
5月4日
研讨会, 演讲, 讲座
Department of Mathematics - PhD Student Seminar - Integration of single-cell atlases with generative adversarial networks
As single-cell technologies evolved over years, diverse single-cell atlas datasets have been rapidly accumulated. Integrative analyses harmonizing such datasets provide opportunities for gaining deep biological insights.
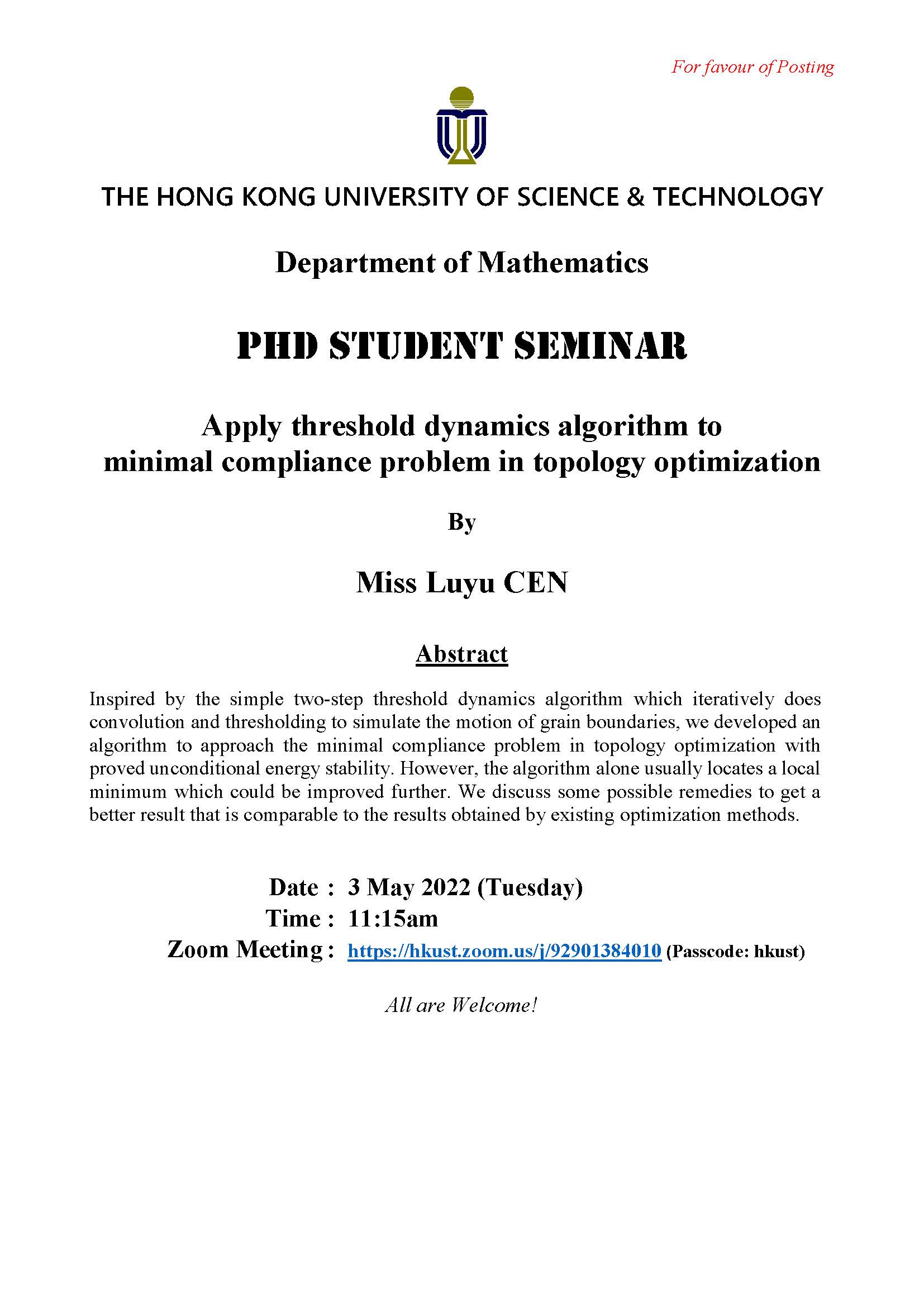
5月3日
研讨会, 演讲, 讲座
Department of Mathematics - PhD Student Seminar - Apply threshold dynamics algorithm to minimal compliance problem in topology optimization
Inspired by the simple two-step threshold dynamics algorithm which iteratively does convolution and thresholding to simulate the motion of grain boundaries, we developed an algorithm to approach the minimal compliance problem in topology optimization with

5月2日
研讨会, 演讲, 讲座
Department of Mathematics - PhD Student Seminar - Application of Reinforcement Learning to High-frequency Market Making Strategy
With the increasing usage of the electronic limit order book (LOB) in modern financial markets, high-frequency algorithmic trading has captured over 70 percent of the whole trading volume in various financial markets.
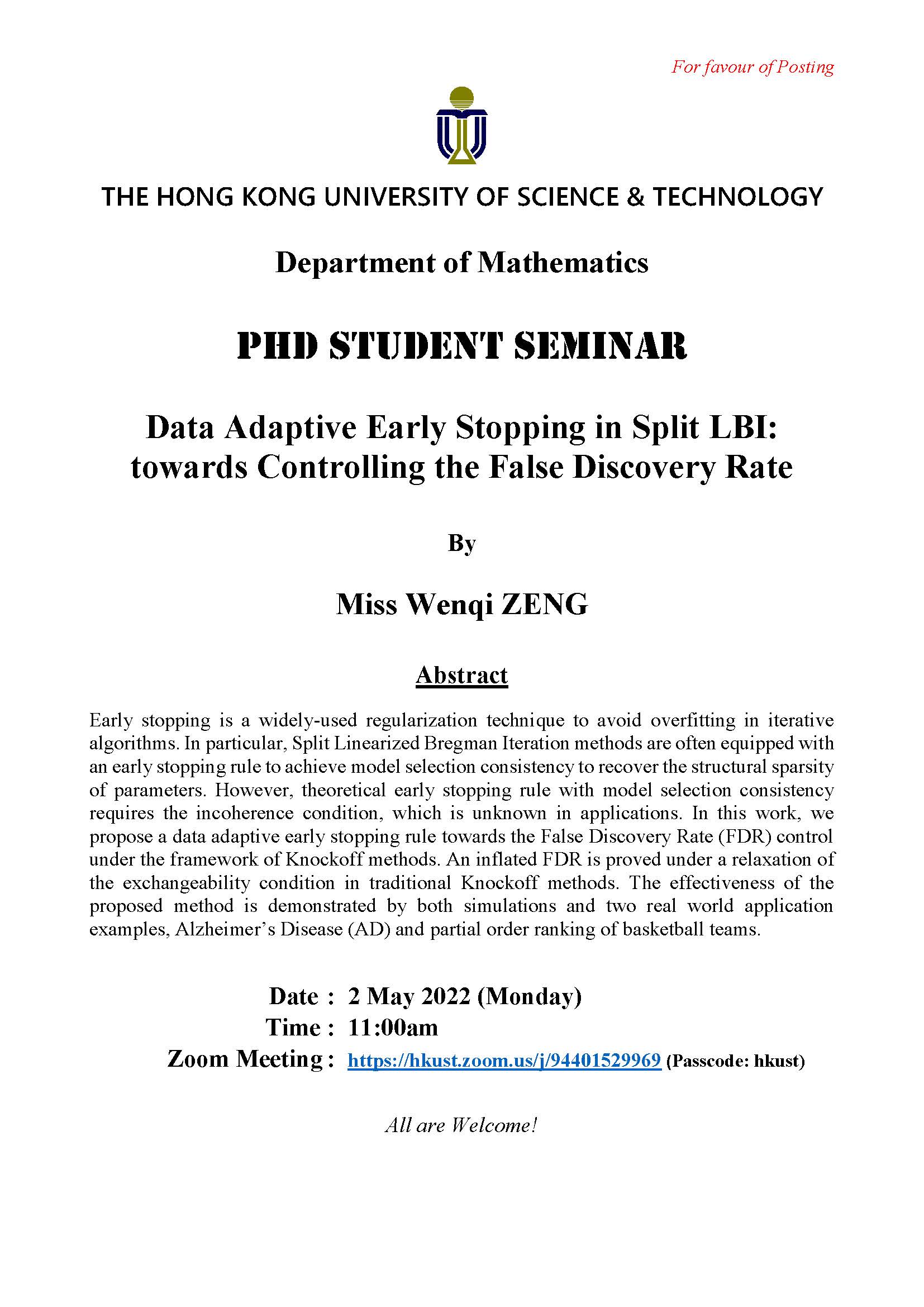
5月2日
研讨会, 演讲, 讲座
Department of Mathematics - PhD Student Seminar - Data Adaptive Early Stopping in Split LBI: towards Controlling the False Discovery Rate
Early stopping is a widely-used regularization technique to avoid overfitting in iterative algorithms.
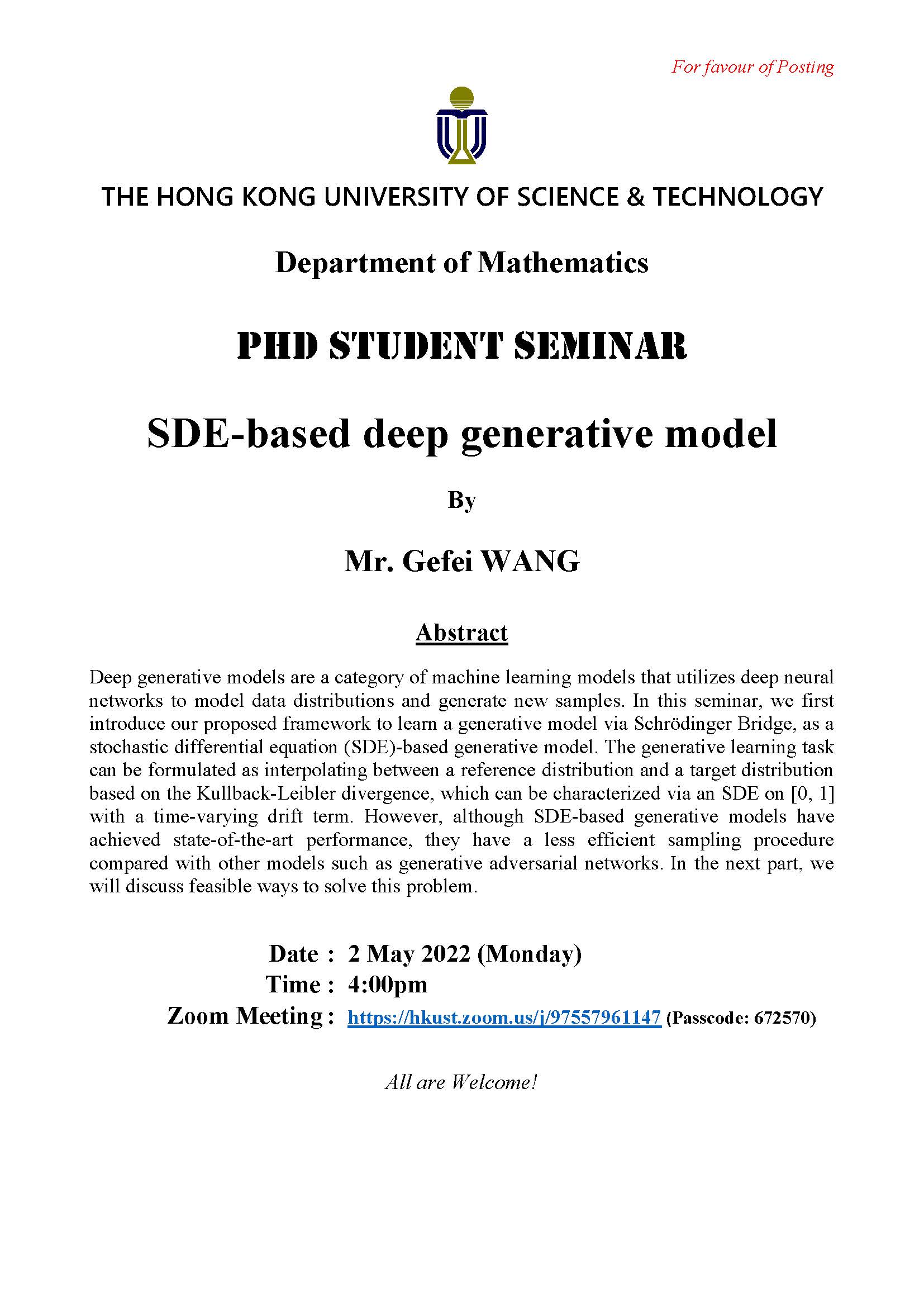
5月2日
研讨会, 演讲, 讲座
Department of Mathematics - PhD Student Seminar - SDE-based deep generative model
Deep generative models are a category of machine learning models that utilizes deep neural networks to model data distributions and generate new samples.
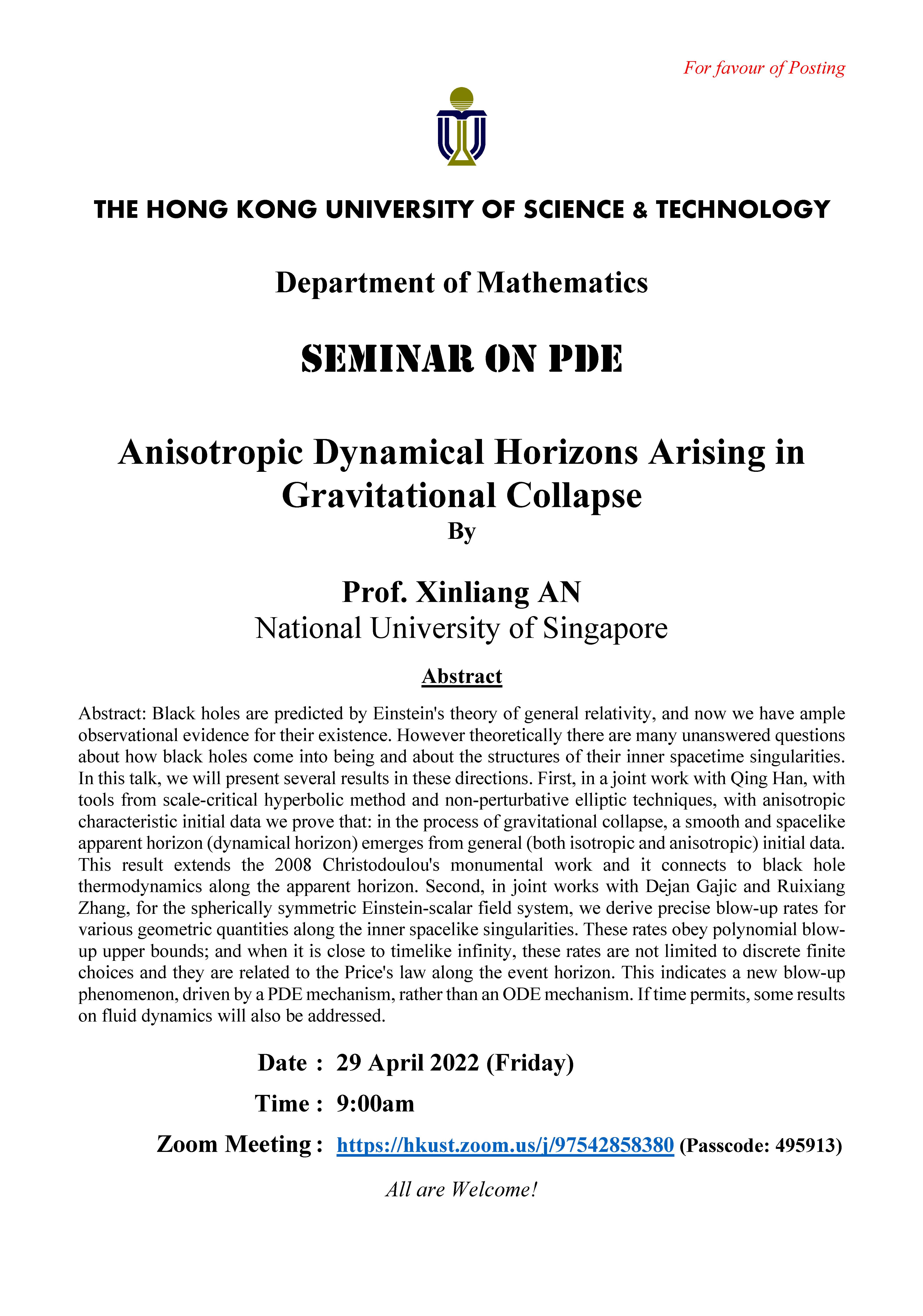
4月29日
研讨会, 演讲, 讲座
Department of Mathematics - Seminar on PDE - Anisotropic Dynamical Horizons Arising in Gravitational Collapse
Black holes are predicted by Einstein's theory of general relativity, and now we have ample observational evidence for their existence.
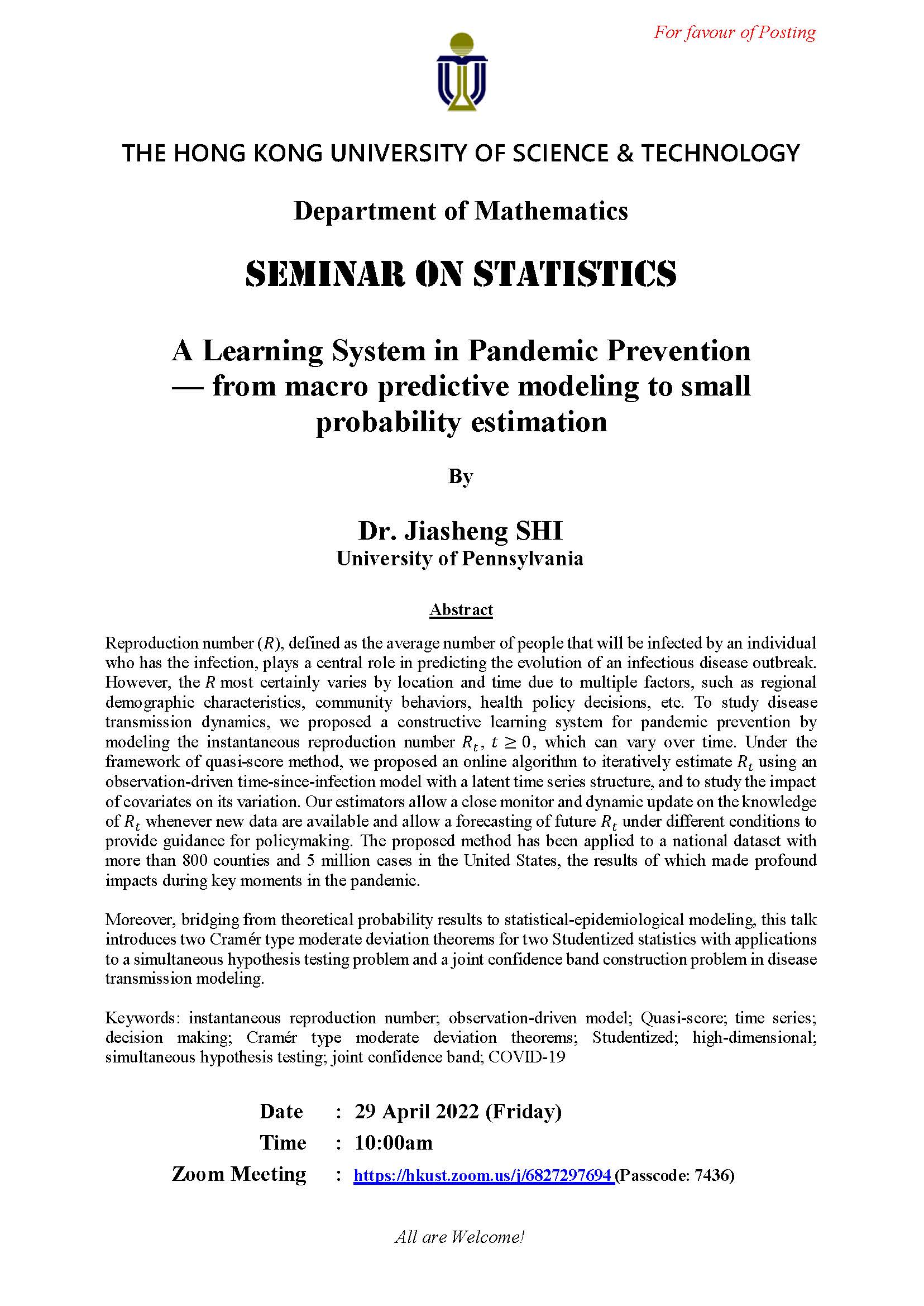
4月29日
研讨会, 演讲, 讲座
Department of Mathematics - Seminar on Statistics - A Learning System in Pandemic Prevention — from macro predictive modeling to small probability estimation
Reproduction number (R
4月29日
研讨会, 演讲, 讲座
OCES Departmental Seminar: Local to Global Drivers of Past and Future Sea-Level and Coastal Environmental Change
Geological proxies provide valuable archives of the sea-level response to past climate variability over periods of more extreme global mean surface temperatures than the brief instrumental period.
浏览理学院过往举办的活动。