A recent line of research on deep learning shows that the training of extremely wide neural networks can be characterized by a kernel function called neural tangent kernel (NTK). However, it is known that this type of result does not perfectly match the practice, as NTK-based analysis requires the network weights to stay very close to their initialization throughout training, and cannot handle regularizers or gradient noises. In this talk, I will present a generalized neural tangent kernel analysis and show that noisy gradient descent with weight decay can still exhibit a ``kernel-like'' behavior. This implies that the training loss converges linearly up to a certain accuracy. I will also discuss the generalization error of an infinitely wide two-layer neural network trained by noisy gradient descent with weight decay.
14 Aug 2020
11:00am - 12:00pm
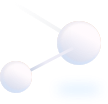
Where
https://hkust.zoom.us/j/5616960008
Speakers/Performers
Dr. Yuan CAO
UCLA
UCLA
Organizer(S)
Department of Mathematics
Contact/Enquiries
mathseminar@ust.hk
Payment Details
Audience
Alumni, Faculty and Staff, PG Students, UG Students
Language(s)
English
Other Events
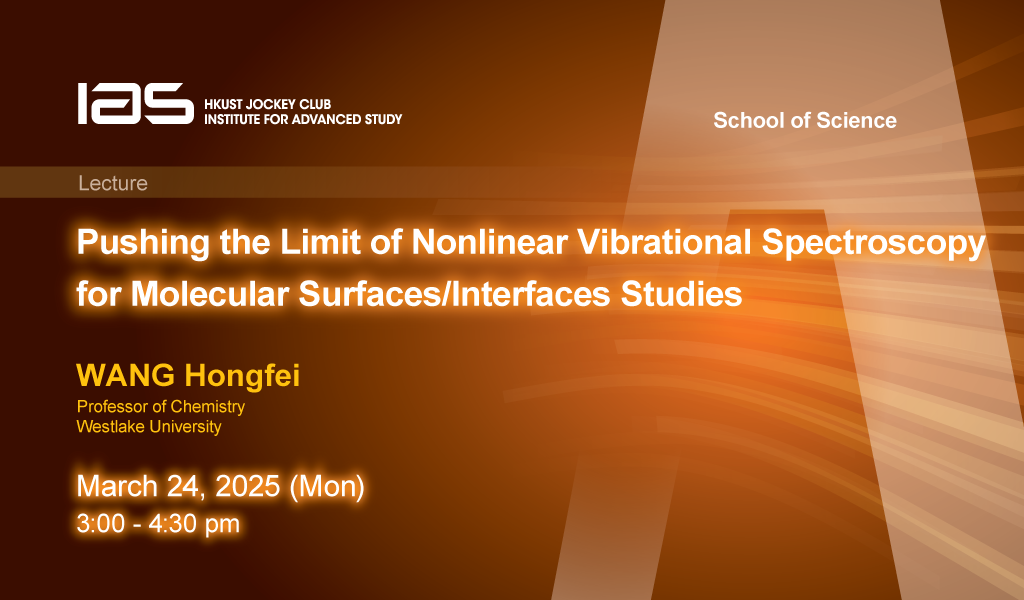
24 Mar 2025
Seminar, Lecture, Talk
IAS / School of Science Joint Lecture - Pushing the Limit of Nonlinear Vibrational Spectroscopy for Molecular Surfaces/Interfaces Studies
Abstract
Surfaces and interfaces are ubiquitous in Nature. Sum-frequency generation vibrational spectroscopy (SFG-VS) is a powerful surface/interface selective and sub-monolayer sensitive spect...
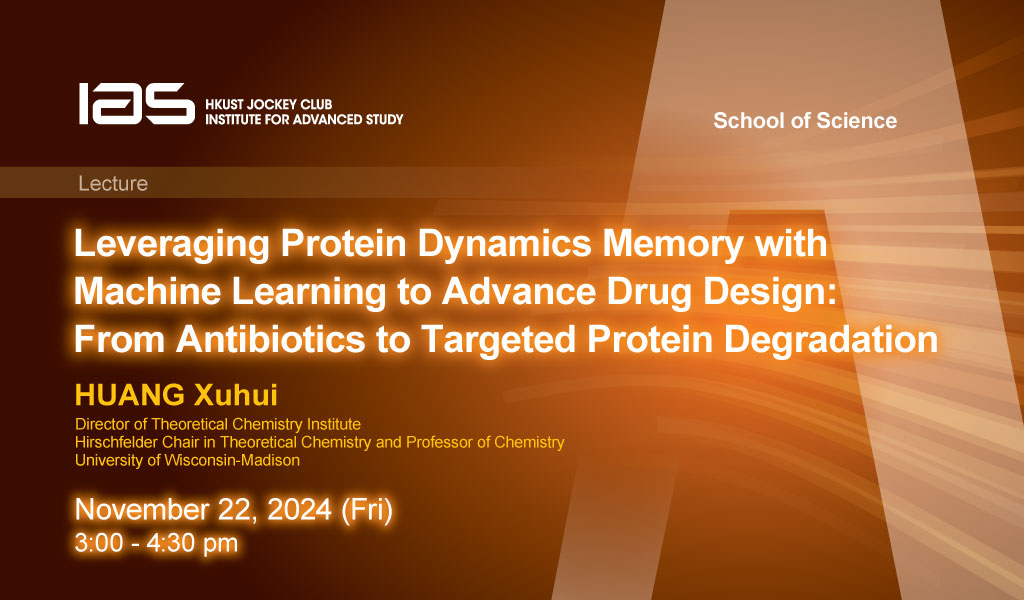
22 Nov 2024
Seminar, Lecture, Talk
IAS / School of Science Joint Lecture - Leveraging Protein Dynamics Memory with Machine Learning to Advance Drug Design: From Antibiotics to Targeted Protein Degradation
Abstract
Protein dynamics are fundamental to protein function and encode complex biomolecular mechanisms. Although Markov state models have made it possible to capture long-timescale protein co...