In this work, we propose two kinds of neural networks inspired by power method and inverse power method to solve linear eigenvalue problems. These neural networks share similar ideas with traditional methods, in which the differential operator is realized by automatic differentiation. The eigenfunction of the eigenvalue problem is learned by the neural network and the iterative algorithms are implemented by optimizing the specially defined loss function. The largest positive eigenvalue, smallest eigenvalue and interior eigenvalues with the given prior knowledge can be solved efficiently. We examine the applicability and accuracy of our methods in the numerical experiments in one dimension, two dimension and higher dimensions. Numerical results show that accurate eigenvalue and eigenfunction approximations can be obtained by our methods.
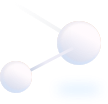
Sichuan University
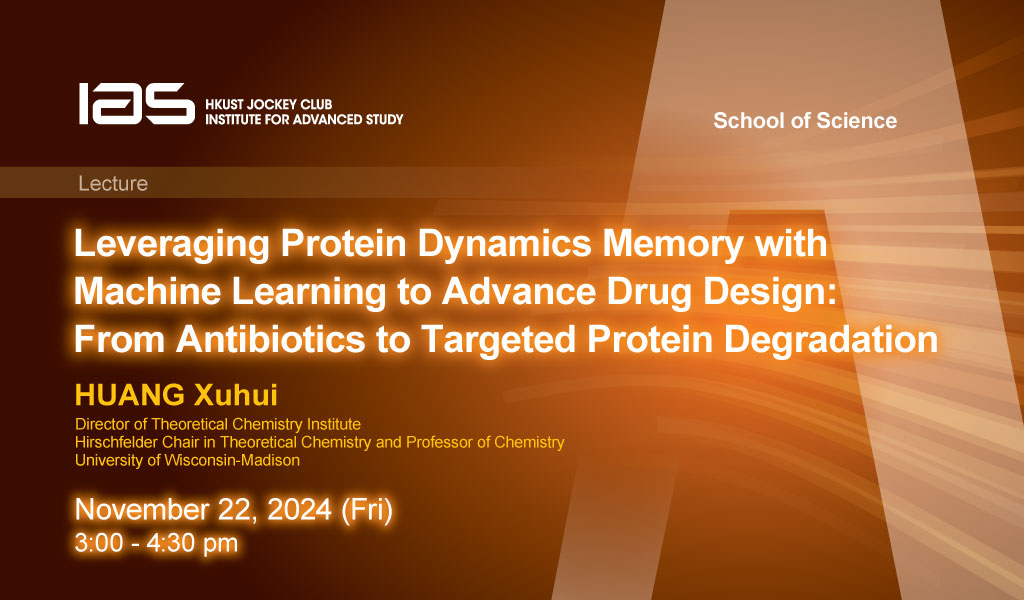
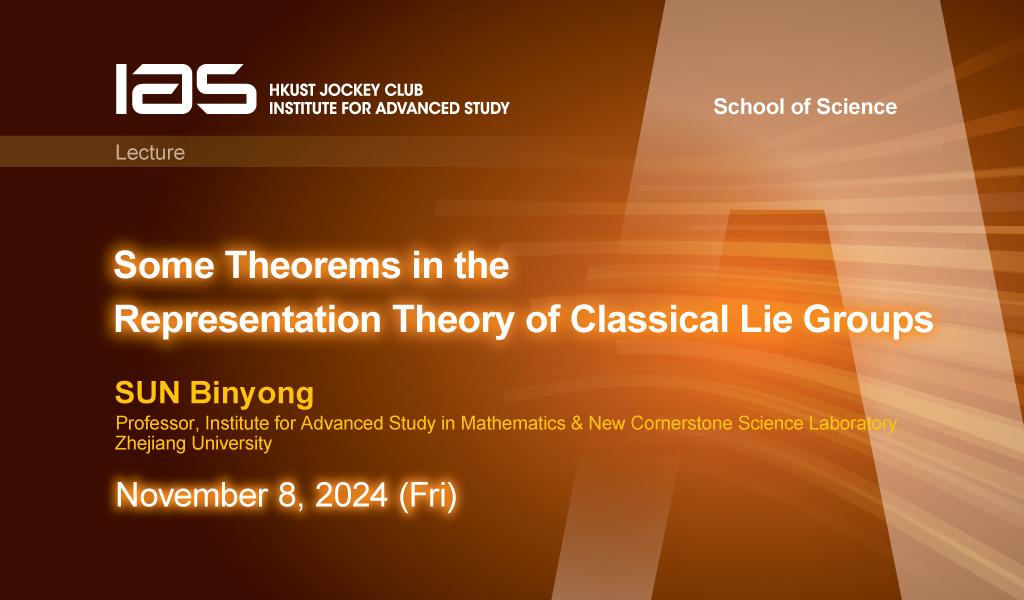