Department of Mathematics
Geometric Landscape Analysis of Some Non-Convex Optimizations
Non-convex optimization is one of the most powerful tools for solving scientific and engineering problems. Many important problems, from traditional low-rank approximation in numerical linear algebra to modern deep neural network training in machine learning, are formulated as non-convex optimization problems. For many of such problems, simple algorithms, such as alternating minimization and gradient descent, often work remarkably well, despite possible local minima caused by non-convexity. The success of such simple algorithms remains a mystery for many problems with practical applications.
One way to examine why these simple algorithms perform successfully for non-convex optimization is to study the landscape of the objective functions by characterizing and locating their critical points. Recently, there has been a stream of research dedicated to the landscape analysis of non-convex functions arising in high-dimensional data analysis with low-dimensional models, including blind deconvolution, dictionary learning, tensor completion, phase synchronization, deep neural networks, low-rank matrix recovery, and phase retrieval. Those results revealed that there are no poor local minima of those non-convex functions. Consequently, any algorithm finding a local minimum will give a good solution. Therefore, the interested problems can be efficiently and effectively solved with a theoretical guarantee by a large class of algorithms.
In our research project, we investigated landscape of non-convex optimization arising from phase retrieval, which is a fundamental problem in many imaging techniques such as X-ray crystallography, transmission electron microscopy, and coherent diffractive imaging. We want to obtain a high-resolution image by using a limited number of samples. We have shown that, with a nearly optimal sample size, a family of non-convex optimizations for phase retrieval can have a benign landscape --- all local minima are global and all other critical points have negative directional curvatures. Combined with algorithms that are guaranteed to find a local minimum, phase retrieval problems can be efficiently solved with a theoretical guarantee using many optimization algorithms. Our results not only provide a comprehensive understanding on why simple non-convex algorithms usually find a global minimizer for phase retrieval, but also will be useful for developing new efficient algorithms with a theoretical guarantee by applying algorithms that are guaranteed to find a local minimum.
Scientific Breakthroughs & Discoveries
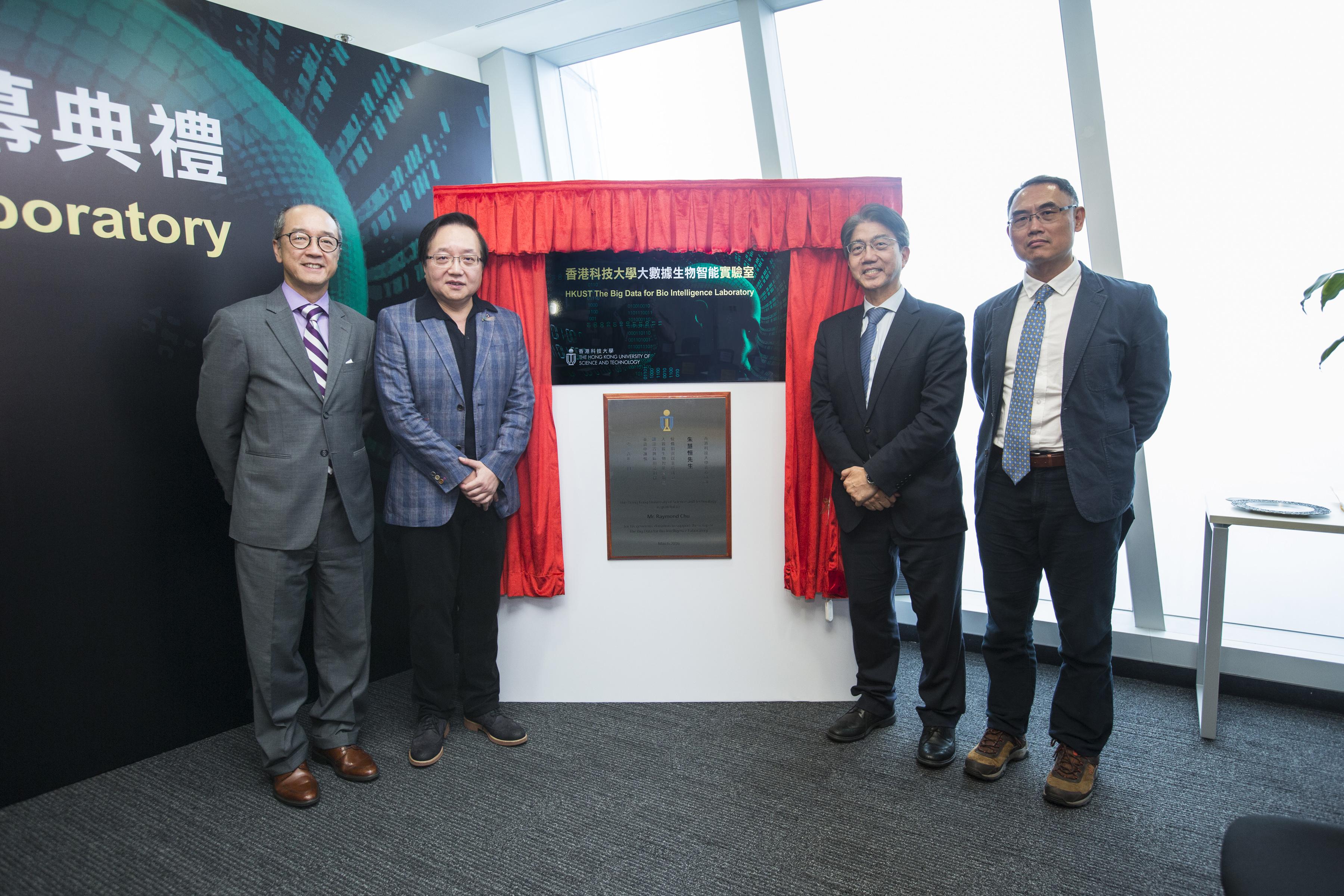
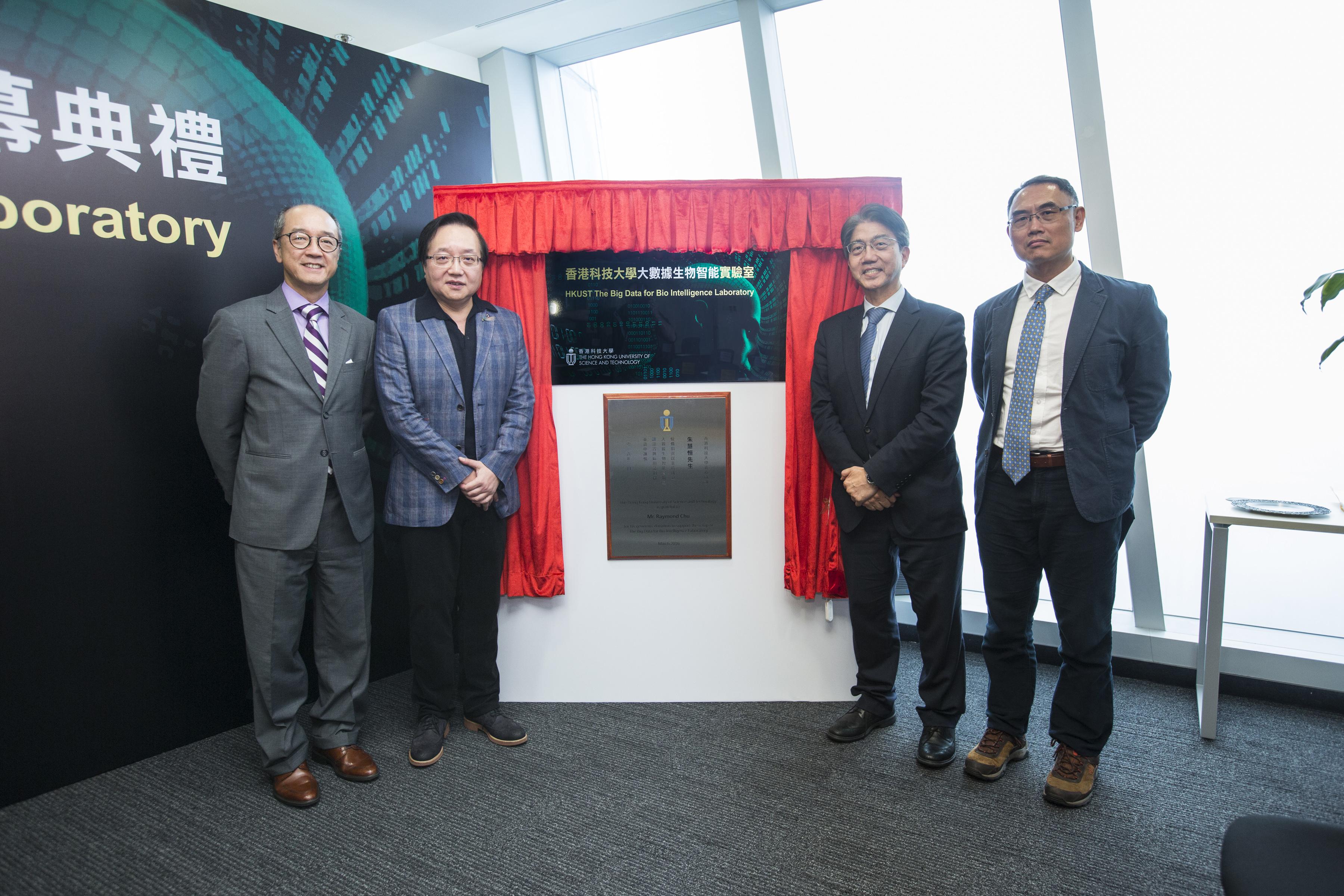
|
HKUST Establishes Laboratory on Big Data for Bio…
HKUST celebrated the opening of The Big Data for Bio Intelligence Laboratory which is dedicated to designing data analytic solutions for big data in biology...