Latent variable models lay the statistical foundation for data science problems with unstructured, incomplete and heterogeneous information. Spectral methods extract low-dimensional geometric structures for downstream tasks in a computationally efficient way. Despite their conceptual simplicity and wide applicability, theoretical understanding is lagging far behind and that hinders development of principled approaches. In this talk, I will first talk about the bias and variance of PCA, and apply the results to distributed estimation of principal eigenspaces. Then I will present an $ell_p$ theory of eigenvector analysis that yields optimal recovery guarantees for spectral methods in many challenging problems. The results find applications in dimensionality reduction, mixture models, network analysis, recommendation systems, ranking and beyond.
20 Mar 2020
9:30am - 10:30am
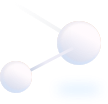
Where
https://hkust.zoom.com.cn/j/5616960008
Speakers/Performers
Dr. Kaizheng WANG
Princeton University
Princeton University
Organizer(S)
Department of Mathematics
Contact/Enquiries
mathseminar@ust.hk
Payment Details
Audience
Alumni, Faculty and Staff, PG Students, UG Students
Language(s)
English
Other Events
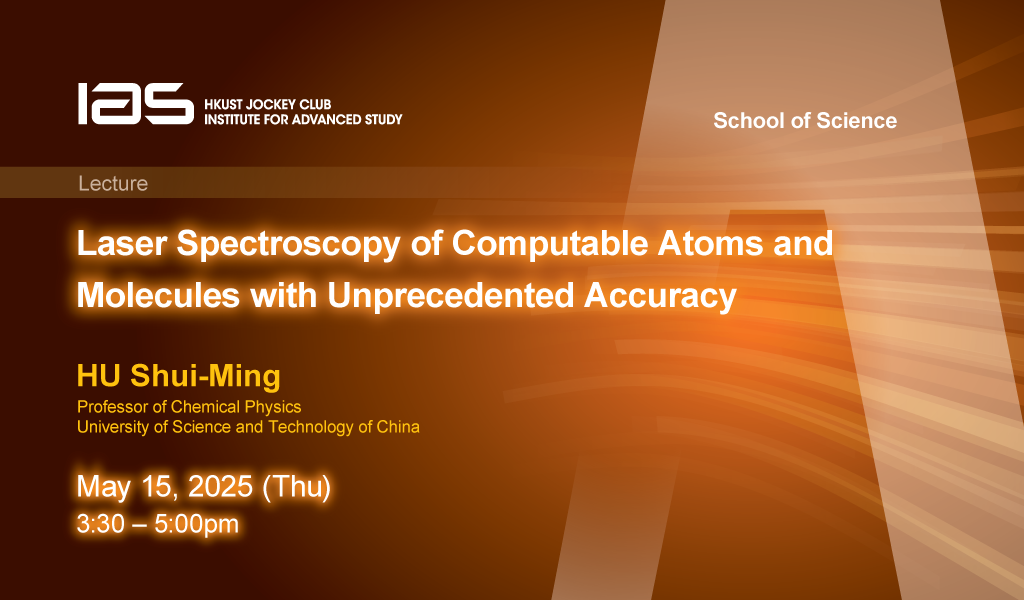
15 May 2025
Seminar, Lecture, Talk
IAS / School of Science Joint Lecture - Laser Spectroscopy of Computable Atoms and Molecules with Unprecedented Accuracy
Abstract
Precision spectroscopy of the hydrogen atom, a fundamental two-body system, has been instrumental in shaping quantum mechanics. Today, advances in theory and experiment allow us to ext...
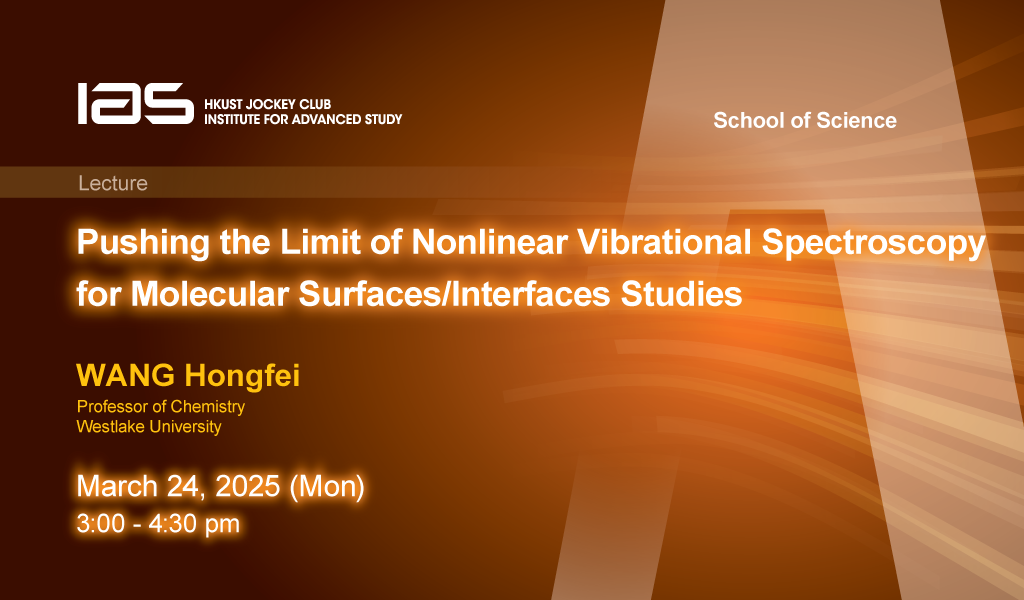
24 Mar 2025
Seminar, Lecture, Talk
IAS / School of Science Joint Lecture - Pushing the Limit of Nonlinear Vibrational Spectroscopy for Molecular Surfaces/Interfaces Studies
Abstract
Surfaces and interfaces are ubiquitous in Nature. Sum-frequency generation vibrational spectroscopy (SFG-VS) is a powerful surface/interface selective and sub-monolayer sensitive spect...