Multiple comparisons in hypothesis test are often subject to structural constraints in applications. In structural Magnetic Resonance Imaging for Alzheimer's Disease, one studies not only the atrophy brain regions, but also comparisons of anatomically adjacent regions. Such constraints can be described by linear transformations of parameters whose sparsity and sign patterns are considered for directional effect estimation. Other applications include total variations, wavelet transforms, fused LASSO, and trend filtering, etc. The standard Knockoff method fails in this class of problems due to the broken anti-symmetry and exchangeability. In this paper, we propose a novel data adaptive selection scheme towards controlling the directional false discovery rate under linear transformations, the Split Knockoff method. The proposed scheme relaxes the linear manifold constraint to its neighborhood, known as variable splitting in optimization. It yields an orthogonal design, beneficial for both power and directional false discovery rate control, yet with heterogeneous noise. Exploiting an almost supermartingale, the directional false discovery rate can be controlled up to an arbitrarily small multiplicative factor. Simulation experiments and two real world applications, Alzheimer's Disease and human age ranking, are conducted to show the efficacy of the proposed method.
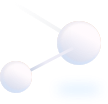
HKUST
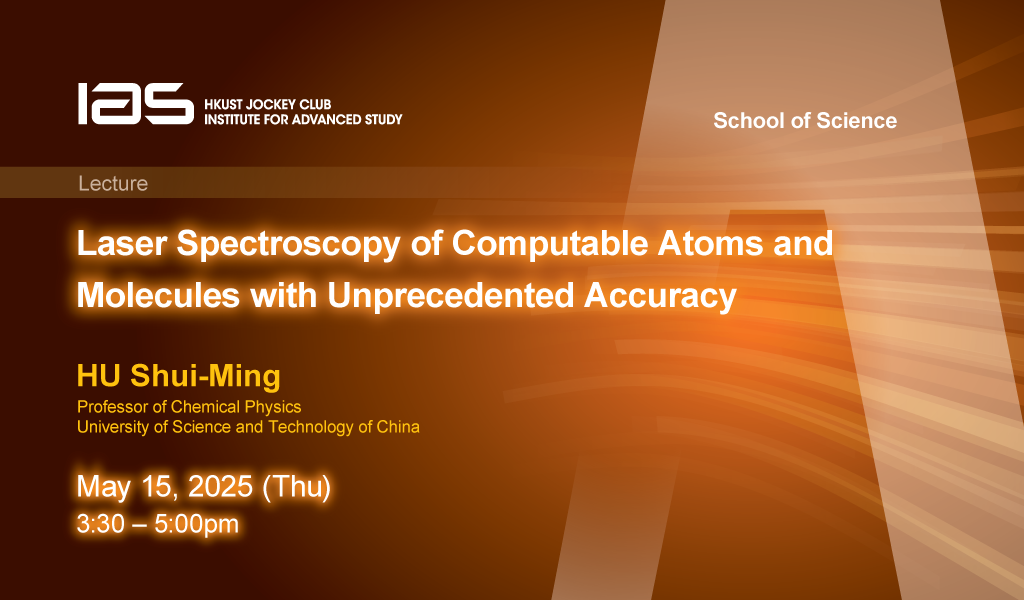
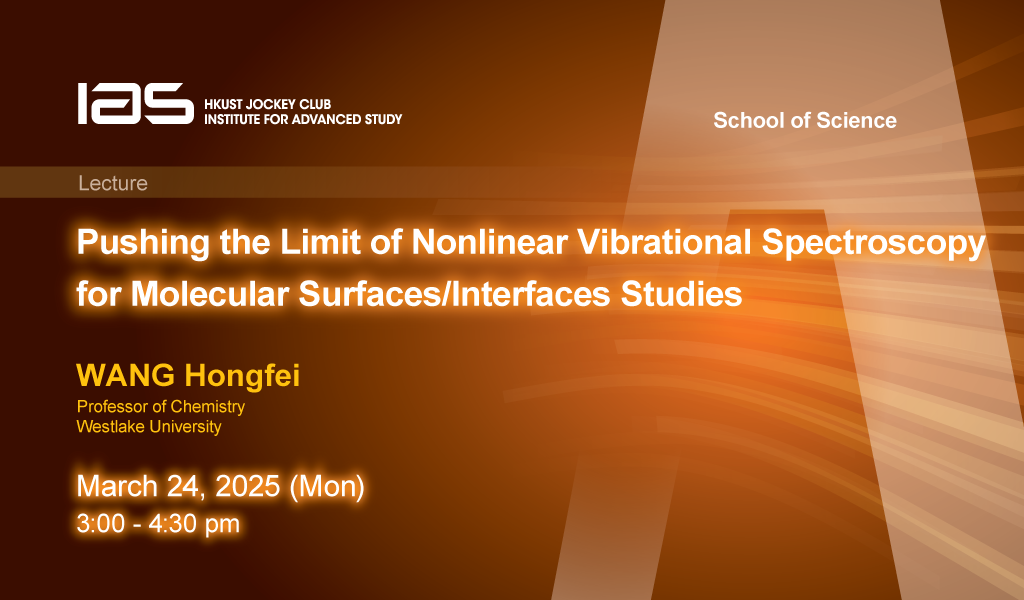