Department of Mathematics
Development of Statistical and Machine Learning Methods for Large Scale Genomic Data Analysis
This is an ITF project with the industry partner WeGene. Through the WeGene platform, genotype data has been collected from tens of thousands of samples as well as their phenotypic information such as human height and 2D facial images. In this study, it aims to develop statistical and machine learning methods for human genomic big data analysis, such as identification of risk variants for different phenotypes in the East Asia population, prediction of human adult height and reconstruction of human 2D facial shapes from genomic data.
The integration of the WeGene IT architecture and the newly developed machine learning methods, such as dimension-reduction, regression model and a deep neural network, enables high-performance computational environments to handle genomic big data and reveal the link between human genomic data and human complex phenotypes. The application of the techniques developed in this project not only facilitate health management (risk prediction for complex traits and disease), but also provide new tools to connect genomic data with imaging data.
Related research publications:
- X. Luo, C. Yang, Y. Wei. Detection of cell-type-specific risk-CpG sites in epigenome-wide association studies. Nature Communications, 10, 3113. 2019.
- Z. Yuan, H. Zhu, P. Zeng, S. Yang, S. Sun, C. Yang, J. Liu, Xiang Zhou. Testing and controlling for horizontal pleiotropy with the probabilistic Mendelian randomization in transcriptome-wide association studies. 11, 3861. 2020.
Software:
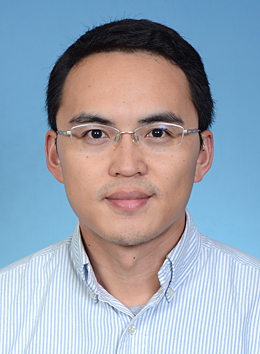
- Professor, Department of Mathematics
- Associate Director of Big Data for Bio Intelligence Laboratory
Scientific Breakthroughs & Discoveries
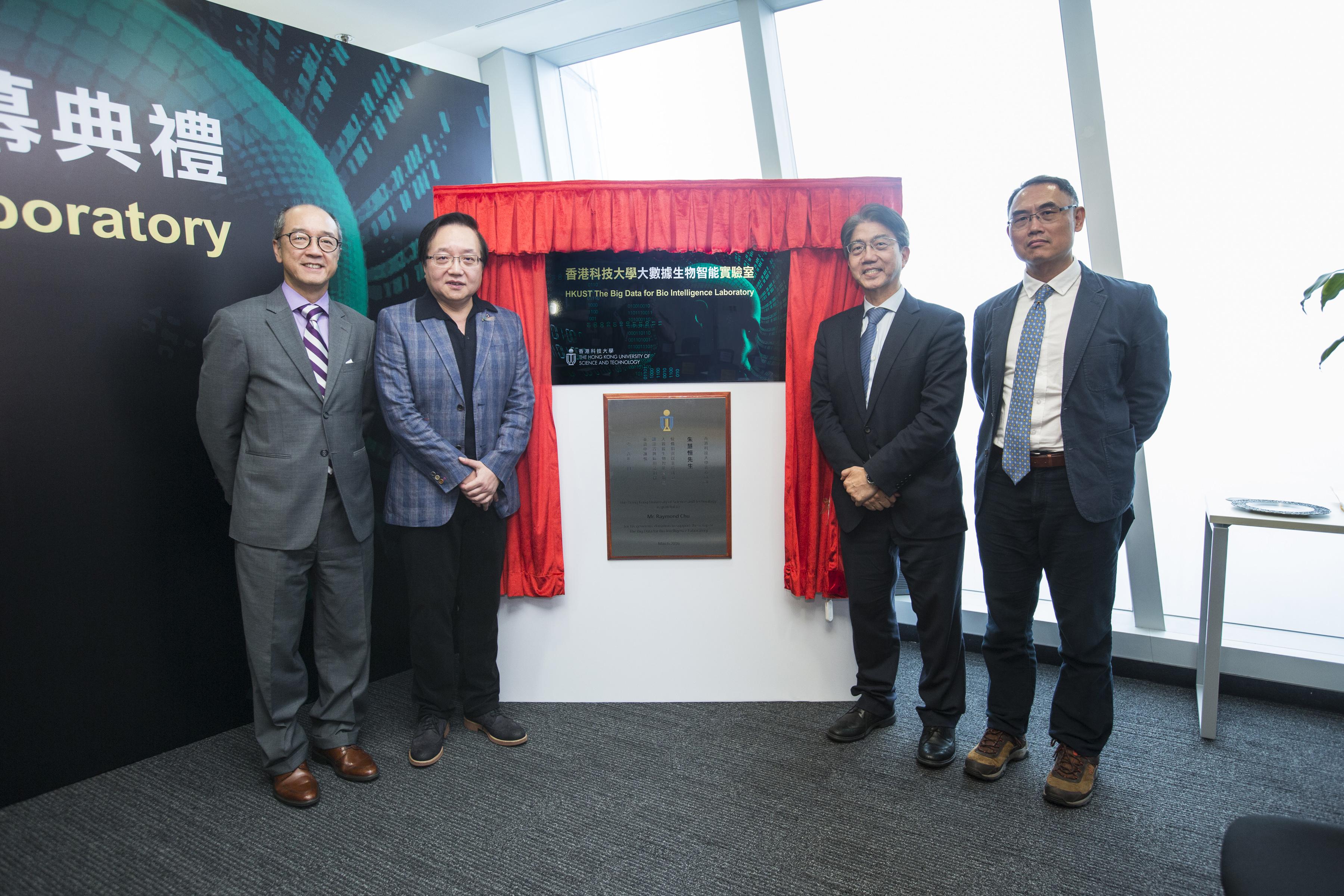
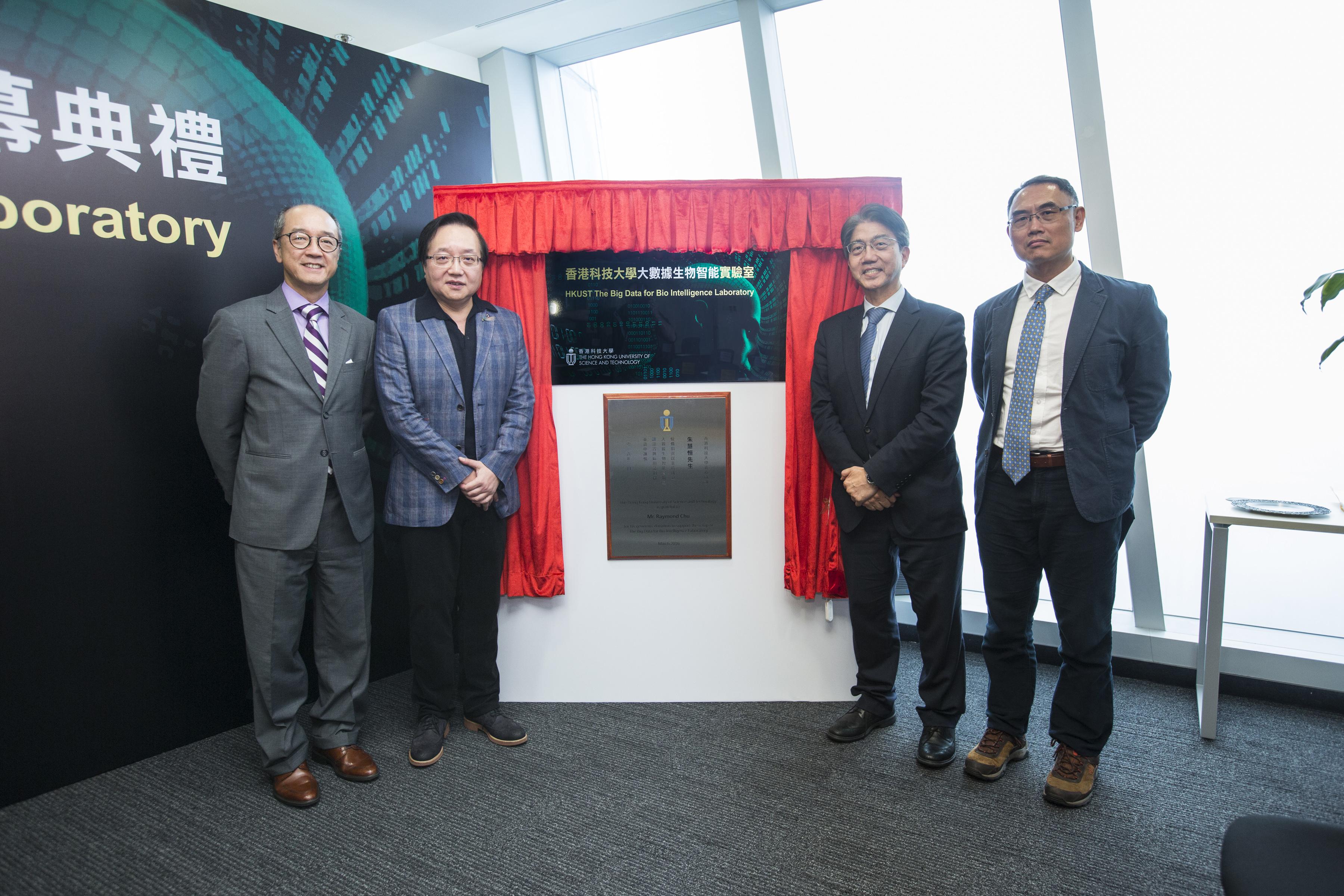
|
HKUST Establishes Laboratory on Big Data for Bio…
HKUST celebrated the opening of The Big Data for Bio Intelligence Laboratory which is dedicated to designing data analytic solutions for big data in biology...