The invariant distribution, which is characterized by the stationary Fokker-Planck equation, is an important object in the study of randomly perturbed dynamical systems. Traditional numerical methods for computing the invariant distribution based on the Fokker-Planck equation, such as finite difference or finite element methods, are limited to low-dimensional systems due to the curse of dimensionality. In this work, we propose a deep learning based method to compute the generalized potential, i.e. the negative logarithm of the invariant distribution multiplied by the noise. The idea of the method is to learn a decomposition of the force field, as specified by the Fodder-Planck equation, from the trajectory data. The potential component of the decomposition gives the generalized potential. The method can deal with high-dimensional systems, possibly with partially known dynamics. Using the generalized potential also allows us to deal with systems at low temperatures, where the invariant distribution becomes singular around the metastable states. These advantages make it an efficient method to analyze invariant distributions for practical dynamical systems. The effectiveness of the proposed method is demonstrated by numerical examples.
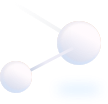
National University of Singapore
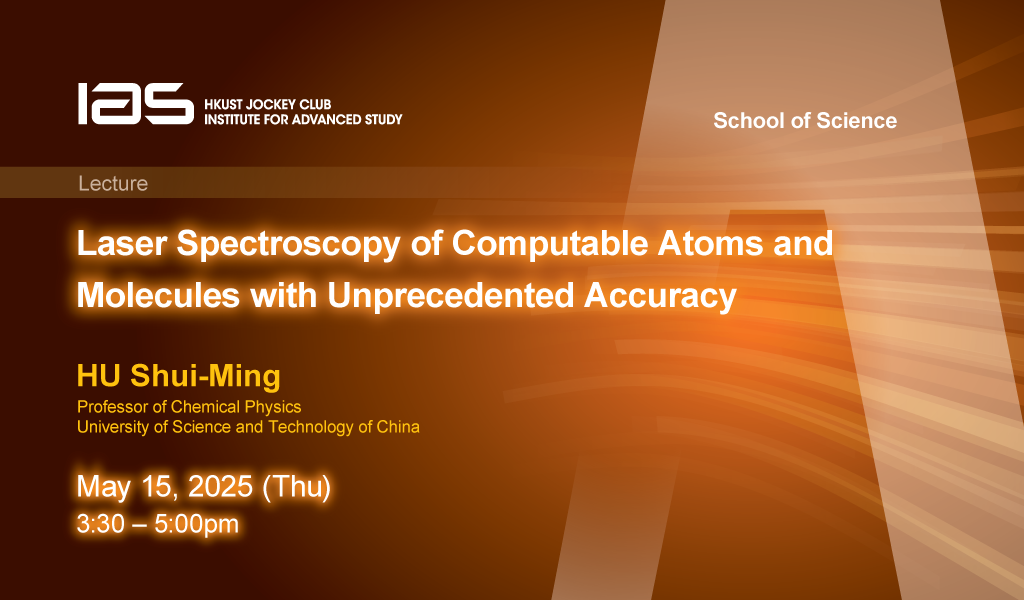
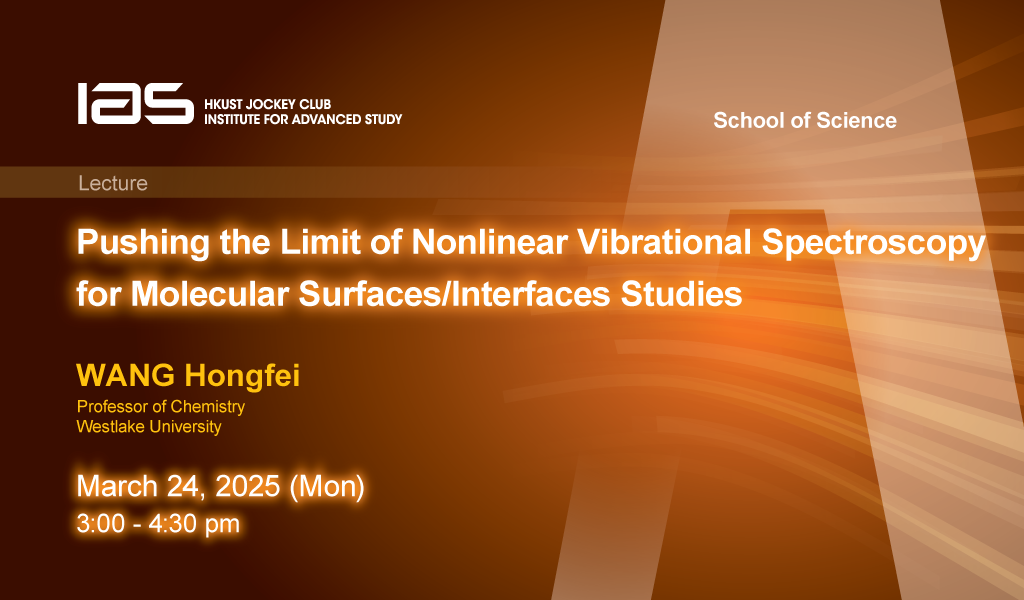