In this era of big data, quantifying relationships between different components of a complex system is an appealing and challenging problem. Gaussian graphical model incorporates an undirected graph whose edge describes the conditional dependence among variables, and it has a wide variety of applications in biological networks, social networks, and financial data. Precision matrix (also known as inverse covariance, or concentration matrix) encode the partial covariances between pairs of variables given others, whose nonzero entries correspond to edges in graphical model. We provide a new estimator of precision matrix in a pair-by-pair manner by regressing a pair of variables on the remaining ones each time. The minorize-maximization algorithm is applied to maximize log-likelihood function for parameter estimation. This procedure could be computationally efficient by parallel computing different pairs. More importantly, the core strength of this method is that the uncertainty of each edge can be quantified.
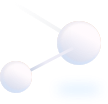
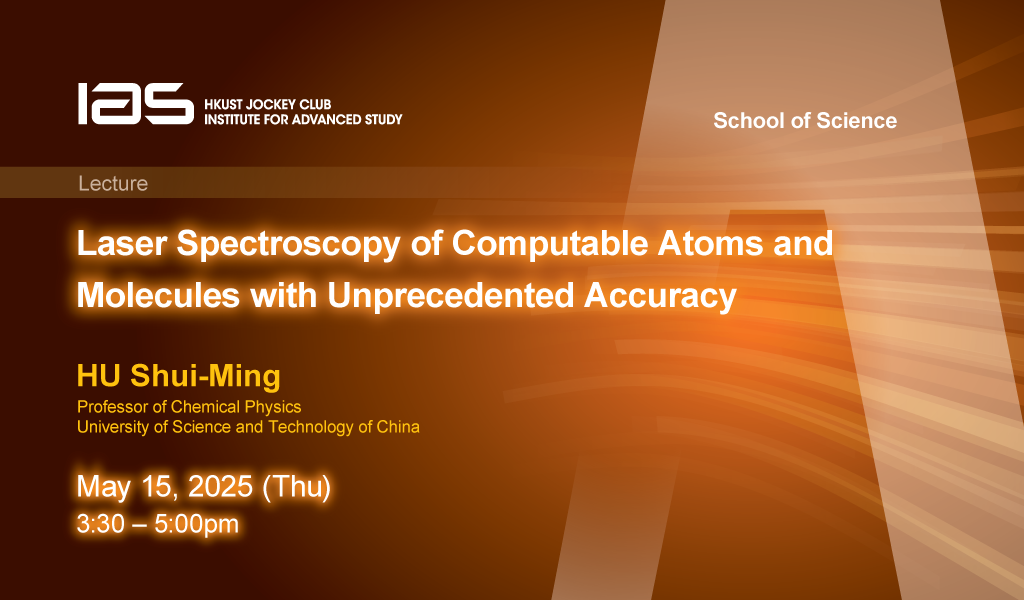