After its introduction in the seminal work of Koenker and Bassett (1978), quantile regression (QR) has gained widespread recognition and has found diverse applications in fields such as environmental sciences, economics, finance, genomics, and medicine. QR provides a valuable set of complementary methods that enable the exploration of data heterogeneity that may be overlooked by least squares methods. Despite the opportunities presented by big data analytics, traditional estimation and inference techniques for QR often demand significant computing power and memory resources that may not be readily available. In this talk, we will introduce CONQUER, an abbreviation for convolution-smoothed quantile regression, which transforms QR from a traditional statistical tool to a more efficient machine learning method capable of handling ultra-large datasets. We establish rigorous convergence guarantees for CONQUER under various settings. For penalized CONQUER with structured high-dimensional data, we propose a unified algorithm based on the local adaptive majorize-minimization principle, which applies to various convex penalties such as lasso, elastic-net, group lasso, and sparse group lasso, as well as folded-concave penalties after multi-step convex relaxations. Convolution smoothing strikes a balance between statistical optimality and computational efficiency, making QR adaptable to the Big Data era with massive datasets on parallel and distributed computing platforms. To facilitate practical usage, we have developed user-friendly CONQUER R and Python packages.
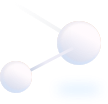
University of California San Diego
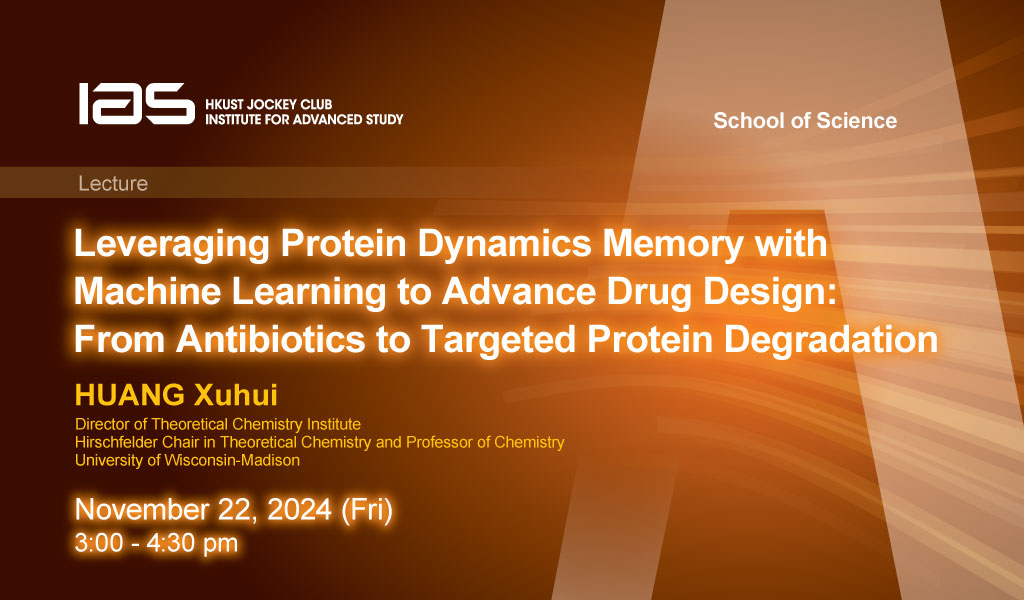
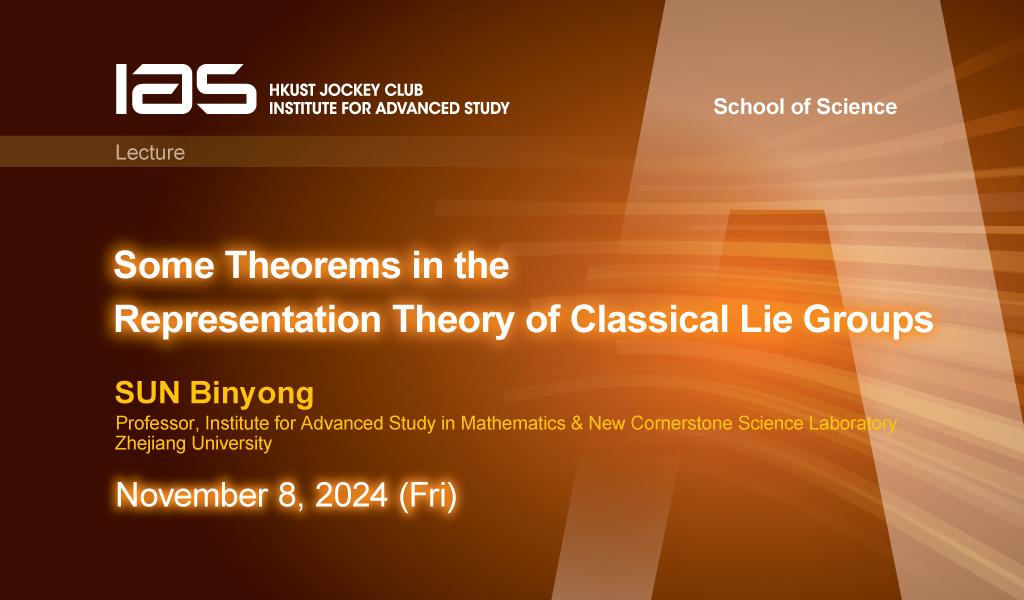