We consider the problem of minimizing an objective function without any derivative information. Such optimization is called zeroth-order, derivative-free, or black-box optimization. When the problem dimension is large-scale, the existing zeroth-order state-of-the-arts often suffer the curse of dimensionality. In this talk, we explore a novel compressible gradients assumption and propose two new methods, namely ZORO and SCOBO, for high-dimensional zeroth-order optimization. In particular, ZORO uses evaluations of the objective function and SCOBO uses only comparison information between points. Furthermore, we propose a block coordinate descent algorithm, coined ZO-BCD, for ultra-high-dimensional settings. We show the query complexities of ZORO, SCOBO, and ZO-BCD are only logarithmically dependent on the problem dimension. Numerical experiments show that the proposed methods outperform the state-of-the-arts on both synthetic and real datasets.
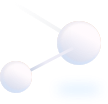
UCLA
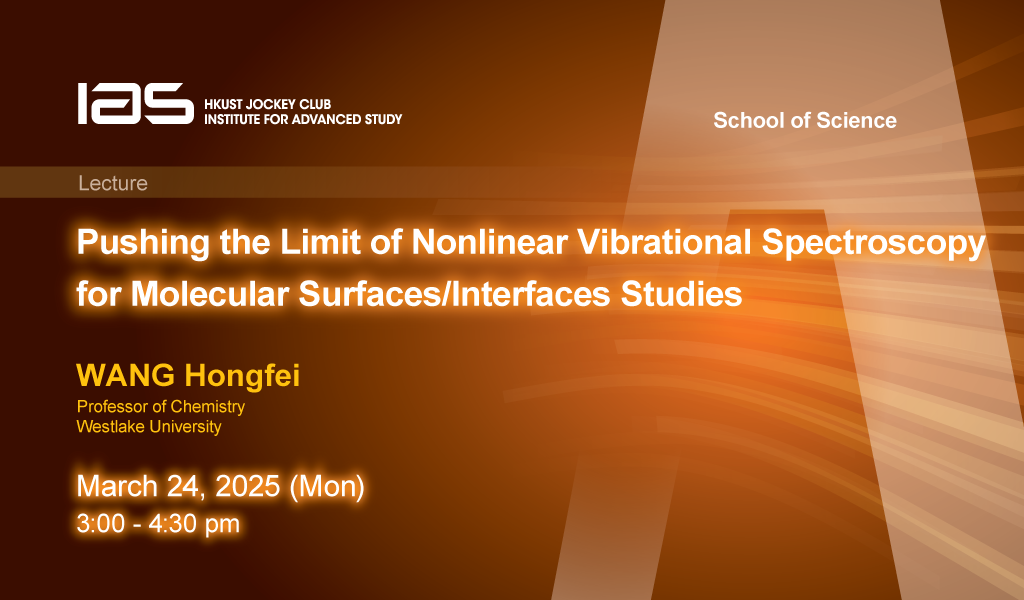
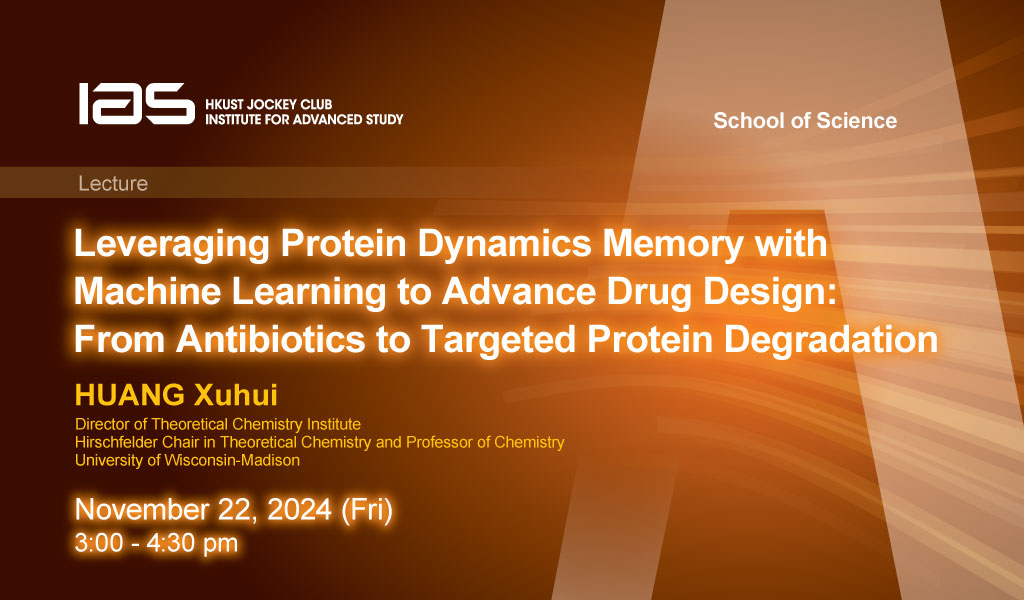