The analysis of geometric (graph- and manifold-structured) data have recently gained prominence in the machine learning community. For the first part of the talk, I will introduce Lanczos network (LanczosNet), which uses the Lanczos algorithm to construct low rank approximations of the graph Laplacian for graph convolution. Relying on the tridiagonal decomposition of the Lanczos algorithm, we efficiently exploit multi-scale information via fast approximated computation of matrix power, and design learnable spectral filters. Being fully differentiable, LanczosNet facilitates both graph kernel learning as well as learning node embeddings. I will show the application of LanczosNet to citation networks and QM8 quantum chemistry dataset.
For the second part of the talk, I will introduce a novel multi-representation learning paradigm for manifolds naturally equipped with a group action. Utilizing a representation theoretic mechanism, multiple associated vector bundles can be constructed over the orbit space, providing multiple views for learning the geometry of the underlying manifold. The consistency across these associated vector bundles form a common base for unsupervised manifold learning, through the redundancy inherent to the algebraic relations across irreducible representations of the transformation group. I will demonstrate the efficacy of the proposed algorithmic paradigm through dramatically improved robust nearest neighbor search in cryo-electron microscopy image analysis.
For the second part of the talk, I will introduce a novel multi-representation learning paradigm for manifolds naturally equipped with a group action. Utilizing a representation theoretic mechanism, multiple associated vector bundles can be constructed over the orbit space, providing multiple views for learning the geometry of the underlying manifold. The consistency across these associated vector bundles form a common base for unsupervised manifold learning, through the redundancy inherent to the algebraic relations across irreducible representations of the transformation group. I will demonstrate the efficacy of the proposed algorithmic paradigm through dramatically improved robust nearest neighbor search in cryo-electron microscopy image analysis.
26 Sep 2019
4:30pm - 5:50pm
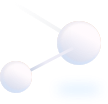
Where
Room 2405, Academic Building (near Lifts 17-18), HKUST
Speakers/Performers
Prof. Zhizhen ZHAO
Department of Electrical and Computer Engineering, University of Illinois at Urbana-Champaign
Department of Electrical and Computer Engineering, University of Illinois at Urbana-Champaign
Organizer(S)
Department of Mathematics
Contact/Enquiries
mathseminar@ust.hk
Payment Details
Audience
Alumni, Faculty and Staff, PG Students, UG Students
Language(s)
English
Other Events
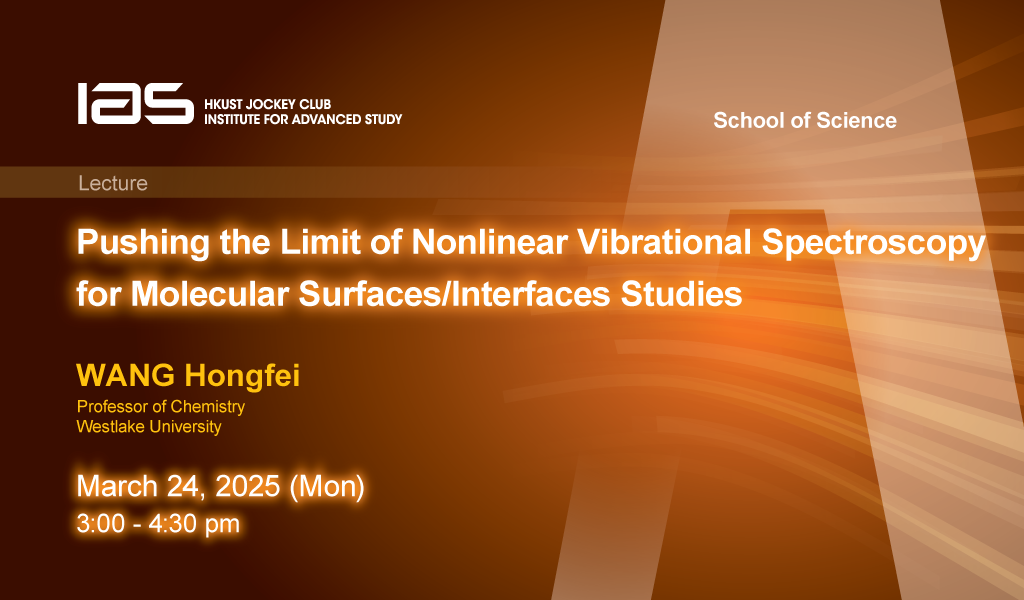
24 Mar 2025
Seminar, Lecture, Talk
IAS / School of Science Joint Lecture - Pushing the Limit of Nonlinear Vibrational Spectroscopy for Molecular Surfaces/Interfaces Studies
Abstract
Surfaces and interfaces are ubiquitous in Nature. Sum-frequency generation vibrational spectroscopy (SFG-VS) is a powerful surface/interface selective and sub-monolayer sensitive spect...
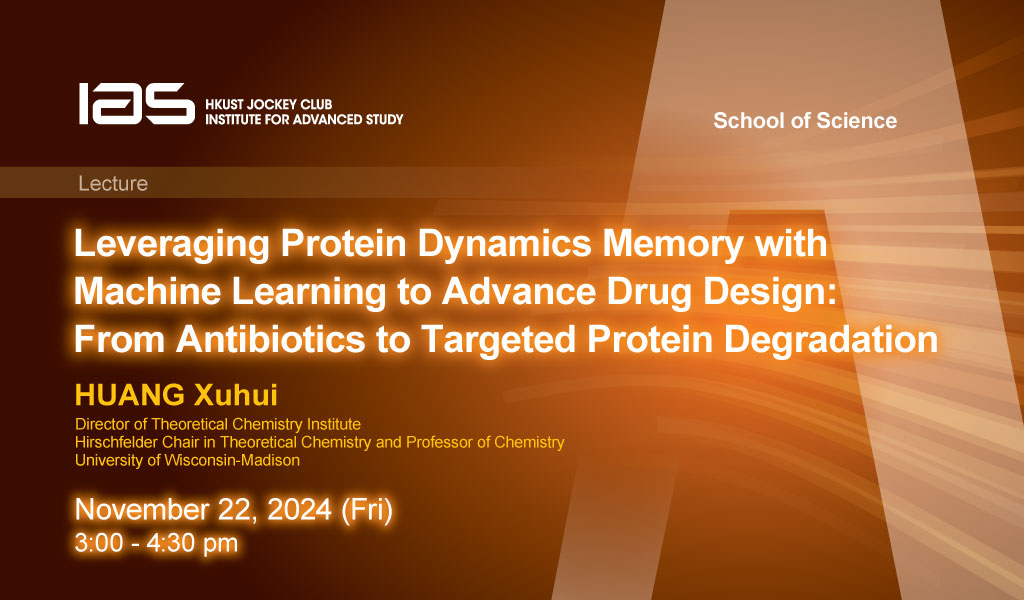
22 Nov 2024
Seminar, Lecture, Talk
IAS / School of Science Joint Lecture - Leveraging Protein Dynamics Memory with Machine Learning to Advance Drug Design: From Antibiotics to Targeted Protein Degradation
Abstract
Protein dynamics are fundamental to protein function and encode complex biomolecular mechanisms. Although Markov state models have made it possible to capture long-timescale protein co...