Abstract
Protein dynamics are fundamental to protein function and encode complex biomolecular mechanisms. Although Markov state models have made it possible to capture long-timescale protein conformational changes, many systems are still beyond their reach. In this lecture, the speaker will explore how incorporating dynamics memory (i.e., non-Markovian effects) into machine learning models can significantly reduce the computational cost of predicting long-time dynamics in these complex systems with high accuracy. First, the speaker will introduce the integrative-generalized master equation (IGME) method, which encodes non-Markovian dynamics through time-integrated memory kernels. Using IGME, his research team predicts multiple protein-protein interactions (PPIs) between KRAS and VHL, establishing a new workflow for PROTAC linker design in targeted protein degradation. One of the predictions matches well with the co-crystal structure, while these non-canonical PPIs remain challenging for AlphaFold3 to predict. Next, the speaker will present MEMnets, a deep-learning approach for identifying the slow collective variables (CVs) of protein dynamics. Unlike conventional deep learning models like VAMPnets, which assume Markovian dynamics, MEMnets builds on IGME theory with a novel loss function that minimizes the time integration of memory kernels. His research team demonstrates that MEMnets-derived CVs elucidate the molecular mechanisms behind the loading gate opening of bacterial RNA Polymerase (RNAP), revealing a transiently open cryptic pocket that binds the antibiotic Myx. Finally, the speaker will introduce the TS-DART method, which automatically identifies transition states (TS) across multiple free energy barriers in biomolecular systems. Inspired by Trustworthy AI, TS-DART detects TS as out-of-distribution data in a hyperspherical latent space. Using TS-DART, his research team successfully shows how antibiotic binding shifts the transition state, inhibiting bacterial RNAP elongation.
About the Speaker
Prof. HUANG Xuhui obtained his Ph.D. from Columbia University in 2006 with Prof. Bruce BERNE. He did his postdoc research at Stanford University with Profs. Michael LEVITT and Vijay PANDE. He was an Assistant, Associate and Full Professor at The Hong Kong University of Science and Technology (HKUST) between 2010 and Summer 2021. Since Fall 2021, Prof. Huang took up the position of the Hirschfelder Endowed Chair Professor in Theoretical Chemistry, and Director of Theoretical Chemistry Institute at University of Wisconsin-Madison. He has received numerous awards, including the Theory & Computation Award for Mid-Career Scientists from Biophysical Society in 2023, Pople Medal from the Asia-Pacific Association of Theoretical and Computational Chemists in 2021, American Chemical Society OpenEye Outstanding Junior Faculty Award in 2014, and Hong Kong Research Grant Council Early Career Award in 2013. He is a founding member of The Hong Kong Young Academy of Sciences (YASHK) and a Fellow of The Royal Society of Chemistry (FRSC). His group pioneered in elucidating the dynamics of protein conformational changes by developing statistical-mechanics-based methods and machine learning tools.
For Attendees' Attention
Seating is on a first come, first served basis.
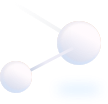
Director of Theoretical Chemistry Institute; Hirschfelder Chair in Theoretical Chemistry and Professor of Chemistry, University of Wisconsin-Madison
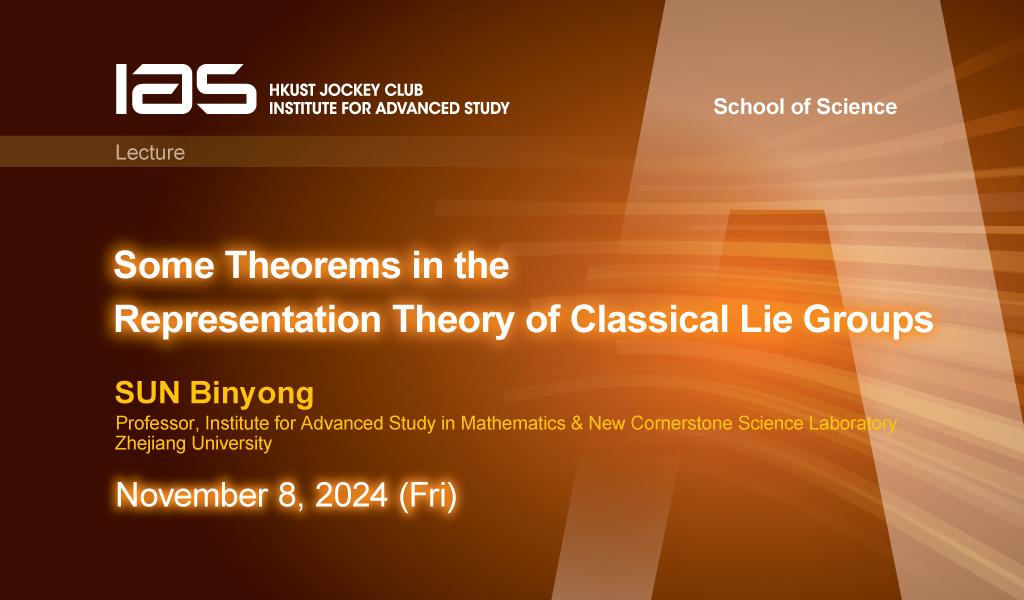
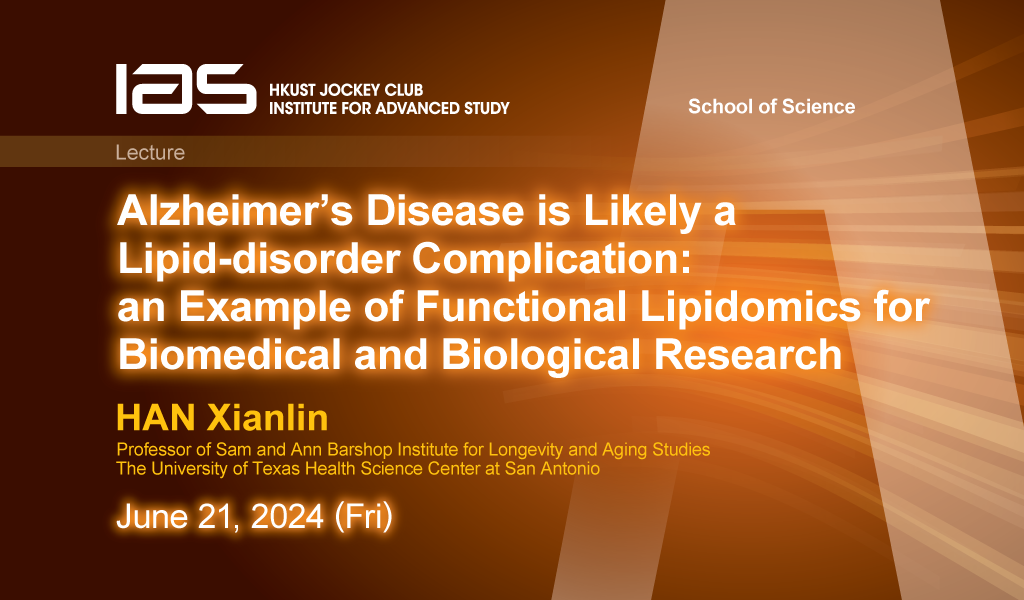