Conductivity imaging from various observational data represents one fundamental task in medical imaging. In this talk, we discuss numerical methods for identifying the conductivity parameters in elliptic PDEs. Commonly, a regularized formulation consists of a data fidelity and a regularizer is employed, and then it is discretized using finite difference method, finite element methods or deep neural networks in practical computation. One key issue is to establish a priori error estimates for the recovered conductivity distribution. In this talk, we discuss our recent findings on using deep neural networks for this class of problems, by effectively utilizing relevant stability results.
19 Jun 2023
4:00pm - 5:00pm
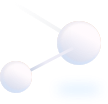
Where
Room 4475 (Lifts 25/26)
Speakers/Performers
Prof. Bangti JIN
The Chinese University of Hong Kong
The Chinese University of Hong Kong
Organizer(S)
Department of Mathematics
Contact/Enquiries
Payment Details
Audience
Alumni, Faculty and staff, PG students, UG students
Language(s)
English
Other Events
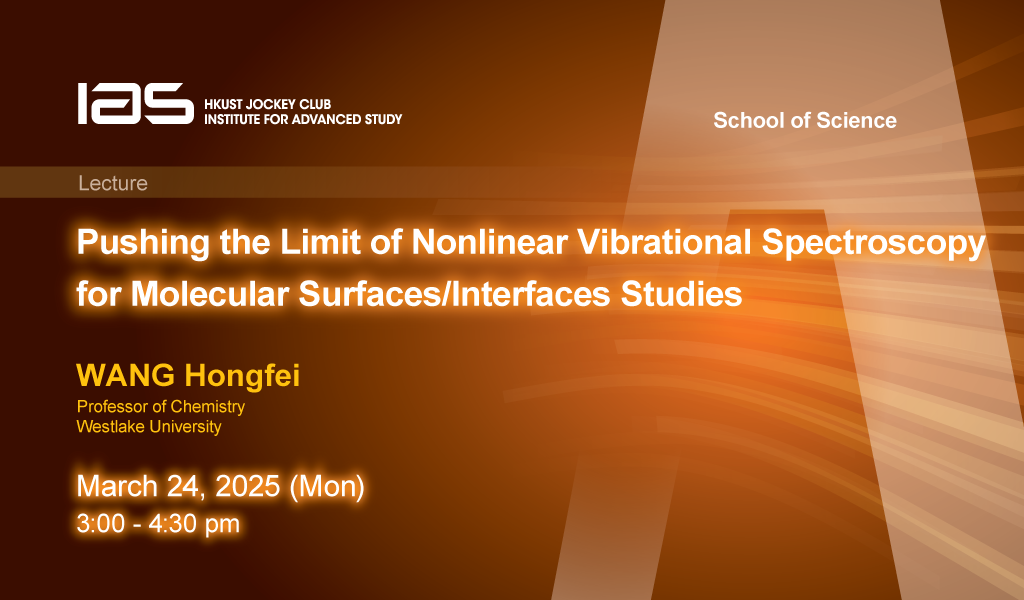
24 Mar 2025
Seminar, Lecture, Talk
IAS / School of Science Joint Lecture - Pushing the Limit of Nonlinear Vibrational Spectroscopy for Molecular Surfaces/Interfaces Studies
Abstract
Surfaces and interfaces are ubiquitous in Nature. Sum-frequency generation vibrational spectroscopy (SFG-VS) is a powerful surface/interface selective and sub-monolayer sensitive spect...
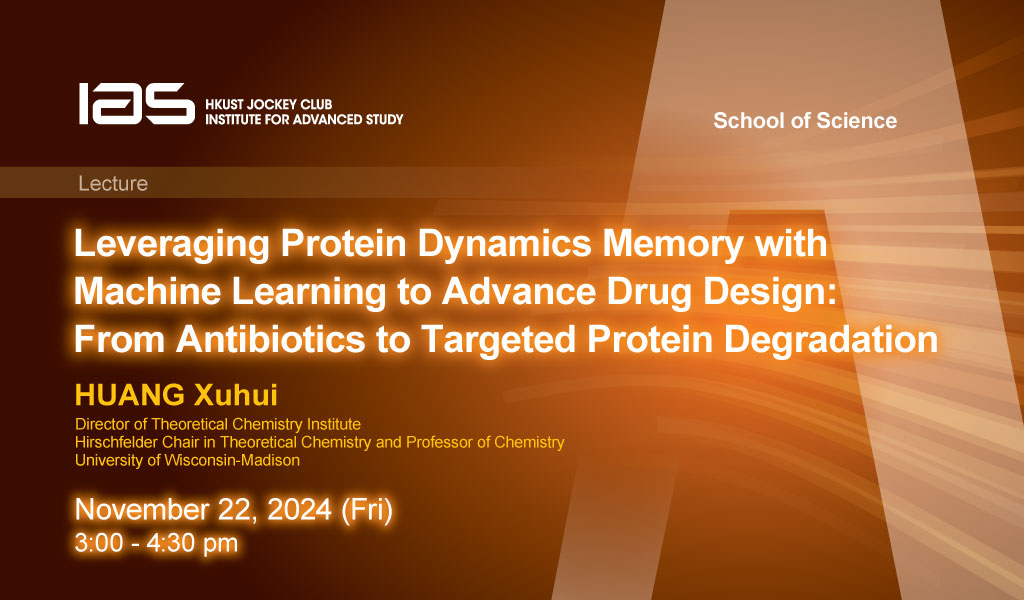
22 Nov 2024
Seminar, Lecture, Talk
IAS / School of Science Joint Lecture - Leveraging Protein Dynamics Memory with Machine Learning to Advance Drug Design: From Antibiotics to Targeted Protein Degradation
Abstract
Protein dynamics are fundamental to protein function and encode complex biomolecular mechanisms. Although Markov state models have made it possible to capture long-timescale protein co...