Recently, there has been a great deal of research attention on understanding the convergence behavior of first-order methods using tools from continuous dynamical systems. The alternating direction method of multipliers (ADMM) is a widely used first-order method for solving optimization problems arising from machine learning and statistics, and the stochastic versions of ADMM plays a key role in many modern large-scale machine learning problems. We introduce a unified algorithmic framework called generalized stochastic ADMM and investigate it via a continuous-time analylsis. We rigorously proved that under some proper scaling, the trajectory of stochastic ADMM weakly converges to the trajectory of the stochastic differential equation with small noise parameters. Our analysis also provides a theoretical explanation on why the relaxation parameter should be chosen between 0 and 2.
30 Jun 2020
11:00am - 12:00pm
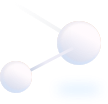
Where
https://hkust.zoom.us/j/5616960008
Speakers/Performers
Dr. Huizhuo YUAN
Peking University
Peking University
Organizer(S)
Department of Mathematics
Contact/Enquiries
mathseminar@ust.hk
Payment Details
Audience
Alumni, Faculty and Staff, PG Students, UG Students
Language(s)
English
Other Events
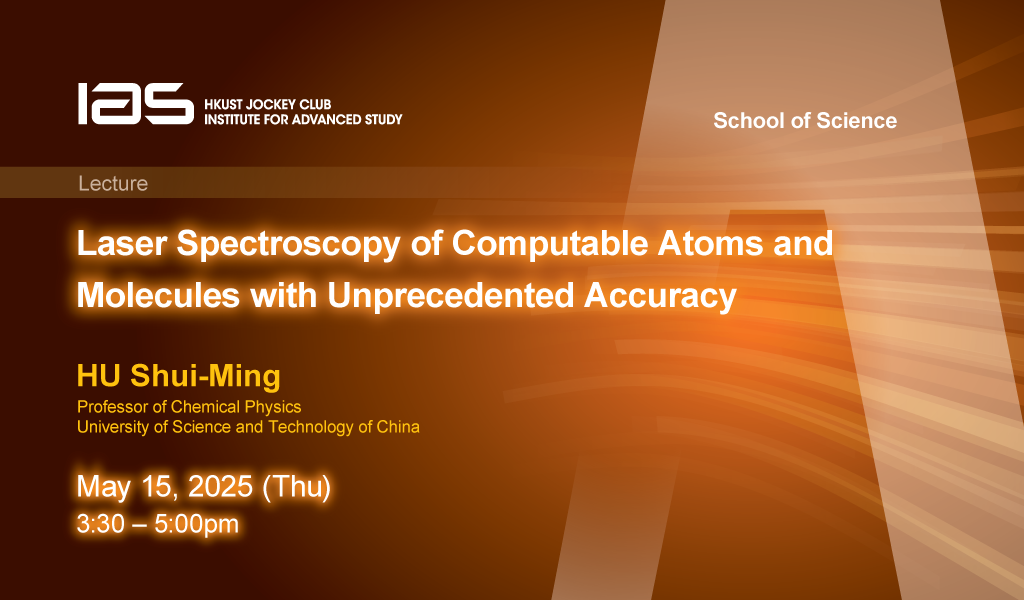
15 May 2025
Seminar, Lecture, Talk
IAS / School of Science Joint Lecture - Laser Spectroscopy of Computable Atoms and Molecules with Unprecedented Accuracy
Abstract
Precision spectroscopy of the hydrogen atom, a fundamental two-body system, has been instrumental in shaping quantum mechanics. Today, advances in theory and experiment allow us to ext...