A recent breakthrough in deep learning theory shows that the training of over-parameterized deep neural networks (DNNs) can be characterized by the neural tangent kernel (NTK). However, existing optimization and generalization guarantees for deep neural networks (DNNs) typically require a network width larger than a high degree polynomial of the training sample size $n$ and the inverse of the target accuracy $epsilon^{-1}$. In this talk, I will discuss how this over-parameterization condition can be improved to more practical settings. Specifically, I will first explain why over-parameterized DNNs can be optimized to zero training error in the NTK regime, and then show what kind of functions can be learnt by DNNs with small test errors. Under standard NTK-type assumptions, these optimization and generalization guarantees hold with network width polylogarithmic in $n$ and $epsilon^{-1}$.
30 Apr 2020
3:00pm - 4:00pm
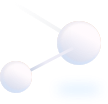
Where
https://hkust.zoom.com.cn/j/5616960008
Speakers/Performers
Dr. Yuan CAO
University of California at Los Angeles
University of California at Los Angeles
Organizer(S)
Department of Mathematics
Contact/Enquiries
mathseminar@ust.hk
Payment Details
Audience
Alumni, Faculty and Staff, PG Students, UG Students
Language(s)
English
Other Events
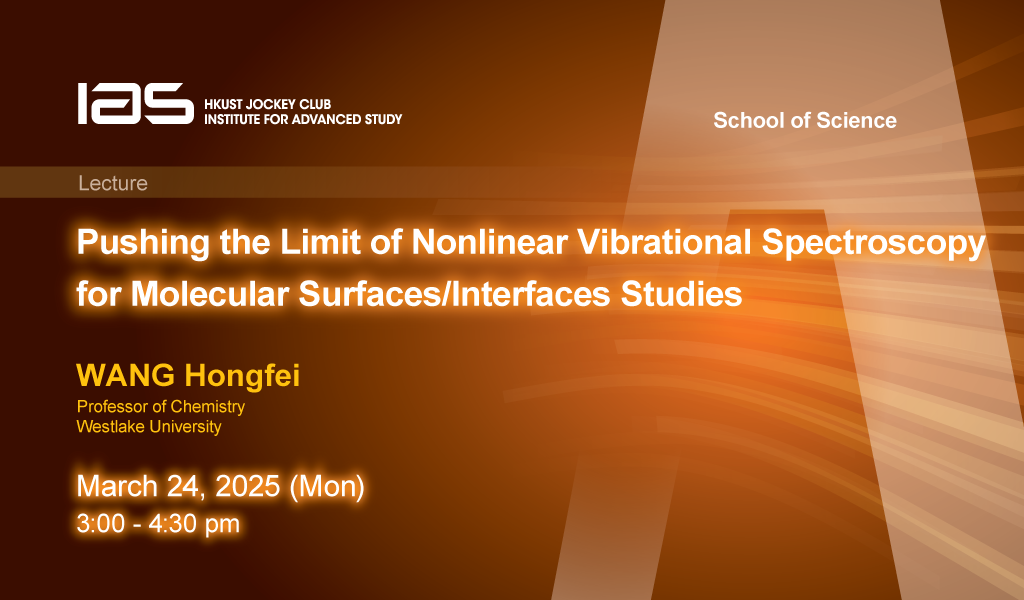
24 Mar 2025
Seminar, Lecture, Talk
IAS / School of Science Joint Lecture - Pushing the Limit of Nonlinear Vibrational Spectroscopy for Molecular Surfaces/Interfaces Studies
Abstract
Surfaces and interfaces are ubiquitous in Nature. Sum-frequency generation vibrational spectroscopy (SFG-VS) is a powerful surface/interface selective and sub-monolayer sensitive spect...
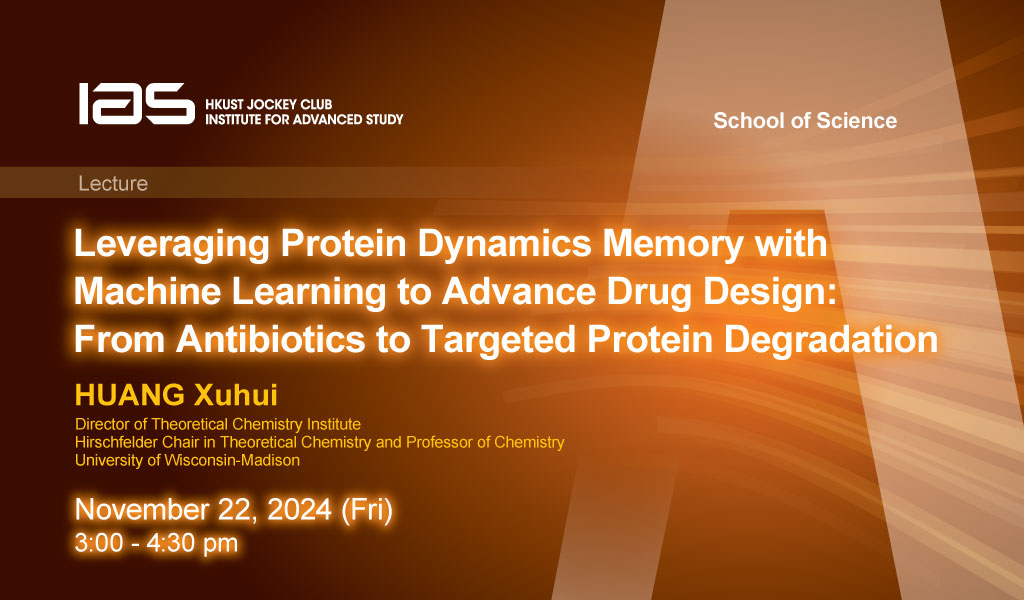
22 Nov 2024
Seminar, Lecture, Talk
IAS / School of Science Joint Lecture - Leveraging Protein Dynamics Memory with Machine Learning to Advance Drug Design: From Antibiotics to Targeted Protein Degradation
Abstract
Protein dynamics are fundamental to protein function and encode complex biomolecular mechanisms. Although Markov state models have made it possible to capture long-timescale protein co...