The genetic data has been extensively used to construct phenotype prediction, which was proved useful to stratify the general European population into different risk groups. However, genetic data-based predictions are less accurate in non-European populations due to genetic differences across different populations. To improve the prediction accuracy in non-European populations, we propose a cross-population analysis framework for genetic prediction with both individual-level (XPA) and summary-level (XPASS) GWAS data. By leveraging trans-ancestry genetic correlation, our methods can borrow information from the large-scale European population data to improve risk prediction in the non-European populations. With innovations in data structure and algorithm design, our methods provide a substantial saving in computational time and memory usage. Through comprehensive simulation studies, we show that our framework provides accurate, efficient, and robust prediction across a range of genetic architectures. In a Chinese cohort, our methods achieved 7.3%–198.0% accuracy gain for height and 19.5%–313.3% accuracy gain for body mass index (BMI) in terms of predictive R2 compared to existing prediction models.
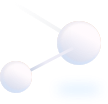
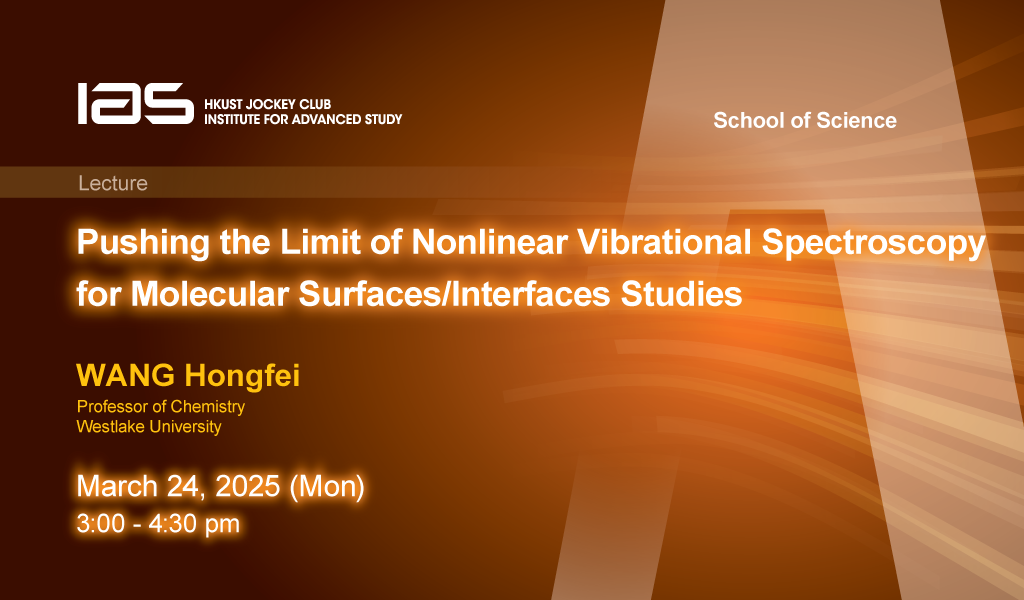
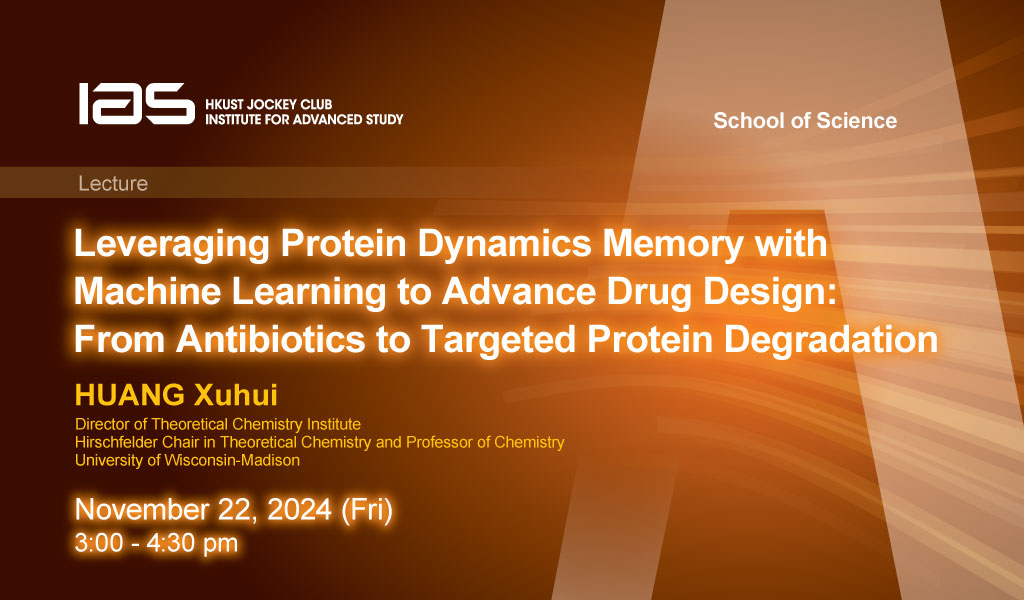