We introduce a new class of multilevel, adaptive, dual-space methods for computing fast convolutional transforms. These methods can be applied to a broad class of kernels, from the Green’s functions for classical partial differential equations (PDEs) to power functions and radial basis functions such as those used in statistics and machine learning. The DMK (dual-space multilevel kernel-splitting) framework uses a hierarchy of grids, initialized by computing a smoothed interaction at the coarsest level, followed by a sequence of corrections at finer and finer scales until the problem is entirely local, at which point direct summation is applied.
The main novelty of DMK is that the interaction at each scale is diagonalized by a short Fourier transform, permitting the use of separation of variables, but without requiring the FFT for its linear complexity. It substantially simplifies the algorithmic structure of the fast multipole methods (FMMs), unifies the tree-based algorithms such as the FMM and FFT-based algorithms such as the Ewald summation, and achieves speeds comparable to the FFT in work per gridpoint, even in a fully adaptive context.
This is joint work with Leslie Greengard.
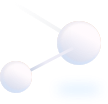
Flatiron Institute, Simons Foundation
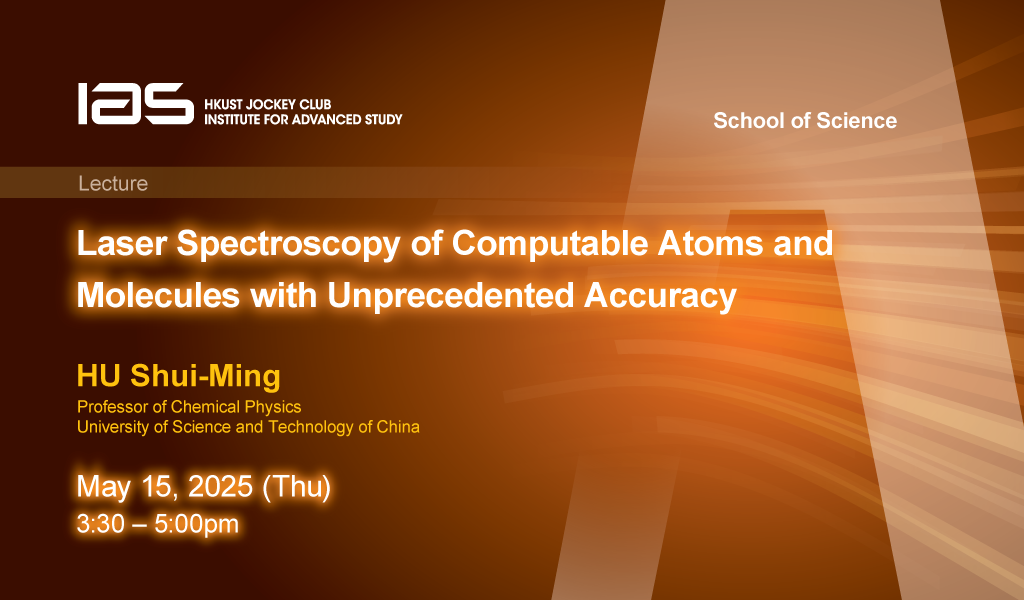