Recurrent neural networks like long short-term memory (LSTM) have been utilized as a tool for modeling and predicting dynamics of complex stochastic molecular systems. Previous studies have shown that Transformer has an advantage over LSTM in dealing with the memory loss of long-sequence data, and exceeds LSTM in many natural language processing tasks. In this seminar, we will show the implementation of Transformer on learning molecular dynamics and compare it with LSTM, which is greatly affected by lag time.
3 May 2021
3:00pm - 4:00pm
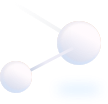
Where
https://hkust.zoom.com.cn/j/6218914432 (Passcode: hkust)
Speakers/Performers
Miss Wenqi ZENG
Organizer(S)
Department of Mathematics
Contact/Enquiries
Payment Details
Audience
Alumni, Faculty and staff, PG students, UG students
Language(s)
English
Other Events
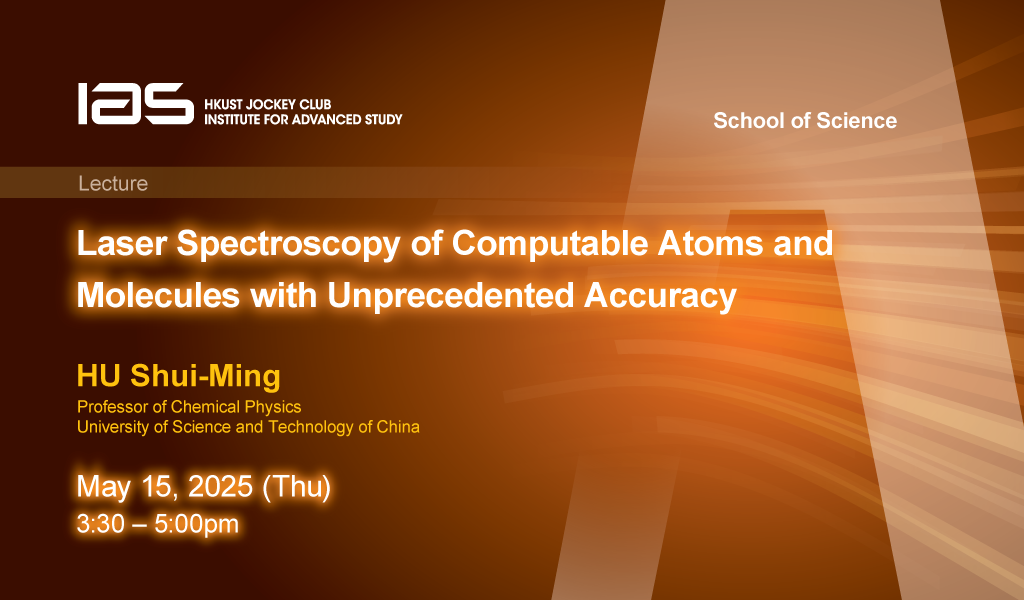
15 May 2025
Seminar, Lecture, Talk
IAS / School of Science Joint Lecture - Laser Spectroscopy of Computable Atoms and Molecules with Unprecedented Accuracy
Abstract
Precision spectroscopy of the hydrogen atom, a fundamental two-body system, has been instrumental in shaping quantum mechanics. Today, advances in theory and experiment allow us to ext...
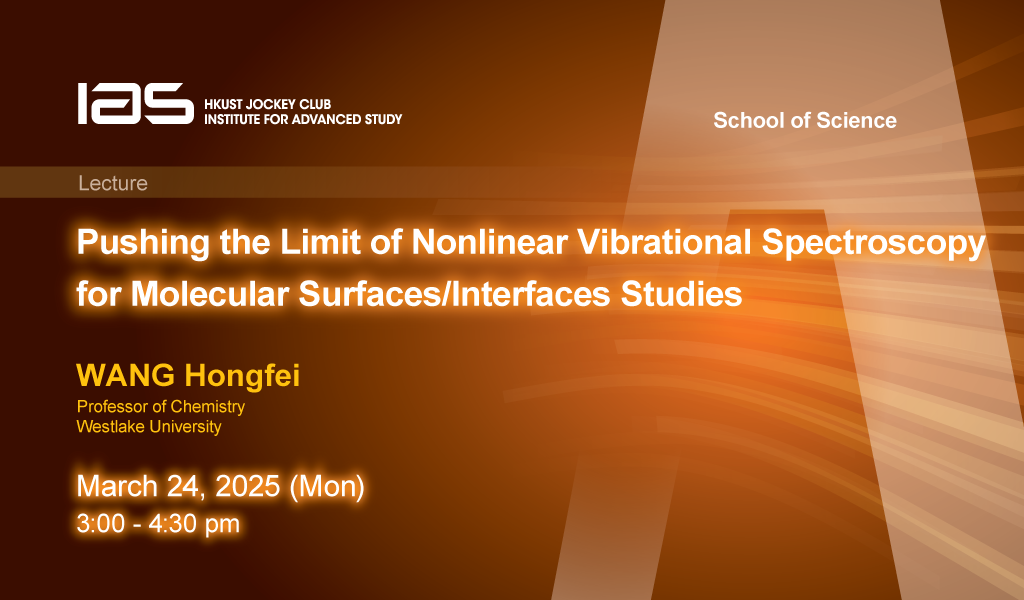
24 Mar 2025
Seminar, Lecture, Talk
IAS / School of Science Joint Lecture - Pushing the Limit of Nonlinear Vibrational Spectroscopy for Molecular Surfaces/Interfaces Studies
Abstract
Surfaces and interfaces are ubiquitous in Nature. Sum-frequency generation vibrational spectroscopy (SFG-VS) is a powerful surface/interface selective and sub-monolayer sensitive spect...