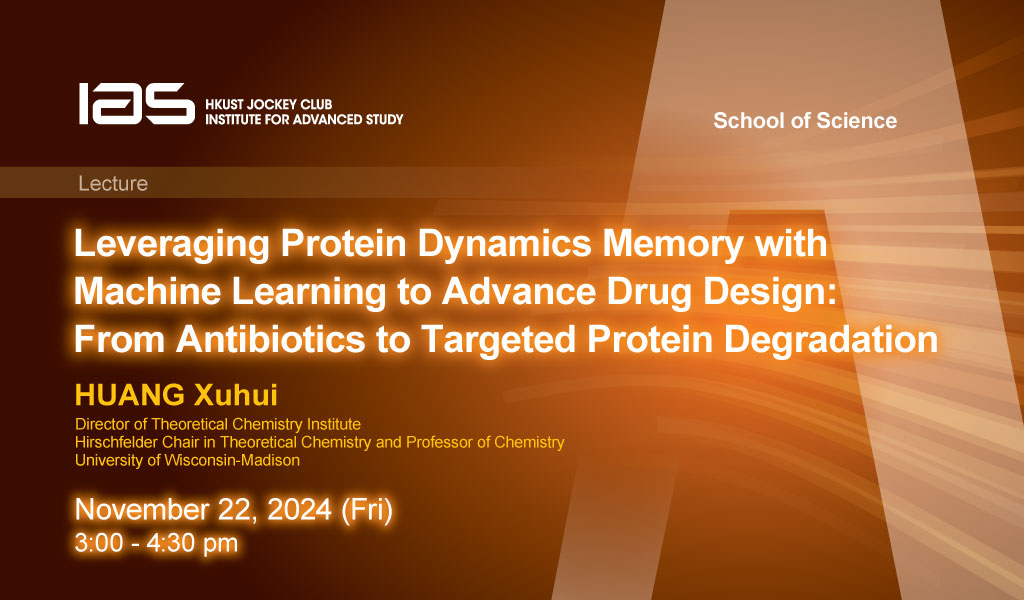
11月22日
研讨会, 演讲, 讲座
IAS / School of Science Joint Lecture - Leveraging Protein Dynamics Memory with Machine Learning to Advance Drug Design: From Antibiotics to Targeted Protein Degradation
Abstract
Protein dynamics are fundamental to protein function and encode complex biomolecular mechanisms. Although Markov state models have made it possible to capture long-timescale protein co...